Machine Learning Studies Of Non-Coding Rnas Based On Artificially Constructed Training Data
PROCEEDINGS OF THE 14TH INTERNATIONAL JOINT CONFERENCE ON BIOMEDICAL ENGINEERING SYSTEMS AND TECHNOLOGIES, VOL 3: BIOINFORMATICS(2021)
摘要
Machine learning (ML) methods are often used to identify members of non-coding RNA classes such as microRNAs or snoRNAs. However, ML methods have not been successfully used for homology search tasks. A systematic evaluation of ML in homology search requires large, controlled, and known ground truth test sets, and thus, methods to construct large realistic artificial data sets. Here we describe a method for producing sets of arbitrarily large and diverse snoRNA sequences based on artificial evolution. These are then used to evaluate supervised ML methods (Support Vector Machine, Artificial Neural Network, and Random Forest) for snoRNA detection in a chordate genome. Our results indicate that ML approaches can indeed be competitive also for homology search.
更多查看译文
关键词
Small Nucleolar RNAs (snoRNAs), Non-Coding RNA Inference, Machine Learning, Chordate Genome
AI 理解论文
溯源树
样例
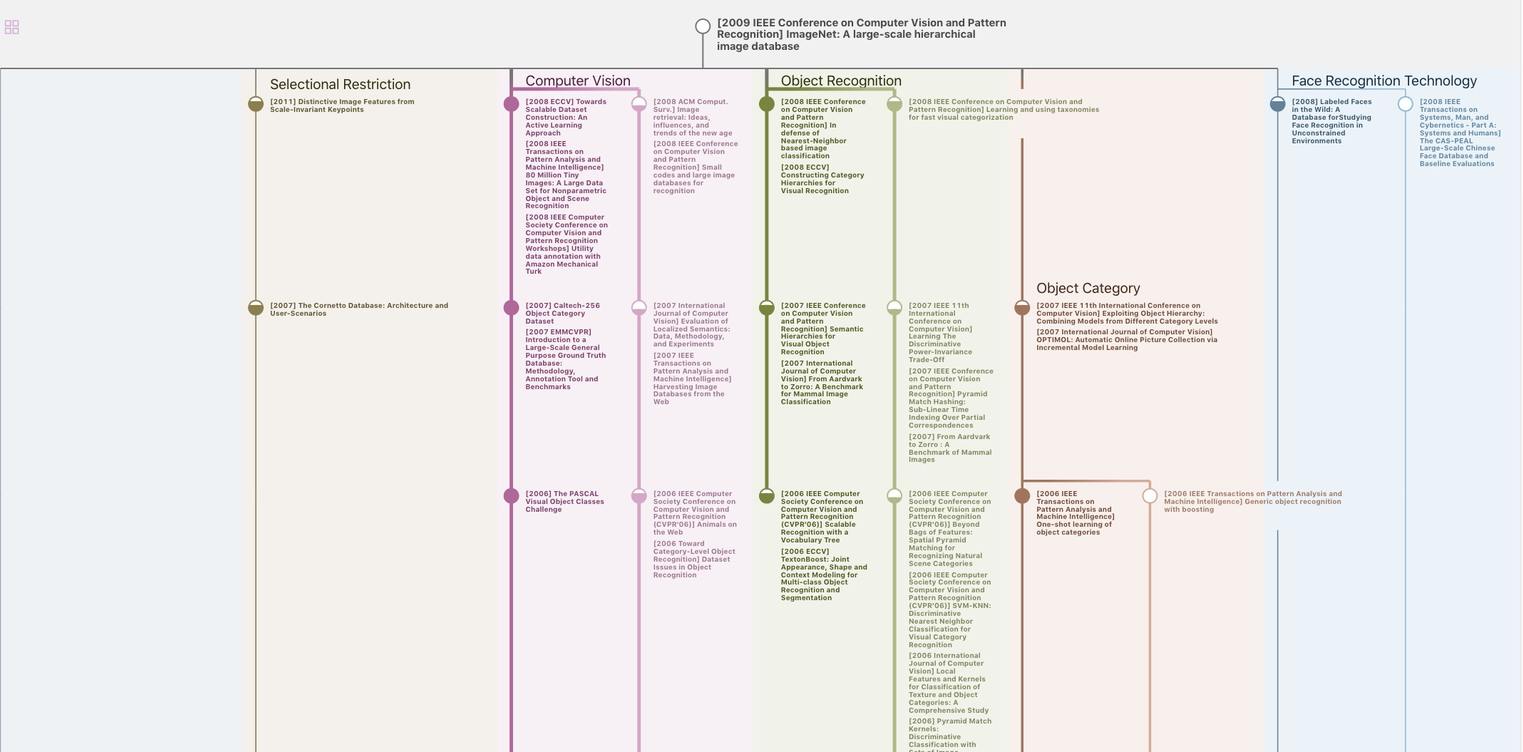
生成溯源树,研究论文发展脉络
Chat Paper
正在生成论文摘要