Traffic Flow Forecasting With Spatial-Temporal Graph Diffusion Network
THIRTY-FIFTH AAAI CONFERENCE ON ARTIFICIAL INTELLIGENCE, THIRTY-THIRD CONFERENCE ON INNOVATIVE APPLICATIONS OF ARTIFICIAL INTELLIGENCE AND THE ELEVENTH SYMPOSIUM ON EDUCATIONAL ADVANCES IN ARTIFICIAL INTELLIGENCE(2021)
摘要
Accurate forecasting of citywide traffic flow has been playing critical role in a variety of spatial-temporal mining applications, such as intelligent traffic control and public risk assessment. While previous work has made significant efforts to learn traffic temporal dynamics and spatial dependencies, two key limitations exist in current models. First, only the neighboring spatial correlations among adjacent regions are considered in most existing methods, and the global interregion dependency is ignored. Additionally, these methods fail to encode the complex traffic transition regularities exhibited with time-dependent and multi-resolution in nature. To tackle these challenges, we develop a new traffic prediction framework-Spatial-Temporal Graph Diffusion Network (ST-GDN). In particular, ST-GDN is a hierarchically structured graph neural architecture which learns not only the local region-wise geographical dependencies, but also the spatial semantics from a global perspective. Furthermore, a multiscale attention network is developed to empower ST-GDN with the capability of capturing multi-level temporal dynamics. Experiments on several real-life traffic datasets demonstrate that ST-GDN outperforms different types of state-ofthe-art baselines. Source codes of implementations are available at hups://github.com/jill001/ST-GDN.
更多查看译文
关键词
traffic,forecasting,diffusion,graph,flow,spatial-temporal
AI 理解论文
溯源树
样例
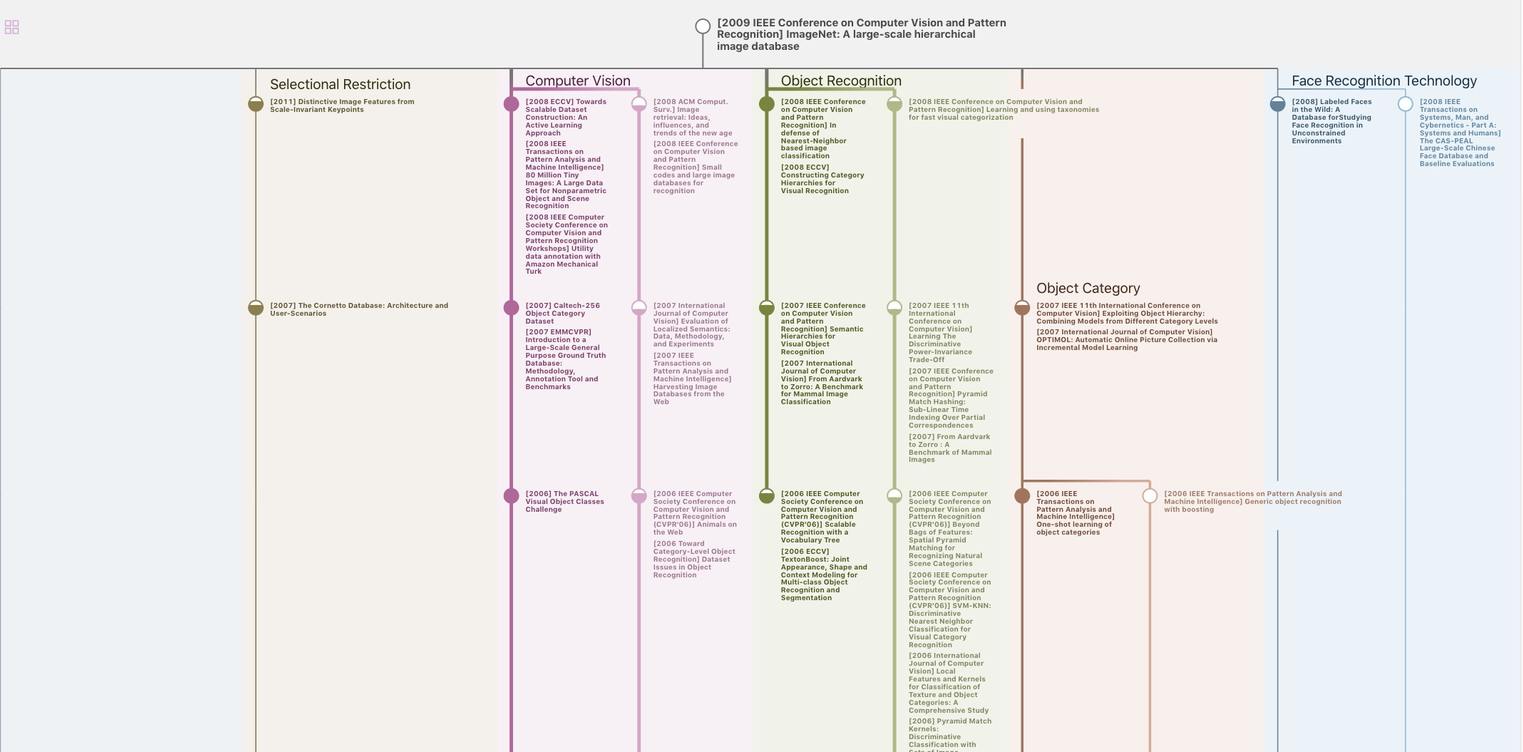
生成溯源树,研究论文发展脉络
Chat Paper
正在生成论文摘要