Asymptotic approximation of the likelihood of stationary determinantal point processes
arxiv(2023)
摘要
Continuous determinantal point processes (DPPs) are a class of repulsive point processes on Double-struck capital Rd$$ {\mathbb{R}}<^>d $$ with many statistical applications. Although an explicit expression of their density is known, it is too complicated to be used directly for maximum likelihood estimation. In the stationary case, an approximation using Fourier series has been suggested, but it is limited to rectangular observation windows and no theoretical results support it. In this contribution, we investigate a different way to approximate the likelihood by looking at its asymptotic behavior when the observation window grows toward Double-struck capital Rd$$ {\mathbb{R}}<^>d $$. This new approximation is not limited to rectangular windows, is faster to compute than the previous one, does not require any tuning parameter, and some theoretical justifications are provided. It moreover provides an explicit formula for estimating the asymptotic variance of the associated estimator. The performances are assessed in a simulation study on standard parametric models on Double-struck capital Rd$$ {\mathbb{R}}<^>d $$ and compare favorably to common alternative estimation methods for continuous DPPs.
更多查看译文
关键词
determinantal point process,maximum likelihood inference,repulsive
AI 理解论文
溯源树
样例
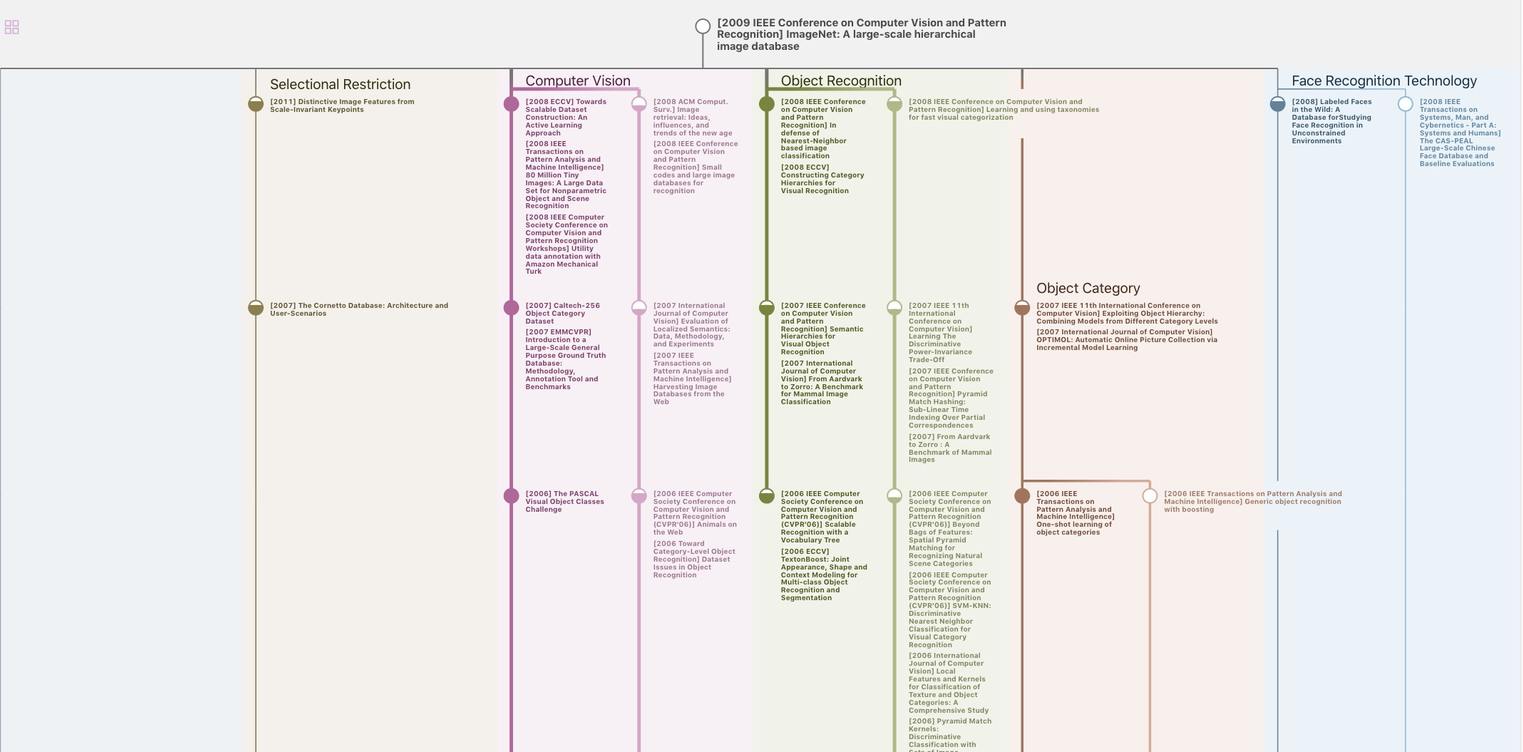
生成溯源树,研究论文发展脉络
Chat Paper
正在生成论文摘要