PURSUhInT: In Search of Informative Hint Points Based on Layer Clustering for Knowledge Distillation
Expert Systems with Applications(2023)
摘要
One of the most efficient methods for model compression is hint distillation, where the student model is injected with information (hints) from several different layers of the teacher model. Although the selection of hint points can drastically alter the compression performance, conventional distillation approaches overlook this fact and use the same hint points as in the early studies. Therefore, we propose a clustering based hint selection methodology, where the layers of teacher model are clustered with respect to several metrics and the cluster centers are used as the hint points. Our method is applicable for any student network, once it is applied on a chosen teacher network. The proposed approach is validated in CIFAR-100 and ImageNet datasets, using various teacher-student pairs and numerous hint distillation methods. Our results show that hint points selected by our algorithm results in superior compression performance compared to state-of-the-art knowledge distillation algorithms on the same student models and datasets.
更多查看译文
关键词
Model compression,Knowledge distillation,Hint selection,Feature matching
AI 理解论文
溯源树
样例
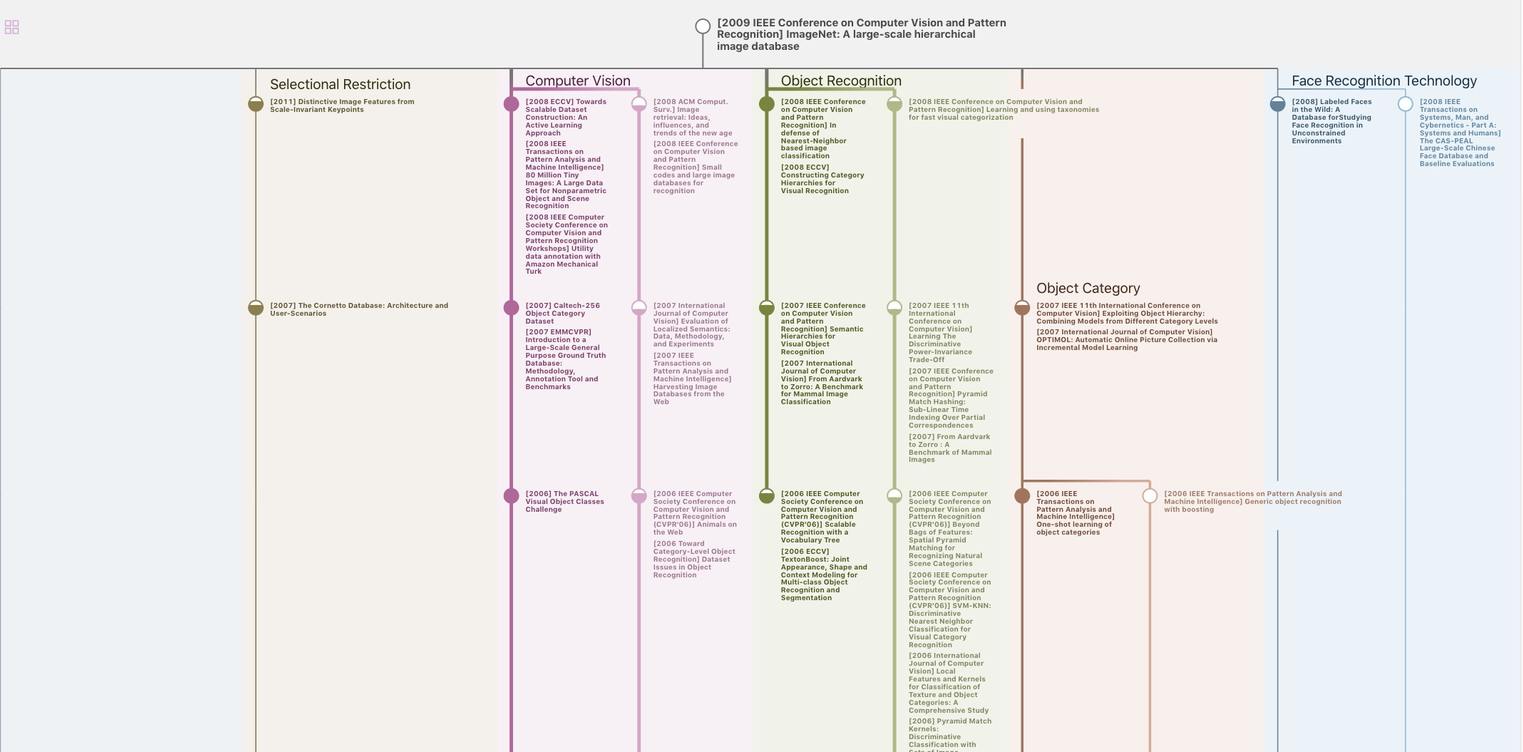
生成溯源树,研究论文发展脉络
Chat Paper
正在生成论文摘要