Multiview Subspace Clustering by an Enhanced Tensor Nuclear Norm
IEEE Transactions on Cybernetics(2022)
摘要
Despite the promising preliminary results, tensor-singular value decomposition (t-SVD)-based multiview subspace is incapable of dealing with real problems, such as noise and illumination changes. The major reason is that tensor-nuclear norm minimization (TNNM) used in t-SVD regularizes each singular value equally, which does not make sense in matrix completion and coefficient matrix learning. In this case, the singular values represent different perspectives and should be treated differently. To well exploit the significant difference between singular values, we study the weighted tensor Schatten
$p$
-norm based on t-SVD and develop an efficient algorithm to solve the weighted tensor Schatten
$p$
-norm minimization (WTSNM) problem. After that, applying WTSNM to learn the coefficient matrix in multiview subspace clustering, we present a novel multiview clustering method by integrating coefficient matrix learning and spectral clustering into a unified framework. The learned coefficient matrix well exploits both the cluster structure and high-order information embedded in multiview views. The extensive experiments indicate the efficiency of our method in six metrics.
更多查看译文
关键词
Multiview clustering,spectral clustering,tensor-singular value decomposition (t-SVD),weighted nuclear norm
AI 理解论文
溯源树
样例
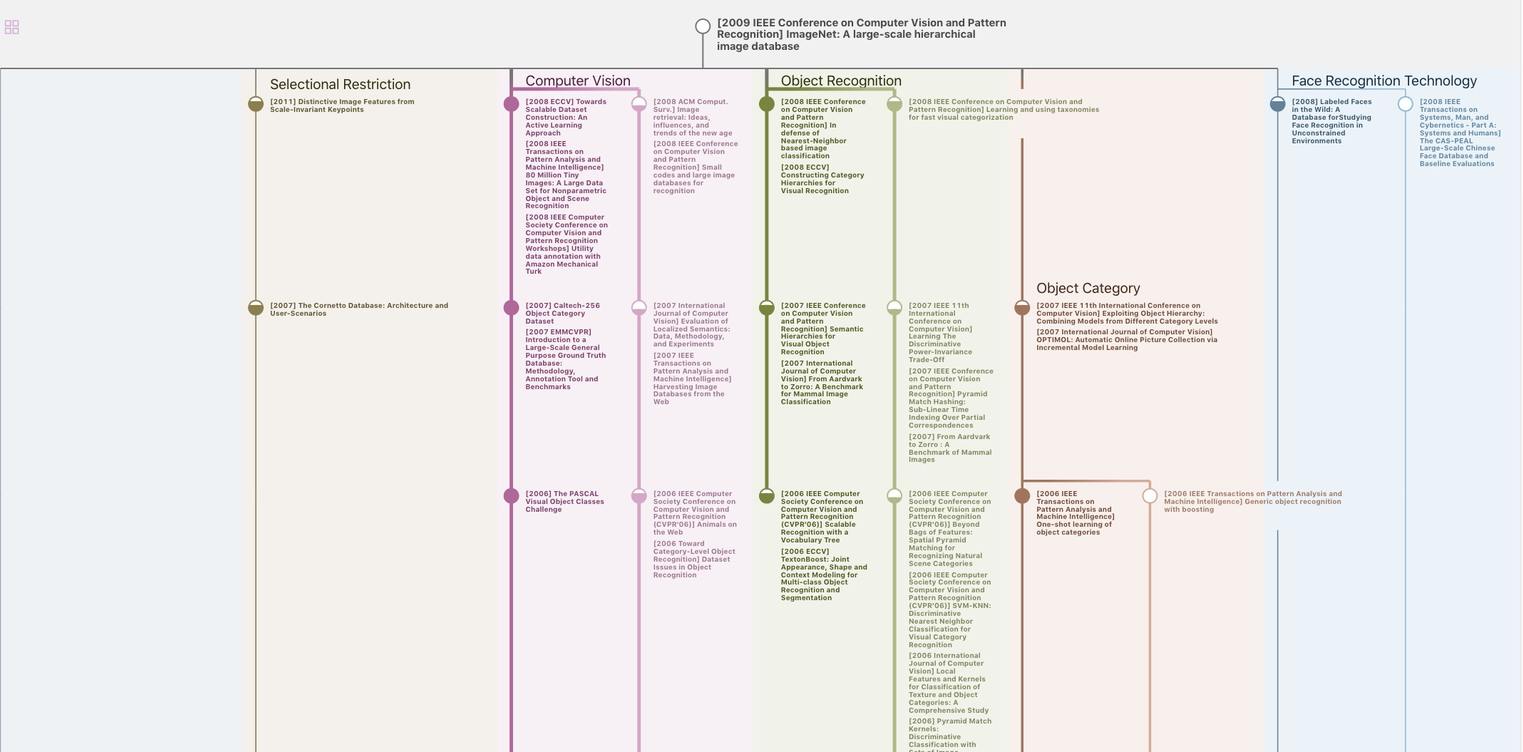
生成溯源树,研究论文发展脉络
Chat Paper
正在生成论文摘要