MetaSCI: Scalable and Adaptive Reconstruction for Video Compressive Sensing
2021 IEEE/CVF CONFERENCE ON COMPUTER VISION AND PATTERN RECOGNITION, CVPR 2021(2021)
摘要
To capture high-speed videos using a two-dimensional detector, video snapshot compressive imaging (SCI) is a promising system, where the video frames are coded by different masks and then compressed to a snapshot measurement. Following this, efficient algorithms are desired to reconstruct the high-speed frames, where the state-of-the-art results are achieved by deep learning networks. However, these networks are usually trained for specific small-scale masks and often have high demands of training time and GPU memory, which are hence not flexible to i) a new mask with the same size and ii) a larger-scale mask. We address these challenges by developing a Meta Modulated Convolutional Network for SCI reconstruction, dubbed MetaSCI. MetaSCI is composed of a shared backbone for different masks, and light-weight meta-modulation parameters to evolve to different modulation parameters for each mask, thus having the properties of fast adaptation to new masks (or systems) and ready to scale to large data. Extensive simulation and real data results demonstrate the superior performance of our proposed approach.
更多查看译文
关键词
video compressive
AI 理解论文
溯源树
样例
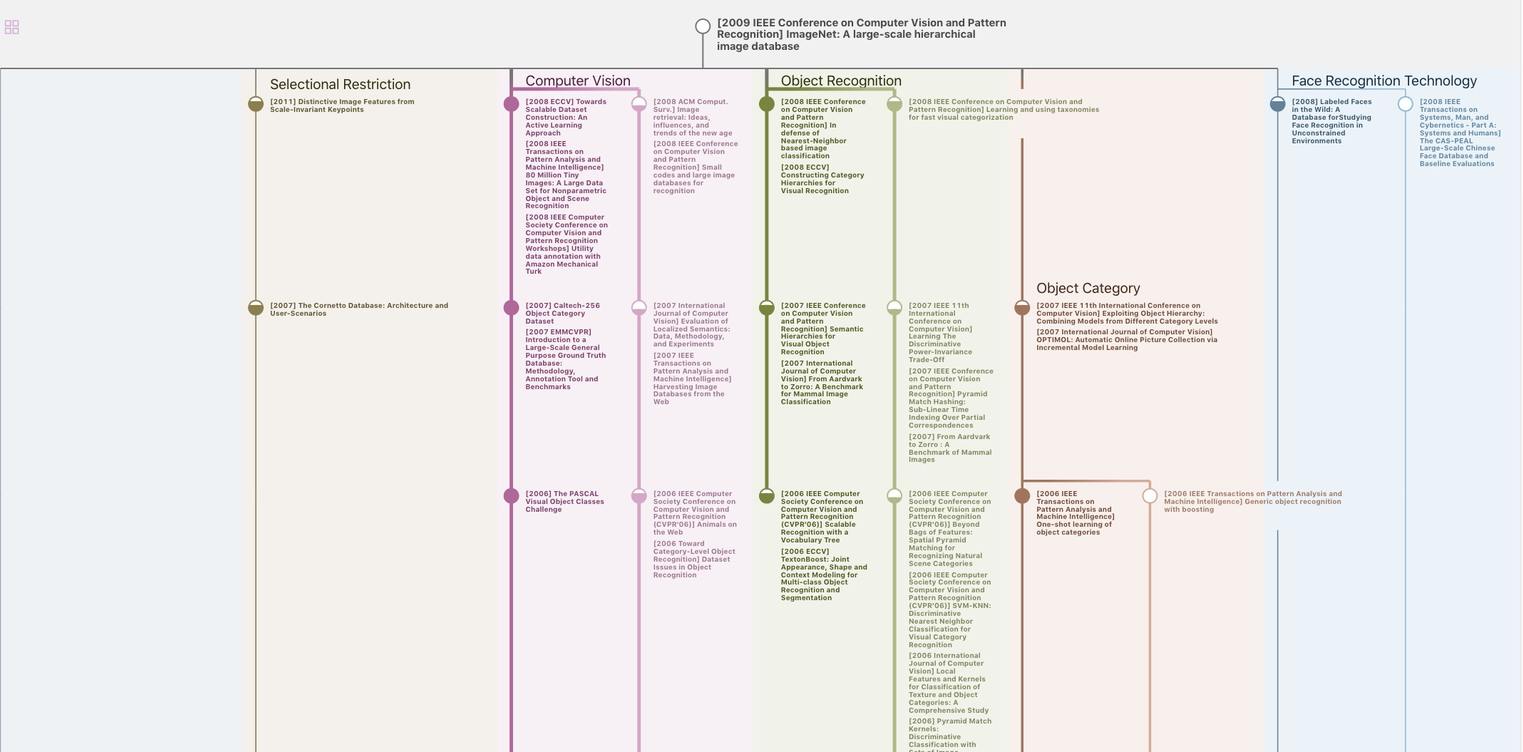
生成溯源树,研究论文发展脉络
Chat Paper
正在生成论文摘要