Dual Attention Suppression Attack: Generate Adversarial Camouflage in Physical World
2021 IEEE/CVF CONFERENCE ON COMPUTER VISION AND PATTERN RECOGNITION, CVPR 2021(2021)
摘要
Deep learning models are vulnerable to adversarial examples. As a more threatening type for practical deep learning systems, physical adversarial examples have received extensive research attention in recent years. However, without exploiting the intrinsic characteristics such as model-agnostic and human-specific patterns, existing works generate weak adversarial perturbations in the physical world, which fall short of attacking across different models and show visually suspicious appearance. Motivated by the viewpoint that attention reflects the intrinsic characteristics of the recognition process, this paper proposes the Dual Attention Suppression (DAS) attack to generate visually-natural physical adversarial camouflages with strong transferability by suppressing both model and human attention. As for attacking, we generate transferable adversarial camouflages by distracting the model-shared similar attention patterns from the target to non-target regions. Meanwhile, based on the fact that human visual attention always focuses on salient items (e.g., suspicious distortions), we evade the human-specific bottom-up attention to generate visuallynatural camouflages which are correlated to the scenario context. We conduct extensive experiments in both the digital and physical world for classification and detection tasks on up-to-date models (e.g., Yolo-V5) and demonstrate that our method outperforms state-of-the-art methods.(1)
更多查看译文
关键词
visually-natural camouflages,digital world,physical world,generate adversarial camouflage,deep learning models,threatening type,physical adversarial examples,extensive research attention,intrinsic characteristics,human-specific patterns,weak adversarial perturbations,visually-natural physical adversarial camouflages,human attention,transferable adversarial camouflages,model-shared similar attention patterns,nontarget regions,human visual attention,visually suspicious appearance,dual attention suppression attack
AI 理解论文
溯源树
样例
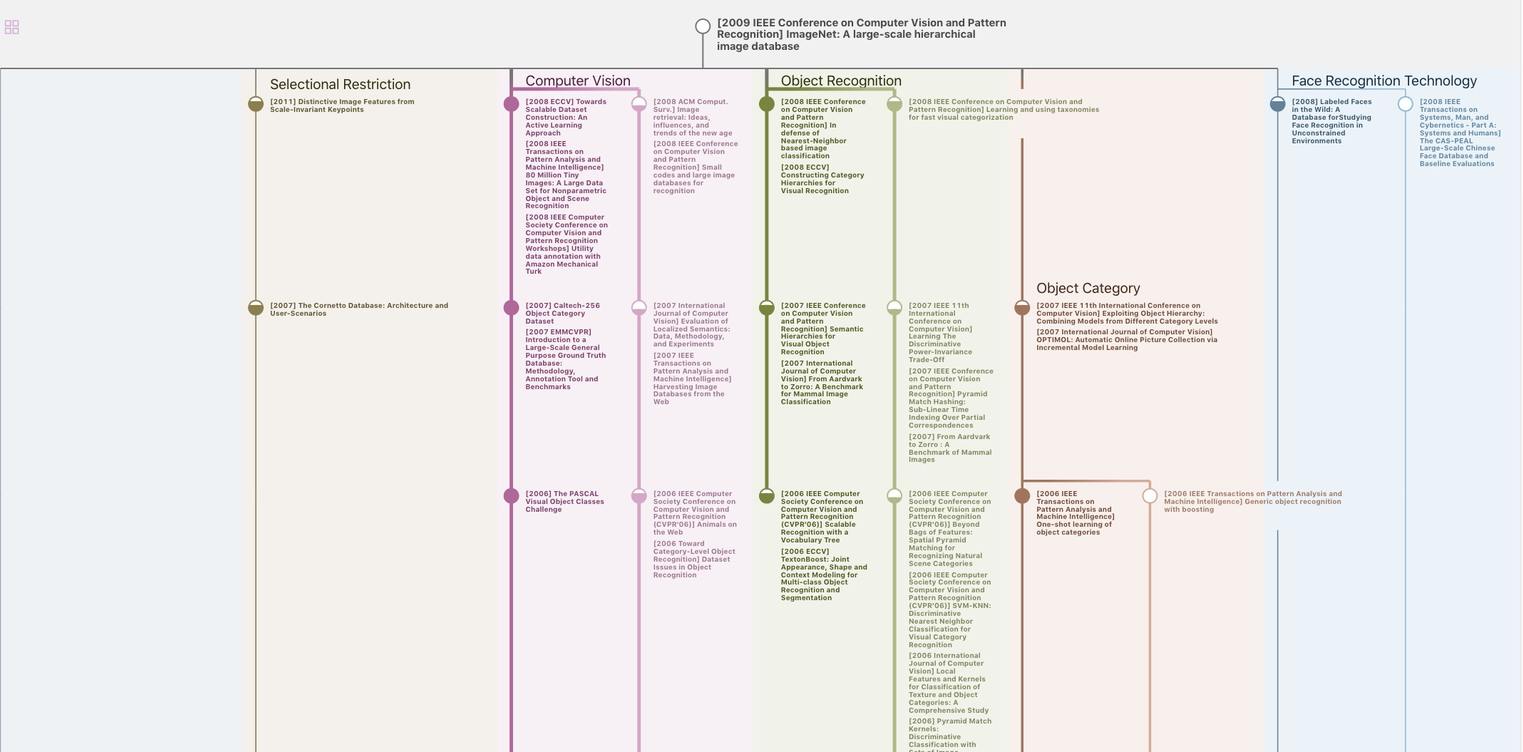
生成溯源树,研究论文发展脉络
Chat Paper
正在生成论文摘要