Inter-Patient Classification with Encoded Peripheral Pulse Series and Multi-Task Fusion CNN: Application in Type 2 Diabetes
IEEE journal of biomedical and health informatics(2021)
摘要
Diabetes mellitus, a chronic disease associated with elevated accumulation of glucose in the blood, is generally diagnosed through an invasive blood test such as oral glucose tolerance test (OGTT). An effective method is proposed to test type 2 diabetes using peripheral pulse waves, which can be measured fast, simply and inexpensively by a force sensor on the wrist over the radial artery. A self-designed pulse waves collection platform includes a wristband, force sensor, cuff, air tubes, and processing module. A dataset was acquired clinically for more than one year by practitioners. A group of 127 healthy candidates and 85 patients with type 2 diabetes, all between the ages of 45 and 70, underwent assessments in both OGTT and pulse data collection at wrist arteries. After preprocessing, pulse series were encoded as images using the Gramian angular field (GAF), Markov transition field (MTF), and recurrence plots (RPs). A four-layer multi-task fusion convolutional neural network (CNN) was developed for feature recognition, the network was well-trained within 30 minutes based on our server. Compared to single-task CNN, multi-task fusion CNN was proved better in classification accuracy for nine of twelve settings with empirically selected parameters. The results show that the best accuracy reached 90.6% using an RP with threshold ∈ of 6000, which is competitive to that using state-of-the-art algorithms in diabetes classification.
更多查看译文
关键词
Biomedical informatics,convolutional neural networks,peripheral pulse,time series,type 2 diabetes
AI 理解论文
溯源树
样例
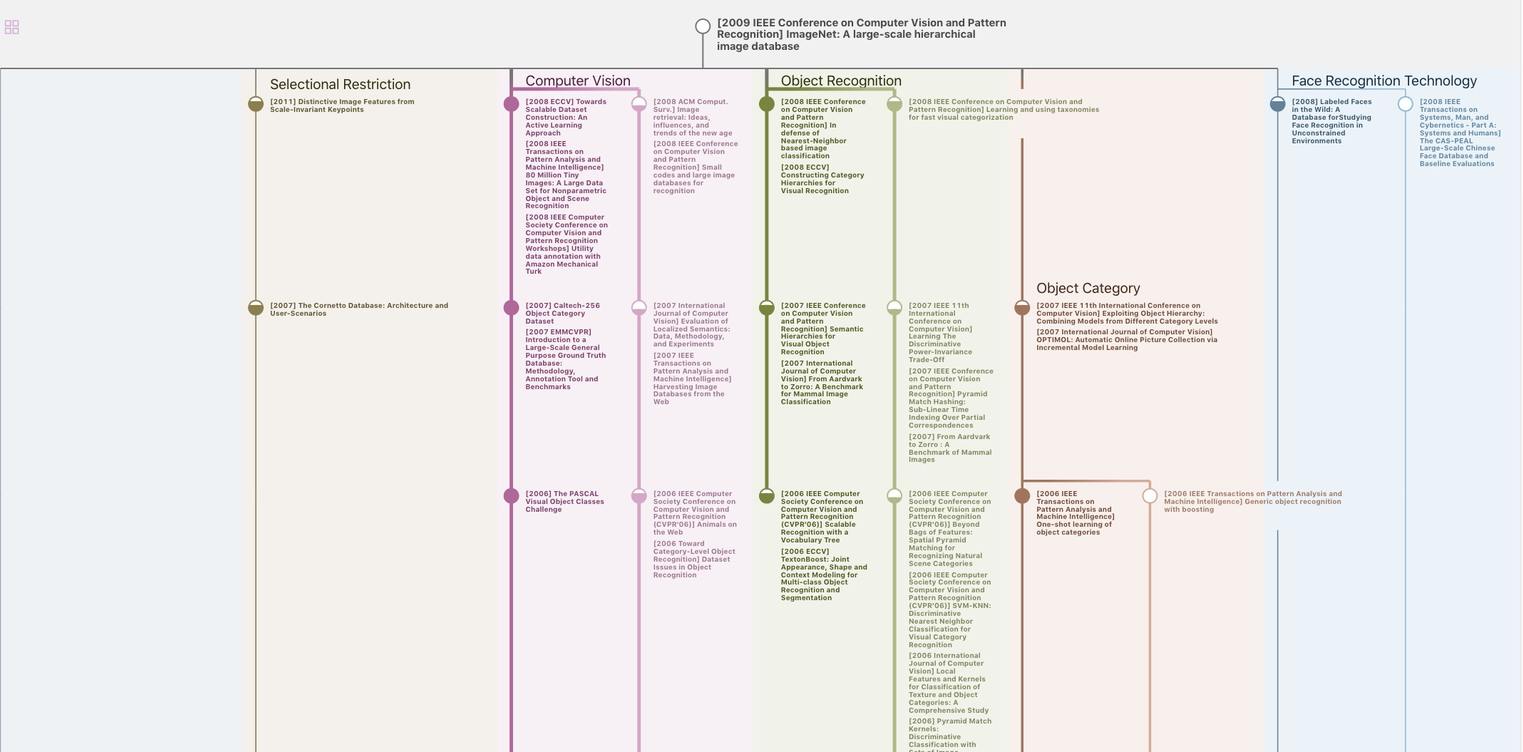
生成溯源树,研究论文发展脉络
Chat Paper
正在生成论文摘要