Software-Supported Audits of Decision-Making Systems: Testing Google and Facebook's Political Advertising Policies
Proceedings of the ACM on Human-Computer Interaction(2022)
摘要
AbstractHow can society understand and hold accountable complex human and algorithmic decision-making systems whose systematic errors are opaque to the public? These systems routinely make decisions on individual rights and well-being, and on protecting society and the democratic process. Practical and statistical constraints on external audits--such as dimensional complexity--can lead researchers and regulators to miss important sources of error in these complex decision-making systems. In this paper, we design and implement a software-supported approach to audit studies that auto-generates audit materials and coordinates volunteer activity. We implemented this software in the case of political advertising policies enacted by Facebook and Google during the 2018 U.S. election. Guided by this software, a team of volunteers posted 477 auto-generated ads and analyzed the companies' actions, finding systematic errors in how companies enforced policies. We find that software can overcome some common constraints of audit studies, within limitations related to sample size and volunteer capacity.
更多查看译文
AI 理解论文
溯源树
样例
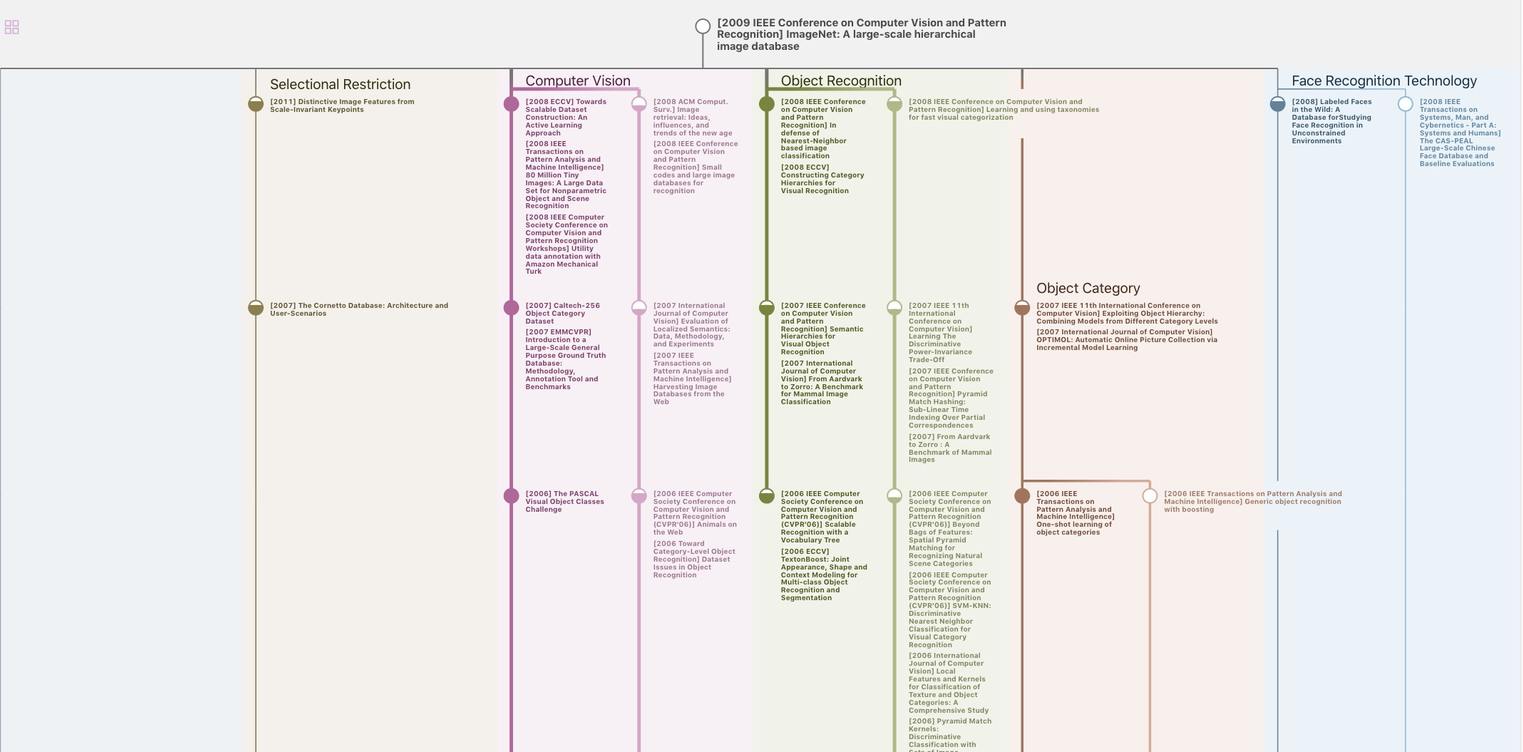
生成溯源树,研究论文发展脉络
Chat Paper
正在生成论文摘要