Loss Surface Simplexes For Mode Connecting Volumes And Fast Ensembling
INTERNATIONAL CONFERENCE ON MACHINE LEARNING, VOL 139(2021)
摘要
With a better understanding of the loss surfaces for multilayer networks, we can build more robust and accurate training procedures. Recently it was discovered that independently trained SGD solutions can be connected along one-dimensional paths of near-constant training loss. In this paper, we show that there are in fact mode-connecting simplicial complexes that form multi-dimensional manifolds of low loss, connecting many independently trained models. Inspired by this discovery, we show how to efficiently build simplicial complexes for fast ensembling, outperforming independently trained deep ensembles in accuracy, calibration, and robustness to dataset shift. Notably, our approach only requires a few training epochs to discover a low-loss simplex, starting from a pre-trained solution. Code is available at https ://github.com/g-benton/loss-surface-simplexes.
更多查看译文
关键词
Dynamic Mode Decomposition
AI 理解论文
溯源树
样例
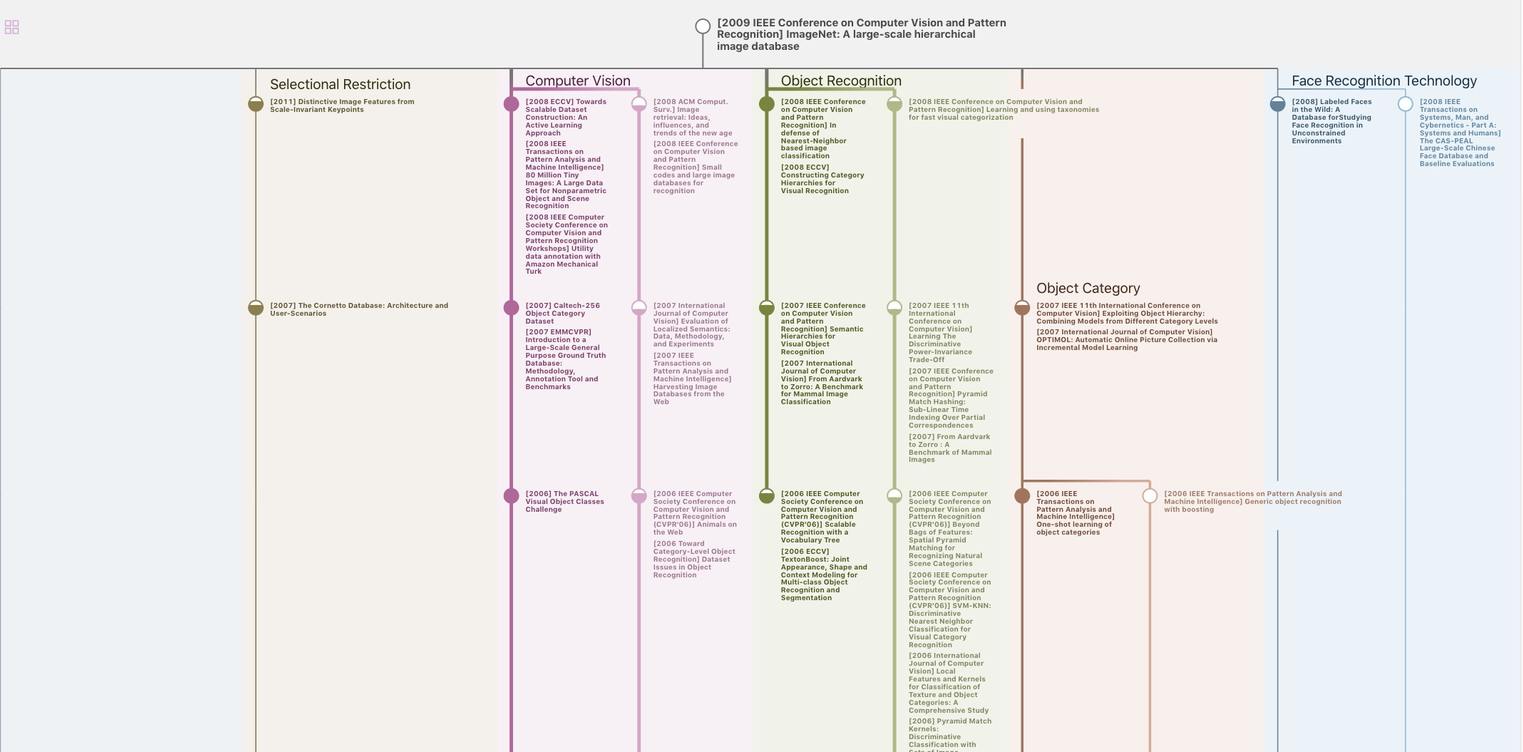
生成溯源树,研究论文发展脉络
Chat Paper
正在生成论文摘要