Scalable Causal Transfer Learning
arxiv(2021)
摘要
One of the most important problems in transfer learning is the task of domain adaptation, where the goal is to apply an algorithm trained in one or more source domains to a different (but related) target domain. This paper deals with domain adaptation in the presence of covariate shift while there exist invariances across domains. A main limitation of existing causal inference methods for solving this problem is scalability. To overcome this difficulty, we propose SCTL, an algorithm that avoids an exhaustive search and identifies invariant causal features across the source and target domains based on Markov blanket discovery. SCTL does not require to have prior knowledge of the causal structure, the type of interventions, or the intervention targets. There is an intrinsic locality associated with SCTL that makes SCTL practically scalable and robust because local causal discovery increases the power of computational independence tests and makes the task of domain adaptation computationally tractable. We show the scalability and robustness of SCTL for domain adaptation using synthetic and real data sets in low-dimensional and high-dimensional settings.
更多查看译文
关键词
scalable causal domain adaptation
AI 理解论文
溯源树
样例
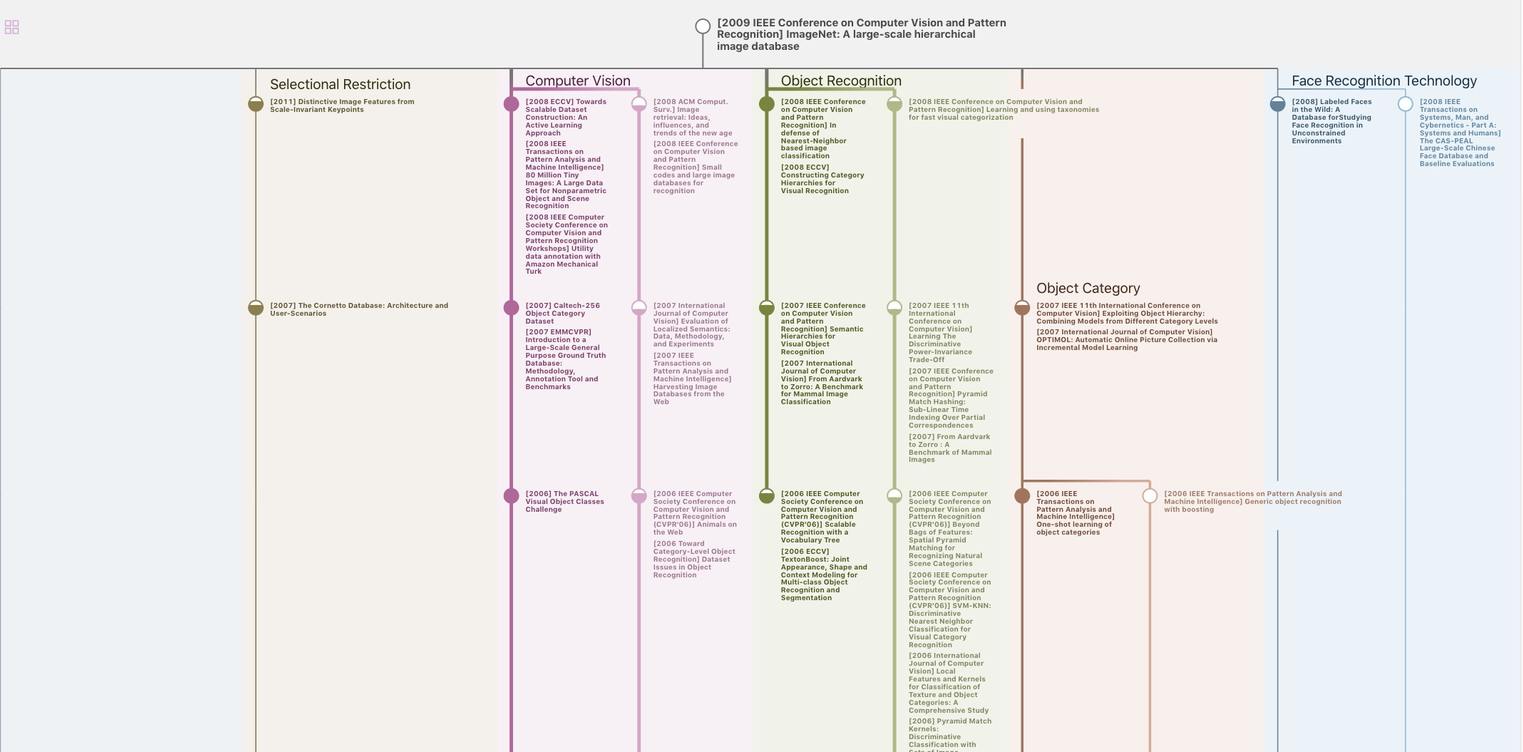
生成溯源树,研究论文发展脉络
Chat Paper
正在生成论文摘要