Developing a Segmentation Model for Microscopic Images of Microplastics Isolated from Clams.
ICPR Workshops(2020)
摘要
Microplastics (MP) have become a major concern, given the threat they pose to marine-derived food and human health. One way to investigate this threat is to quantify MP found in marine organisms, for instance making use of image analysis to identify ingested MP in fluorescent microscopic images. In this study, we propose a deep learning-based segmentation model to generate binarized images (masks) that make it possible to clearly separate MP from other background elements in the aforementioned type of images. Specifically, we created three variants of the U-Net model with a ResNet-101 encoder, training these variants with 99 high-resolution fluorescent images containing MP, each having a mask that was generated by experts using manual color threshold adjustments in ImageJ. To that end, we leveraged a sliding window and random selection to extract patches from the high-resolution images, making it possible to adhere to input constraints and to increase the number of labeled examples. When measuring effectiveness in terms of accuracy, recall, and F 2 -score, all segmentation models exhibited low scores. However, compared to two ImageJ baseline methods, the effectiveness of our segmentation models was better in terms of precision, F 0.5 -score, F 1 -score, and mIoU: U-Net (1) obtained the highest mIoU of 0.559, U-Net (2) achieved the highest F 1 -score of 0.682, and U-Net (3) had the highest precision and F 0.5 -score of 0.594 and 0.626, respectively, with our segmentation models, in general, detecting less false positives in the predicted masks. In addition, U-Net (1), which used binary cross-entropy loss and stochastic gradient descent, and U-Net (2), which used dice loss and Adam, were most effective in discriminating MP from other background elements. Overall, our experimental results suggest that U-Net (1) and U-Net (2) allow for more effective MP identification and measurement than the macros currently available in ImageJ.
更多查看译文
关键词
Deep learning, Environmental monitoring, Image segmentation, Microplastics
AI 理解论文
溯源树
样例
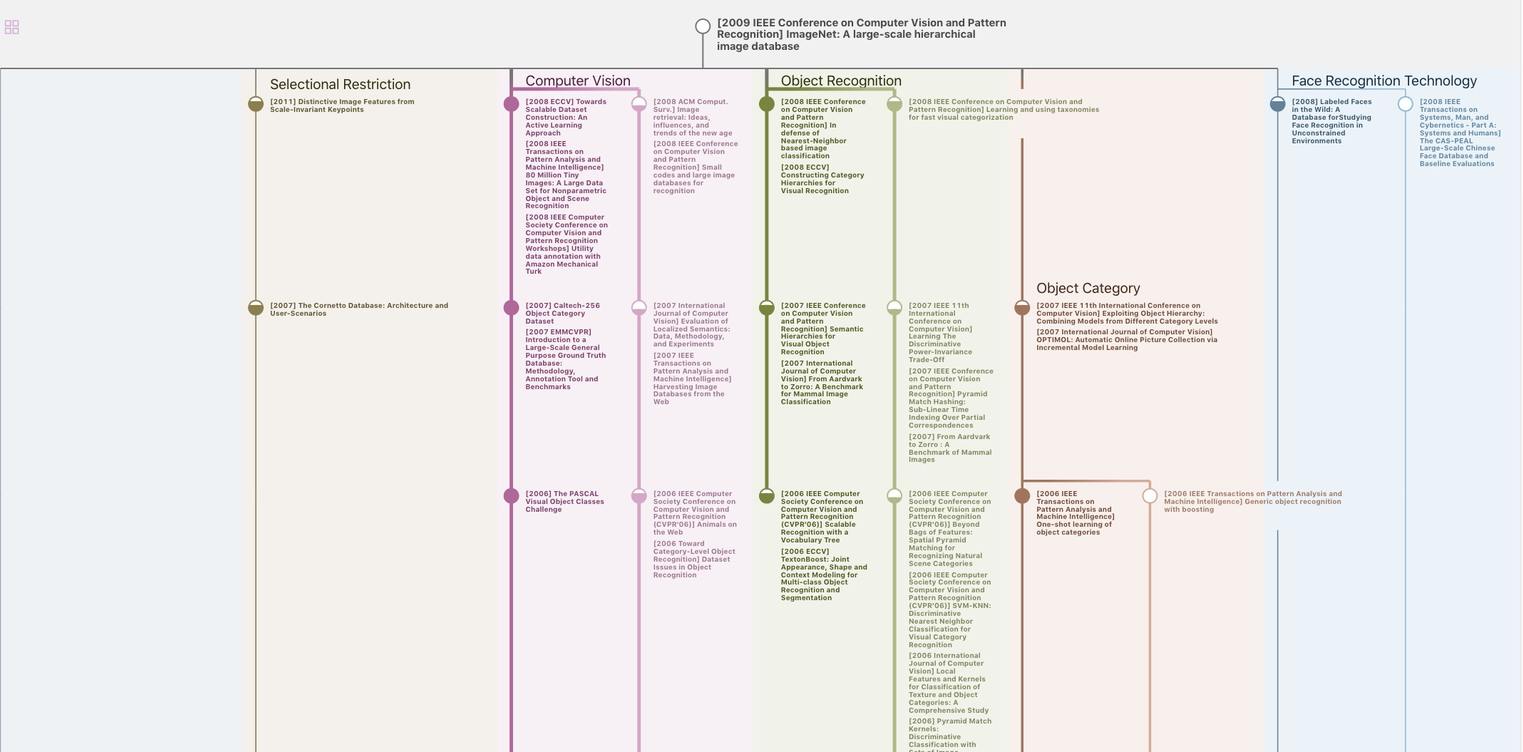
生成溯源树,研究论文发展脉络
Chat Paper
正在生成论文摘要