A computational biomarker of juvenile myoclonic epilepsy from resting-state MEG
Clinical Neurophysiology(2021)
摘要
Objective
For people with idiopathic generalized epilepsy, functional networks derived from their resting-state scalp electrophysiological recordings have shown an inherent higher propensity to generate seizures than those from healthy controls when assessed using the concept of brain network ictogenicity (BNI). Herein we tested whether the BNI framework is applicable to resting-state magnetoencephalography (MEG) from people with juvenile myoclonic epilepsy (JME).
Methods
The BNI framework consists in deriving a functional network from apparently normal brain activity, placing a mathematical model of ictogenicity into the network and then computing how often such network generates seizures in silico. We considered data from 26 people with JME and 26 healthy controls.
Results
We found that resting-state MEG functional networks from people with JME are characterized by a higher propensity to generate seizures (i.e., higher BNI) than those from healthy controls. We found a classification accuracy of 73%.
Conclusions
The BNI framework is applicable to MEG and was capable of differentiating people with epilepsy from healthy controls.
Significance
The BNI framework may be applied to resting-state MEG to aid in epilepsy diagnosis.
更多查看译文
关键词
Epilepsy diagnosis,Juvenile myoclonic epilepsy,Biomarker,MEG,Functional connectivity,Phenomenological model
AI 理解论文
溯源树
样例
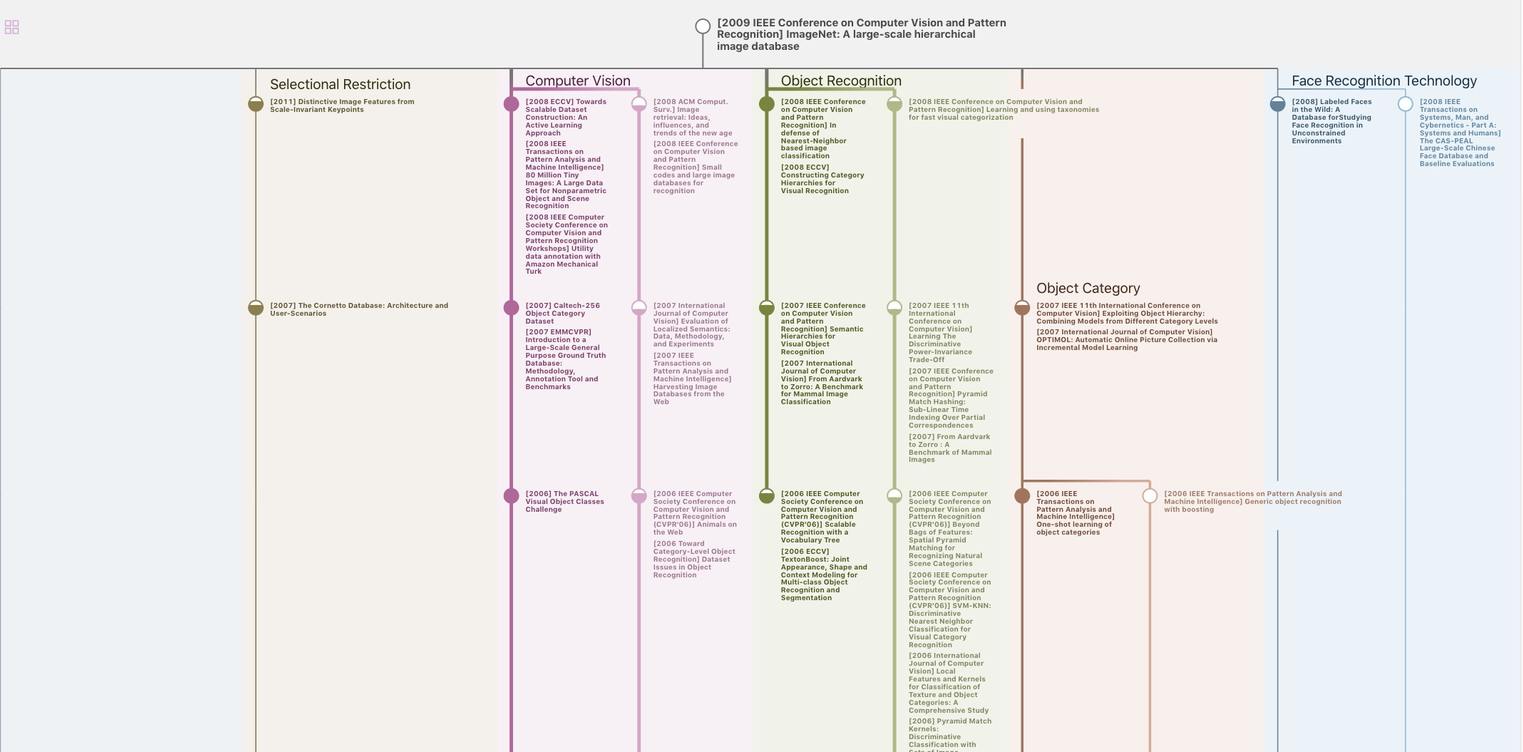
生成溯源树,研究论文发展脉络
Chat Paper
正在生成论文摘要