DAT-RNN - Trajectory Prediction with Diverse Attention.
ICMLA(2020)
摘要
Trajectory prediction, an emerging application of spatial-temporal graph, is extremely critical in dynamic applications such as autonomous vehicles and robots. However, the diversity of trajectories and the modeling of mutual relations make it difficult to predict trajectories precisely and efficiently. In this work, we propose a novel approach, diverse attention RNN (DAT-RNN), to handle the diversity of trajectories and the accurate modeling of neighboring relations with two novel and well-designed modules: DAT-RNN first uses a diversity-aware memory (DAM) module, which is based on the detour integral of each individual, to capture the temporal behavior of each person; then DAT-RNN employs an anomaly attention module (AAM), which integrates a weighted sum of spatial relations from multiple neighbors to assist the prediction. With the well-elaborated modules, DAT-RNN integrates both temporal and spatial relations to improve the prediction under various circumstances. Comprehensive experiments on ETH and UCY datasets demonstrate the efficacy of the proposed approach.
更多查看译文
关键词
Recurrent neural network,deep learning,spatial-temporal graph,trajectory prediction
AI 理解论文
溯源树
样例
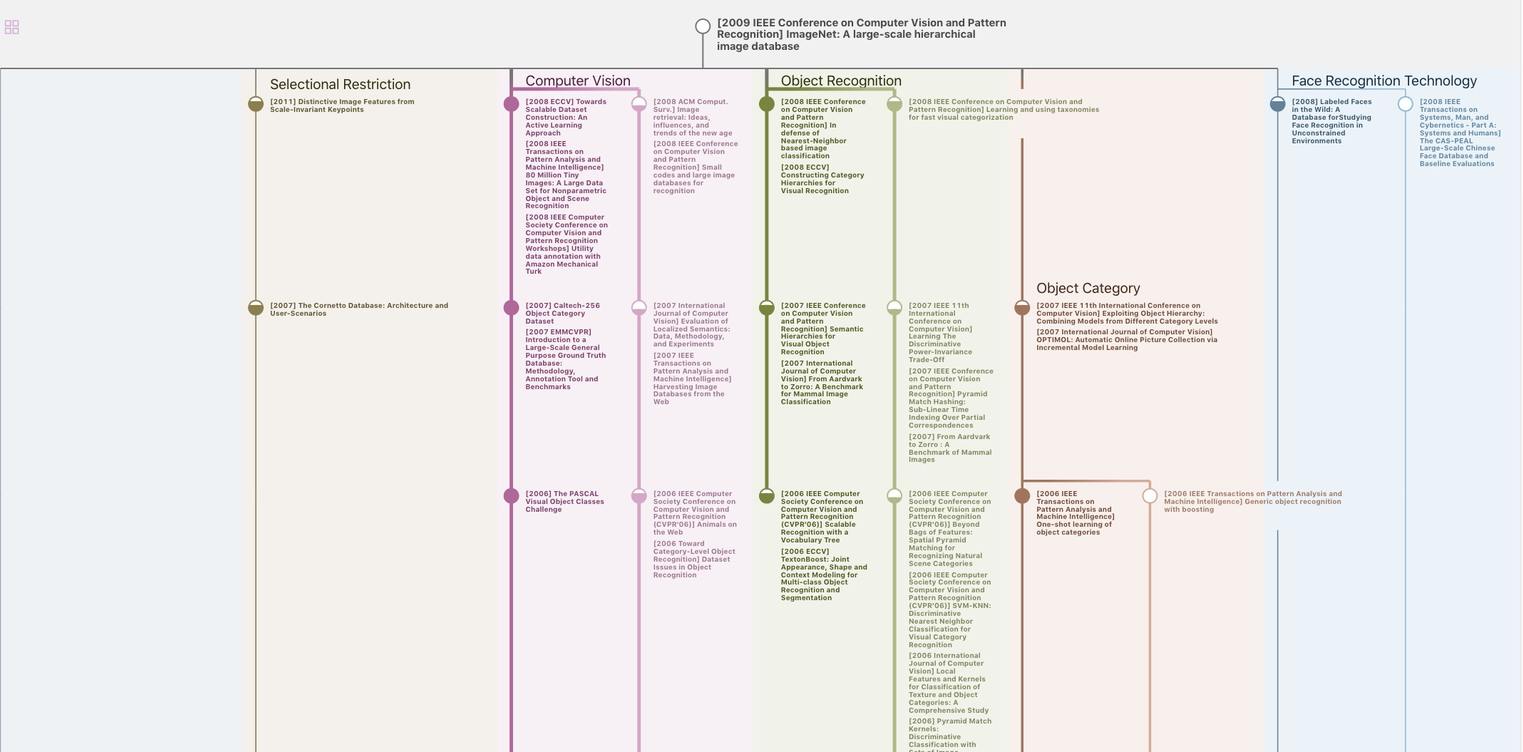
生成溯源树,研究论文发展脉络
Chat Paper
正在生成论文摘要