Multi-Attentional Deepfake Detection.
CVPR(2021)
摘要
Face forgery by deepfake is widely spread over the internet and has raised severe societal concerns. Recently, how to detect such forgery contents has become a hot research topic and many deepfake detection methods have been proposed. Most of them model deepfake detection as a vanilla binary classification problem, i.e, first use a backbone network to extract a global feature and then feed it into a binary classifier (real/fake). But since the difference between the real and fake images in this task is often subtle and local, we argue this vanilla solution is not optimal. In this paper, we instead formulate deepfake detection as a fine-grained classification problem and propose a new multi-attentional deepfake detection network. Specifically, it consists of three key components: 1) multiple spatial attention heads to make the network attend to different local parts; 2) textural feature enhancement block to zoom in the subtle artifacts in shallow features; 3) aggregate the low-level textural feature and high-level semantic features guided by the attention maps. Moreover, to address the learning difficulty of this network, we further introduce a new regional independence loss and an attention guided data augmentation strategy. Through extensive experiments on different datasets, we demonstrate the superiority of our method over the vanilla binary classifier counterparts, and achieve state-of-the-art performance.
更多查看译文
关键词
shallow features,low-level textural feature,high-level semantic features,attention maps,attention guided data augmentation strategy,face forgery,forgery contents,deepfake detection methods,vanilla binary classification problem,global feature,real images,fake images,fine-grained classification problem,multiattentional deepfake detection network,textural feature enhancement block,multiple spatial attention heads,regional independence loss
AI 理解论文
溯源树
样例
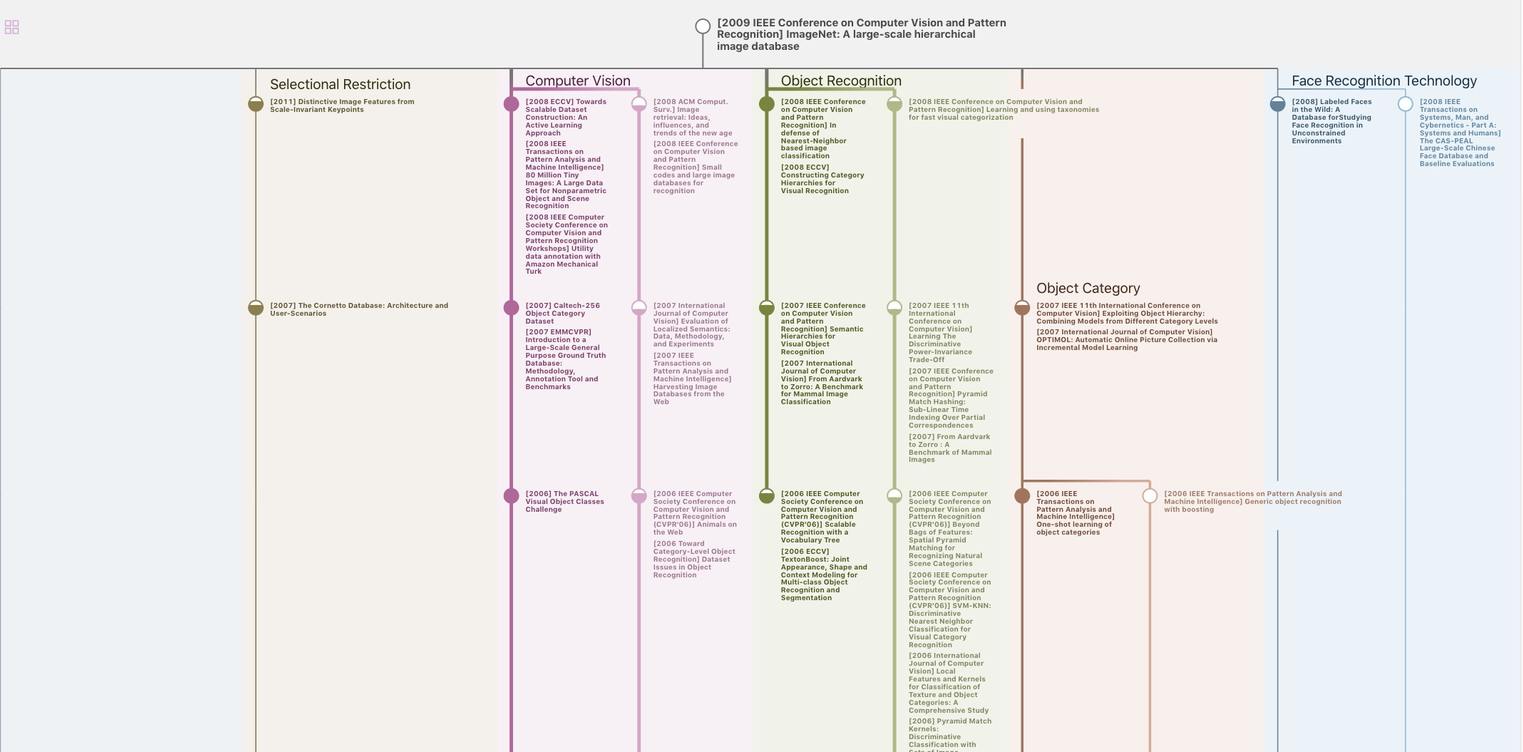
生成溯源树,研究论文发展脉络
Chat Paper
正在生成论文摘要