Machine Unlearning via Algorithmic Stability.
COLT(2021)
摘要
We study the problem of machine unlearning and identify a notion of algorithmic stability, Total Variation (TV) stability, which we argue, is suitable for the goal of exact unlearning. For convex risk minimization problems, we design TV-stable algorithms based on noisy Stochastic Gradient Descent (SGD). Our key contribution is the design of corresponding efficient unlearning algorithms, which are based on constructing a (maximal) coupling of Markov chains for the noisy SGD procedure. To understand the trade-offs between accuracy and unlearning efficiency, we give upper and lower bounds on excess empirical and populations risk of TV stable algorithms for convex risk minimization. Our techniques generalize to arbitrary non-convex functions, and our algorithms are differentially private as well.
更多查看译文
关键词
machine unlearning,stability
AI 理解论文
溯源树
样例
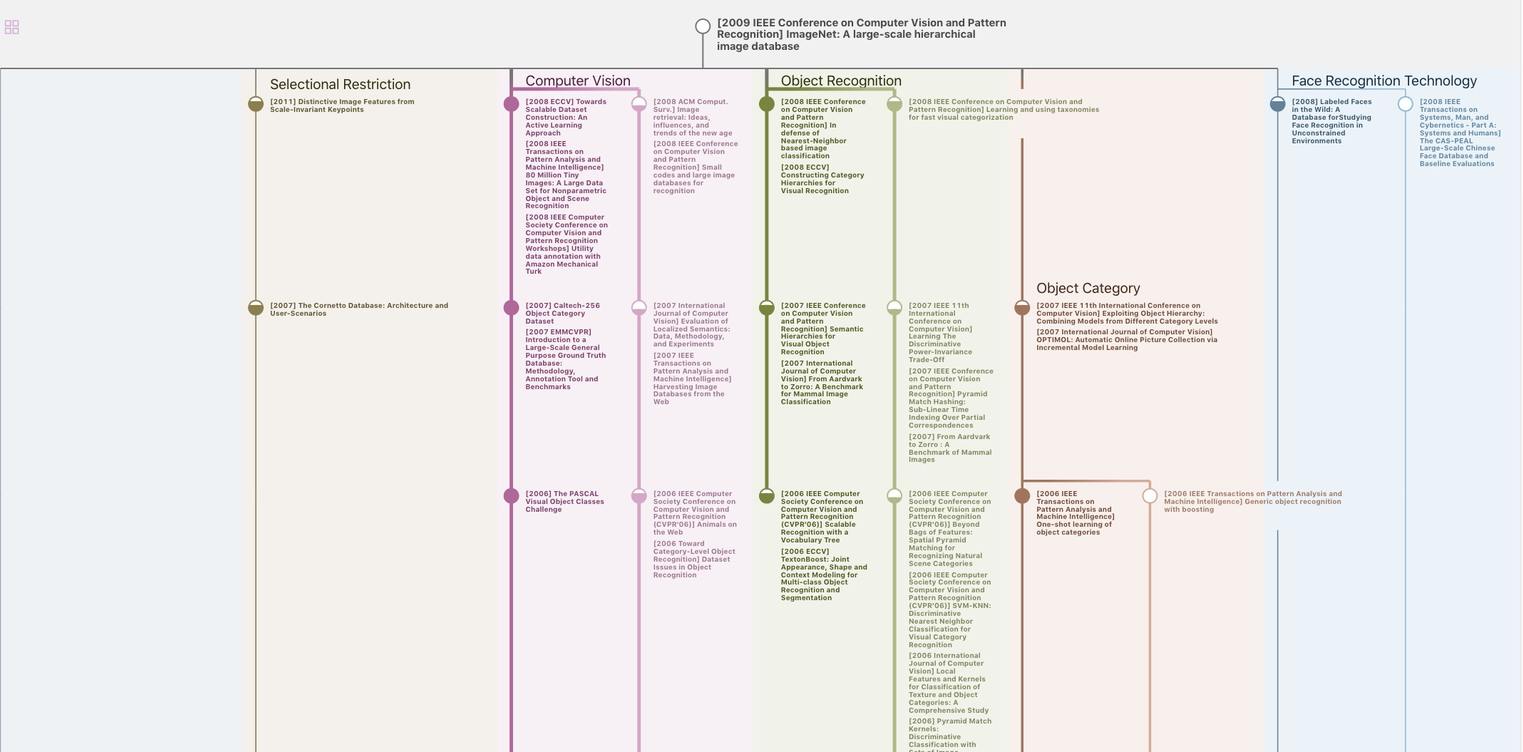
生成溯源树,研究论文发展脉络
Chat Paper
正在生成论文摘要