A complete description of thermodynamic stabilities of molecular crystals
PROCEEDINGS OF THE NATIONAL ACADEMY OF SCIENCES OF THE UNITED STATES OF AMERICA(2022)
摘要
Predictions of relative stabilities of (competing) molecular crystals are of great technological relevance, most notably for the pharmaceutical industry. However, they present a long-standing challenge for modeling, as often minuscule free energy differences are sensitively affected by the description of electronic structure, the statistical mechanics of the nuclei and the cell, and thermal expansion. The importance of these effects has been individually established, but rigorous free energy calculations for general molecular compounds, which simultaneously account for all effects, have hitherto not been computationally viable. Here we present an efficient "end to end" framework that seamlessly combines state-of-the art electronic structure calculations, machine-learning potentials, and advanced free energy methods to calculate ab initio Gibbs free energies for general organic molecular materials. The facile generation of machine-learning potentials for a diverse set of polymorphic compounds-benzene, glycine, and succinic acid-and predictions of thermodynamic stabilities in qualitative and quantitative agreement with experiments highlight that predictive thermodynamic studies of industrially relevant molecular materials are no longer a daunting task.
更多查看译文
关键词
statistical mechanics,machine learning,ab initio thermodynamics,polymorphism
AI 理解论文
溯源树
样例
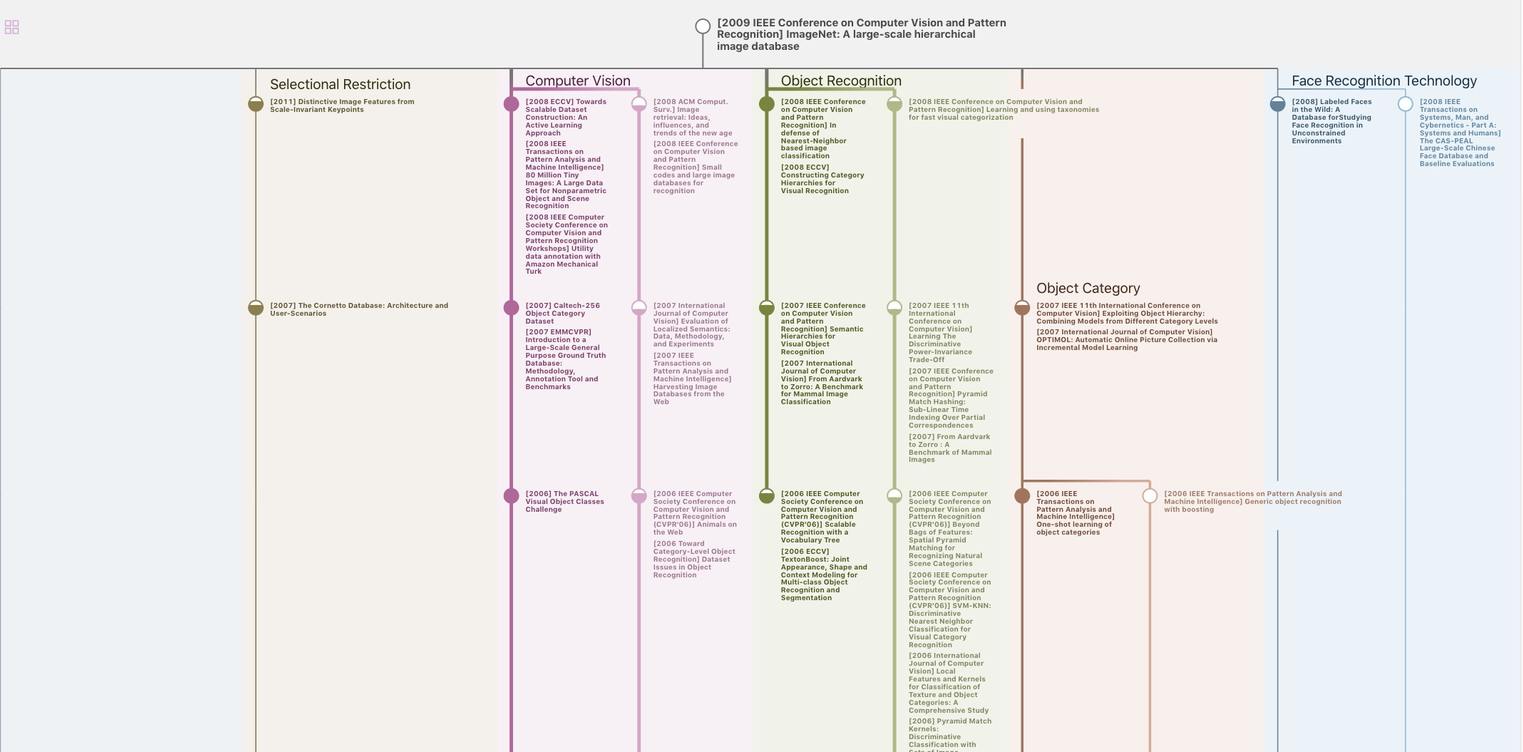
生成溯源树,研究论文发展脉络
Chat Paper
正在生成论文摘要