Explore User Neighborhood for Real-time E-commerce Recommendation
2021 IEEE 37TH INTERNATIONAL CONFERENCE ON DATA ENGINEERING (ICDE 2021)(2021)
摘要
Recommender systems play a vital role in modern online services, such as Amazon and Taobao. Traditional personalized methods, which focus on user-item (UI) relations, have been widely applied in industrial settings, owing to their efficiency and effectiveness. Despite their success, we argue that these approaches ignore local information hidden in similar users. To tackle this problem, user-based methods exploit similar user relations to make recommendations in a local perspective. Nevertheless, traditional user-based methods, like userKNN and matrix factorization, are intractable to be deployed in the real-time applications since such transductive models have to be recomputed or retrained with any new interaction. To overcome this challenge, we propose a framework called self-complementary collaborative filtering (SCCF) which can make recommendations with both global and local information in real time. On the one hand, it utilizes UI relations and user neighborhood to capture both global and local information. On the other hand, it can identify similar users for each user in real time by inferring user representations on the fiy with an inductive model. The proposed framework can be seamlessly incorporated into existing inductive UI approach and benefit from user neighborhood with little additional computation. It is also the first attempt to apply user-based methods in real-time settings. The effectiveness and efficiency of SCCF are demonstrated through extensive offline experiments on four public datasets, as well as a large scale online A/B test in Taobao.
更多查看译文
关键词
Candidate generation,User neighborhood,Real time
AI 理解论文
溯源树
样例
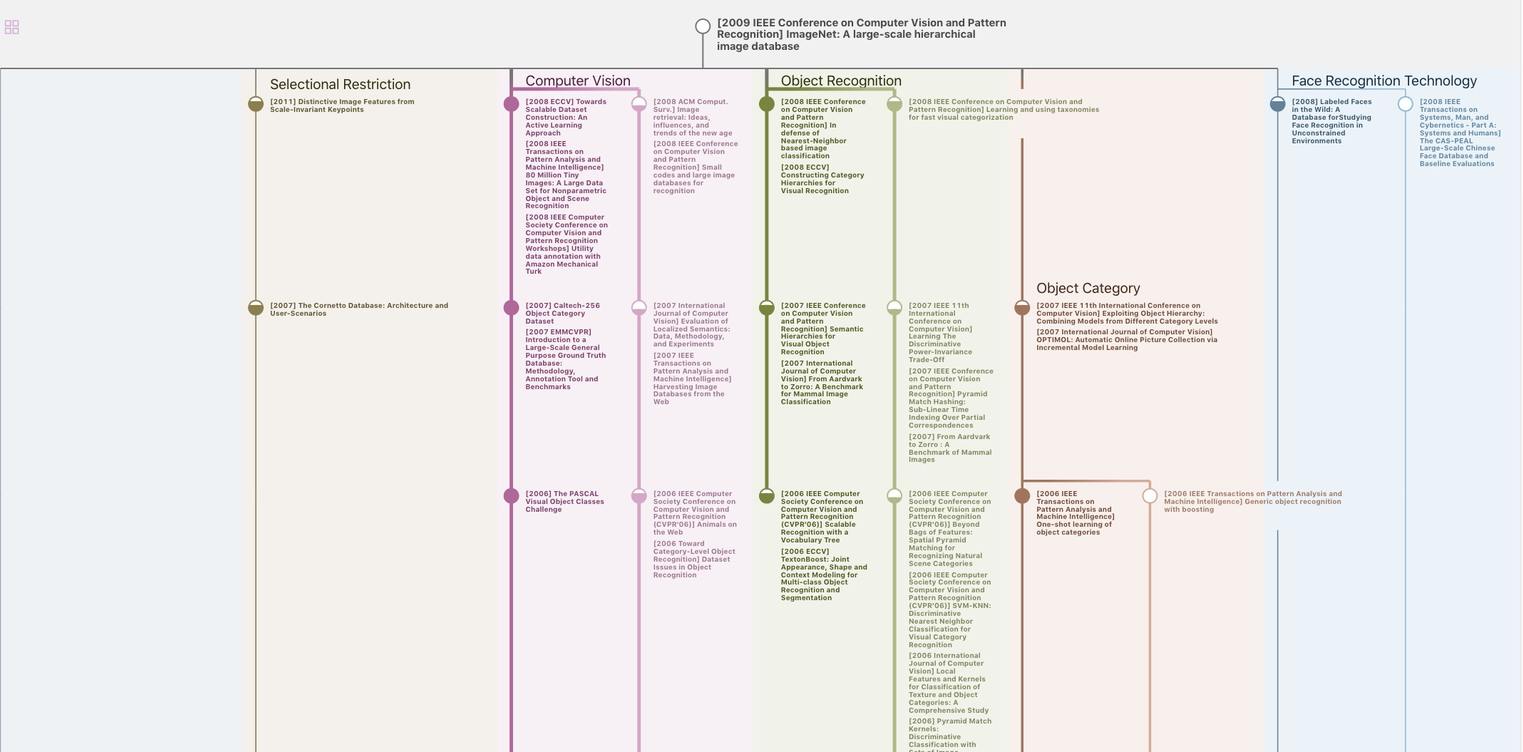
生成溯源树,研究论文发展脉络
Chat Paper
正在生成论文摘要