Learning With Hyperspherical Uniformity
24TH INTERNATIONAL CONFERENCE ON ARTIFICIAL INTELLIGENCE AND STATISTICS (AISTATS)(2021)
摘要
Due to the over-parameterization nature, neural networks are a powerful tool for nonlinear function approximation. In order to achieve good generalization on unseen data, a suitable inductive bias is of great importance for neural networks. One of the most straightforward ways is to regularize the neural network with some additional objectives. l(2) regularization serves as a standard regularization for neural networks. Despite its popularity, it essentially regularizes one dimension of the individual neuron, which is not strong enough to control the capacity of highly over-parameterized neural networks. Motivated by this, hyperspherical uniformity is proposed as a novel family of relational regularizations that impact the interaction among neurons. We consider several geometrically distinct ways to achieve hyperspherical uniformity. The effectiveness of hyperspherical uniformity is justified by theoretical insights and empirical evaluations.
更多查看译文
关键词
hyperspherical uniformity,learning
AI 理解论文
溯源树
样例
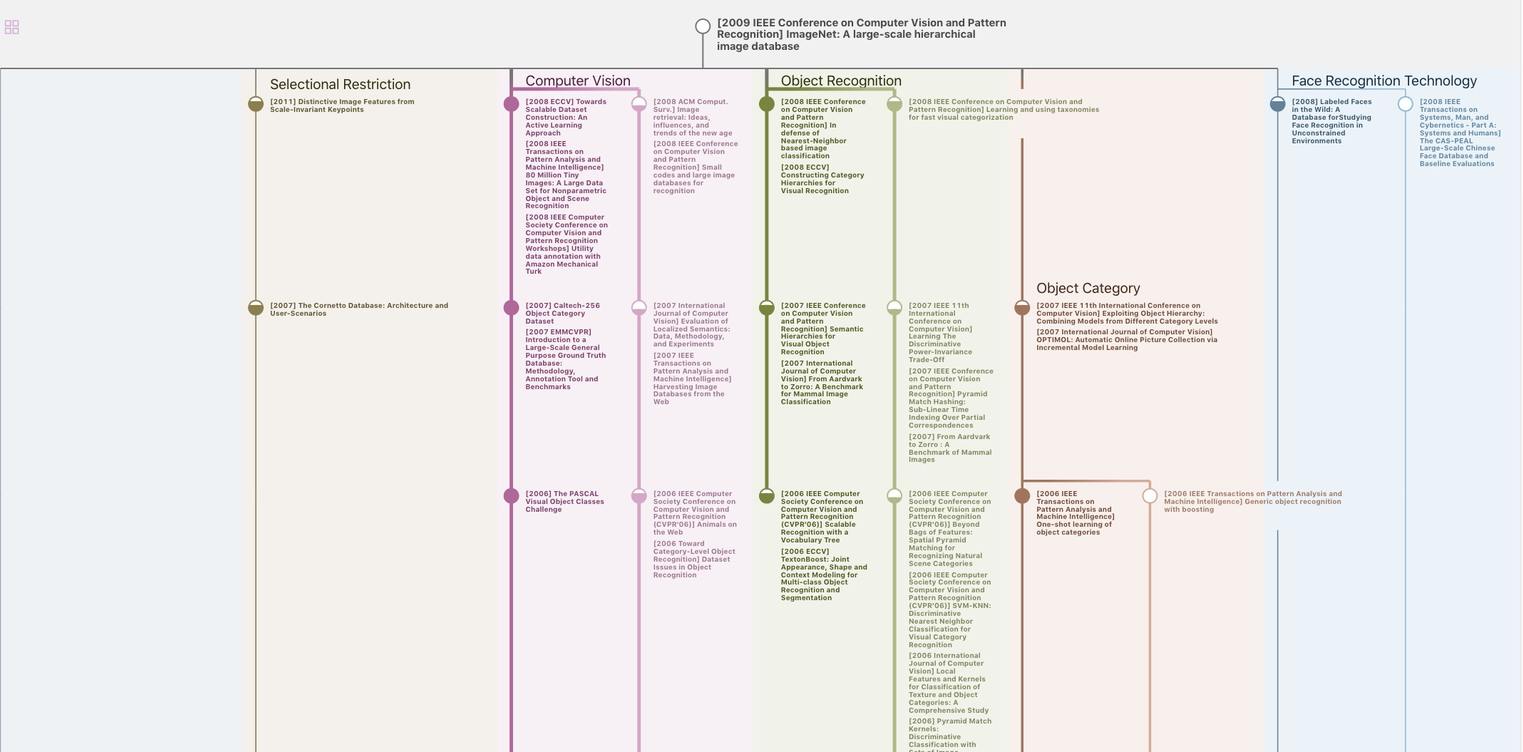
生成溯源树,研究论文发展脉络
Chat Paper
正在生成论文摘要