Estimating The Probability Density Function Of New Fabrics For Fabric Anomaly Detection
PROCEEDINGS OF THE 10TH INTERNATIONAL CONFERENCE ON PATTERN RECOGNITION APPLICATIONS AND METHODS (ICPRAM)(2021)
摘要
Image-based quality control aims at detecting anomalies (i.e. defects) in products. Supervised, data driven approaches have greatly improved Anomaly Detection (AD) performance, but suffer from a major drawback: they require large amounts of annotated training data, limiting their economic viability.In this work, we challenge and overcome this limitation for complex patterned fabrics. Investigating the structure of deep feature representations learned on a large-scale fabric dataset, we find that fabrics form clusters according to their fabric type, whereas anomalies form a cluster on their own. We leverage this clustering behavior to estimate the Probability Density Function (PDF) of new, previously unseen fabrics, in the deep feature representations directly. Using this approach, we outperform supervised and semi-supervised AD approaches trained on new fabrics, requiring only defect-free data for PDF-estimation.
更多查看译文
关键词
Anomaly Detection, Quality Control, Fabric Inspection, Transfer Learning, Probability Density Estimation
AI 理解论文
溯源树
样例
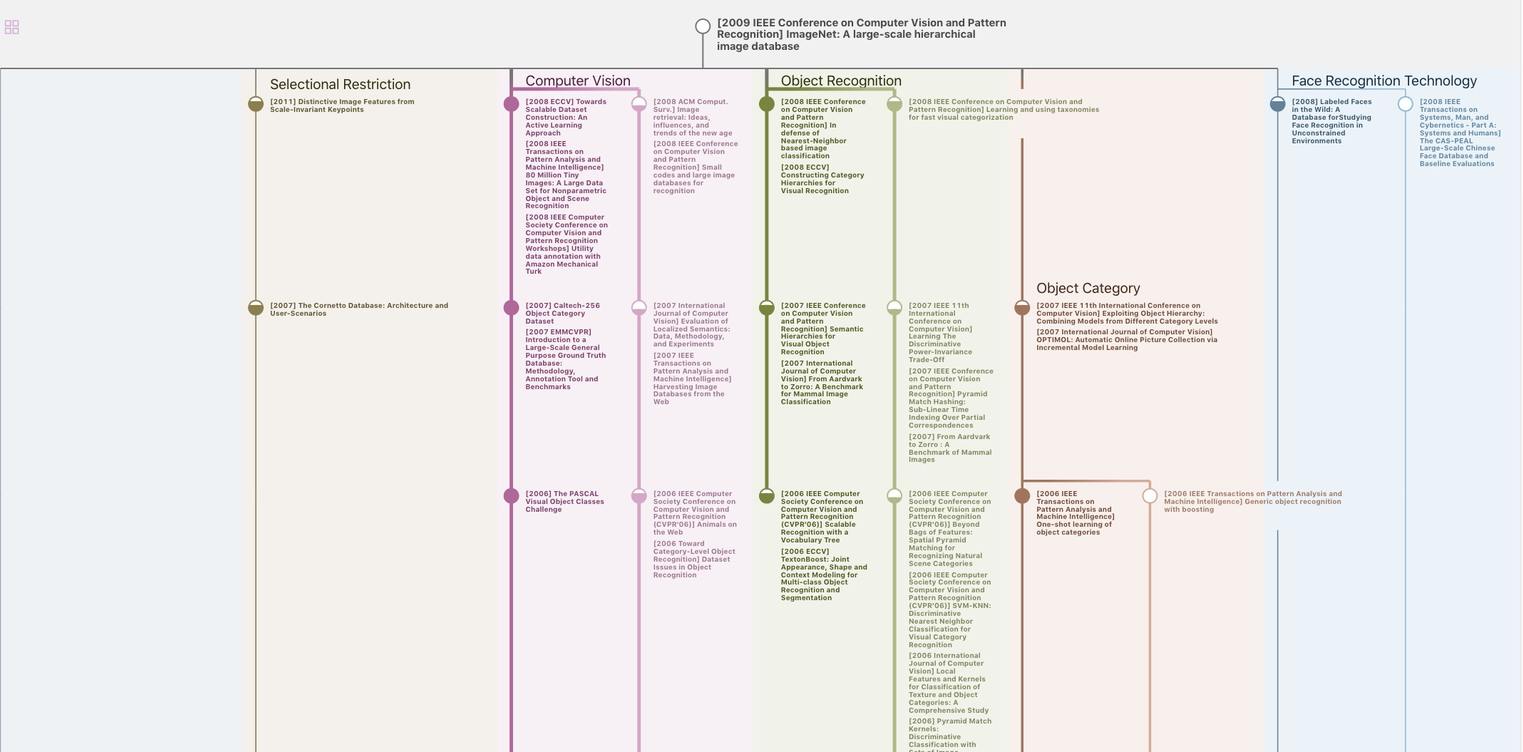
生成溯源树,研究论文发展脉络
Chat Paper
正在生成论文摘要