One For One, Or All For All: Equilibria And Optimality Of Collaboration In Federated Learning
INTERNATIONAL CONFERENCE ON MACHINE LEARNING, VOL 139(2021)
摘要
In recent years, federated learning has been embraced as an approach for bringing about collaboration across large populations of learning agents. However, little is known about how collaboration protocols should take agents' incentives into account when allocating individual resources for communal learning in order to maintain such collaborations. Inspired by game theoretic notions, this paper introduces a framework for incentive-aware learning and data sharing in federated learning. Our stable and envy-free equilibria capture notions of collaboration in the presence of agents interested in meeting their learning objectives while keeping their own sample collection burden low. For example, in an envy-free equilibrium, no agent would wish to swap their sampling burden with any other agent and in a stable equilibrium, no agent would wish to unilaterally reduce their sampling burden.In addition to formalizing this framework, our contributions include characterizing the structural properties of such equilibria, proving when they exist, and showing how they can be computed. Furthermore, we compare the sample complexity of incentive-aware collaboration with that of optimal collaboration when one ignores agents' incentives.
更多查看译文
关键词
collaboration,learning,optimality,equilibria
AI 理解论文
溯源树
样例
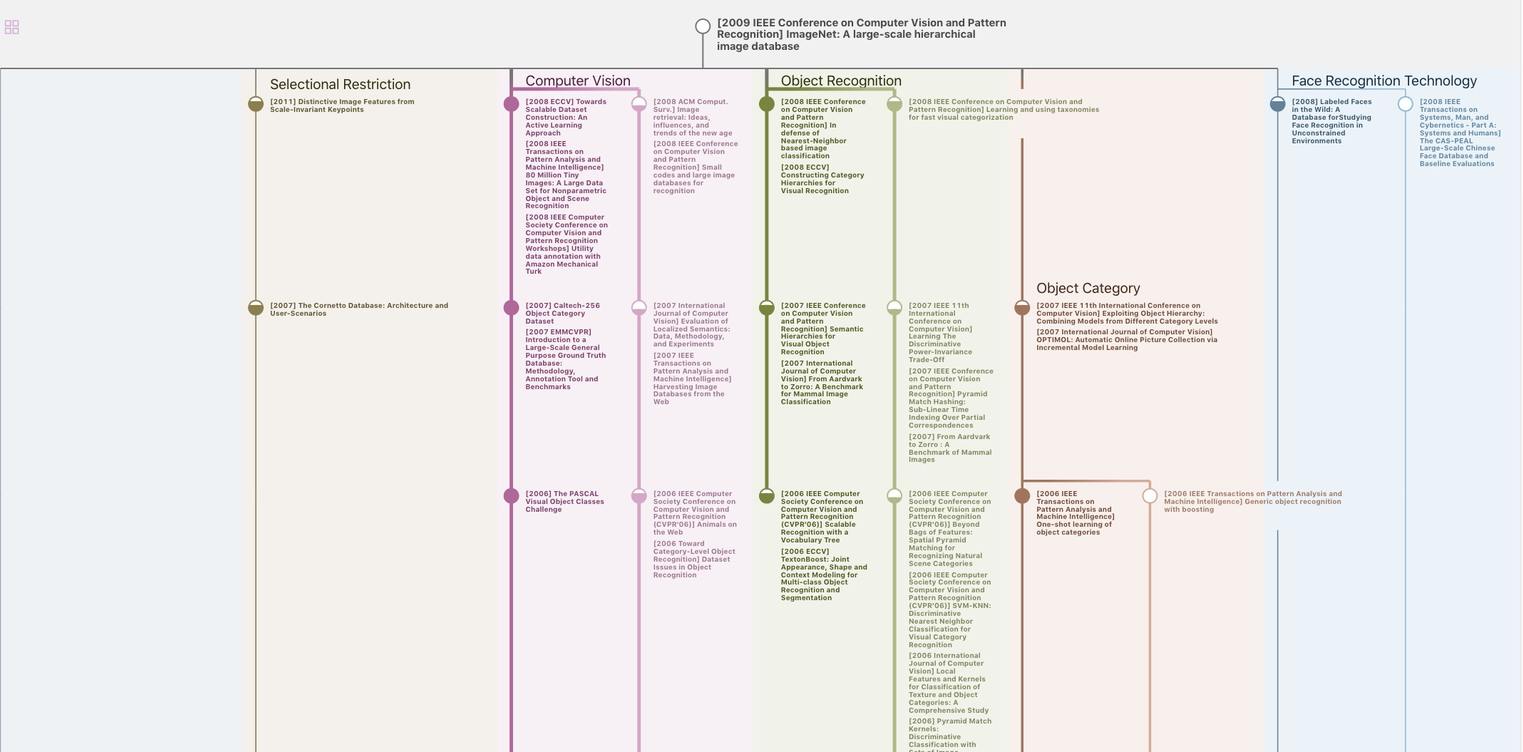
生成溯源树,研究论文发展脉络
Chat Paper
正在生成论文摘要