Batch Bayesian Optimization on Permutations using the Acquisition Weighted Kernel
arxiv(2023)
摘要
In this work we propose a batch Bayesian optimization method for combinatorial problems on permutations, which is well suited for expensive-to-evaluate objectives. We first introduce LAW, an efficient batch acquisition method based on determinantal point processes using the acquisition weighted kernel. Relying on multiple parallel evaluations, LAW enables accelerated search on combinatorial spaces. We then apply the framework to permutation problems, which have so far received little attention in the Bayesian Optimization literature, despite their practical importance. We call this method LAW2ORDER. On the theoretical front, we prove that LAW2ORDER has vanishing simple regret by showing that the batch cumulative regret is sublinear. Empirically, we assess the method on several standard combinatorial problems involving permutations such as quadratic assignment, flowshop scheduling and the traveling salesman, as well as on a structure learning task.
更多查看译文
关键词
Bayesian Optimization,Batch Acquisition,Permutation,Bandit,Regret Analysis,Information Gain,Determinantal Point Processes
AI 理解论文
溯源树
样例
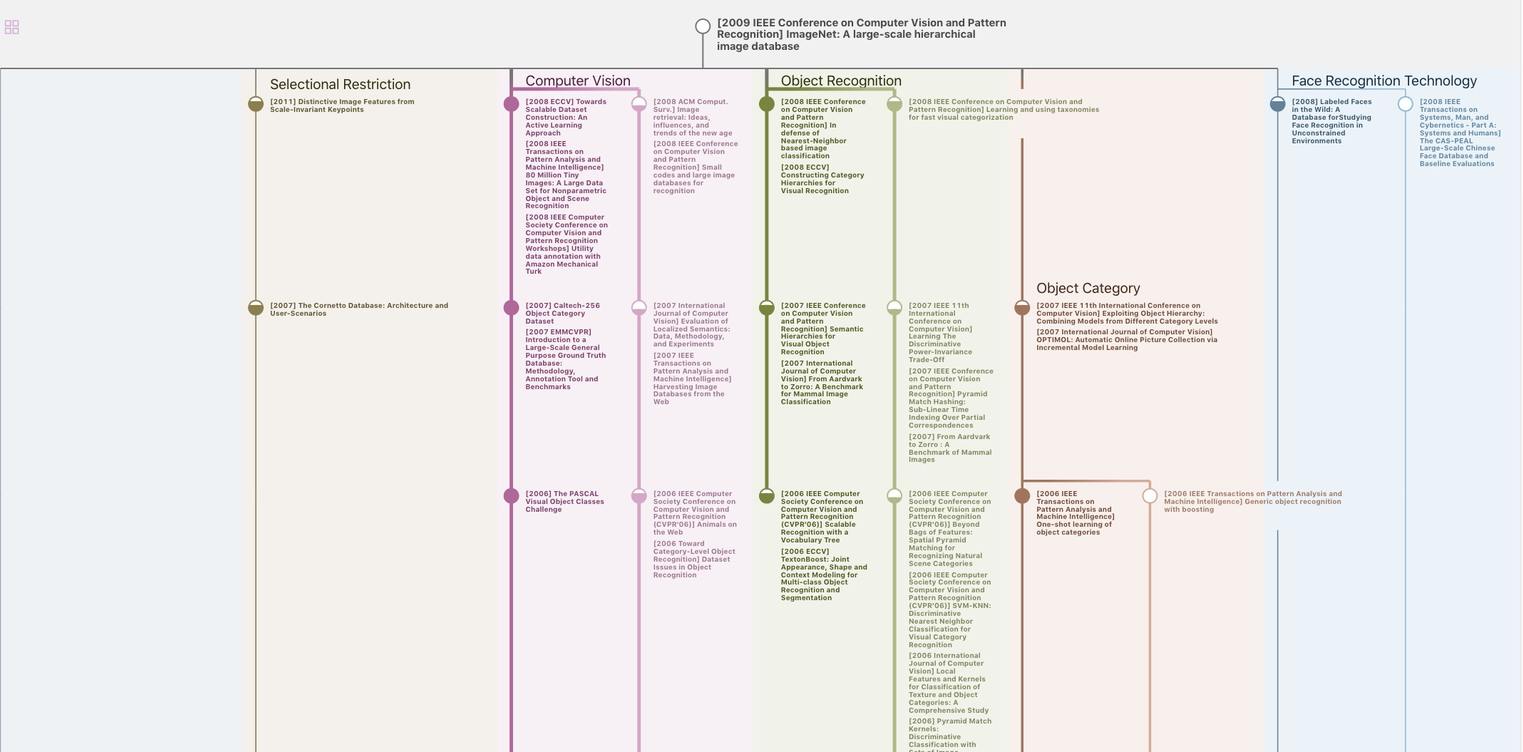
生成溯源树,研究论文发展脉络
Chat Paper
正在生成论文摘要