Probabilistic Seasonal Dengue Forecasting In Vietnam: A Modelling Study Using Superensembles
PLOS MEDICINE(2021)
摘要
BackgroundWith enough advanced notice, dengue outbreaks can be mitigated. As a climate-sensitive disease, environmental conditions and past patterns of dengue can be used to make predictions about future outbreak risk. These predictions improve public health planning and decision-making to ultimately reduce the burden of disease. Past approaches to dengue forecasting have used seasonal climate forecasts, but the predictive ability of a system using different lead times in a year-round prediction system has been seldom explored. Moreover, the transition from theoretical to operational systems integrated with disease control activities is rare.Methods and findingsWe introduce an operational seasonal dengue forecasting system for Vietnam where Earth observations, seasonal climate forecasts, and lagged dengue cases are used to drive a superensemble of probabilistic dengue models to predict dengue risk up to 6 months ahead. Bayesian spatiotemporal models were fit to 19 years (2002-2020) of dengue data at the province level across Vietnam. A superensemble of these models then makes probabilistic predictions of dengue incidence at various future time points aligned with key Vietnamese decision and planning deadlines. We demonstrate that the superensemble generates more accurate predictions of dengue incidence than the individual models it incorporates across a suite of time horizons and transmission settings. Using historical data, the superensemble made slightly more accurate predictions (continuous rank probability score [CRPS] = 66.8, 95% CI 60.6-148.0) than a baseline model which forecasts the same incidence rate every month (CRPS = 79.4, 95% CI 78.5-80.5) at lead times of 1 to 3 months, albeit with larger uncertainty. The outbreak detection capability of the superensemble was considerably larger (69%) than that of the baseline model (54.5%). Predictions were most accurate in southern Vietnam, an area that experiences semi-regular seasonal dengue transmission. The system also demonstrated added value across multiple areas compared to previous practice of not using a forecast. We use the system to make a prospective prediction for dengue incidence in Vietnam for the period May to October 2020. Prospective predictions made with the superensemble were slightly more accurate (CRPS = 110, 95% CI 102-575) than those made with the baseline model (CRPS = 125, 95% CI 120-168) but had larger uncertainty. Finally, we propose a framework for the evaluation of probabilistic predictions. Despite the demonstrated value of our forecasting system, the approach is limited by the consistency of the dengue case data, as well as the lack of publicly available, continuous, and long-term data sets on mosquito control efforts and serotype-specific case data.ConclusionsThis study shows that by combining detailed Earth observation data, seasonal climate forecasts, and state-of-the-art models, dengue outbreaks can be predicted across a broad range of settings, with enough lead time to meaningfully inform dengue control. While our system omits some important variables not currently available at a subnational scale, the majority of past outbreaks could be predicted up to 3 months ahead. Over the next 2 years, the system will be prospectively evaluated and, if successful, potentially extended to other areas and other climate-sensitive disease systems.Author summaryWhy was this study done?A climate-driven dengue early warning system would allow public health decision-makers to design, implement, and target timely interventions to the most at-risk places.Dengue is sensitive to changes in temperature, rainfall, humidity, and wind speed. Therefore, a dengue prediction model driven by seasonal climate forecasts offers a valuable tool for predicting dengue risk in advance.What did the researchers do and find?We developed a superensemble of probabilistic models to predict dengue incidence across Vietnam up to 6 months ahead. The predictive ability of the superensemble was assessed using multiple verification metrics and compared to a baseline model at multiple lead times, seasons, and locations.We found that the model superensemble generated more accurate predictions than the baseline model 1 to 3 months ahead but not 4 to 6 months ahead.The model superensemble, however, was better able to predict outbreaks than the baseline model across all lead times.The predictive ability of the model varied with geographic location, forecast horizon, and time of the year and performed best in the peak season in areas experiencing a high level of transmission.What do these findings mean?While outbreaks of infectious diseases are difficult to predict, particularly several months ahead, model superensembles, which combine multiple climatic drivers with dengue surveillance data at the time of forecast issue date, provide a useful decision-support tool.Early warning systems driven by seasonal climate forecasts could shift dengue control towards a more preventative approach, guiding bespoke and targeted public health interventions and a more efficient allocation of scarce resources.
更多查看译文
AI 理解论文
溯源树
样例
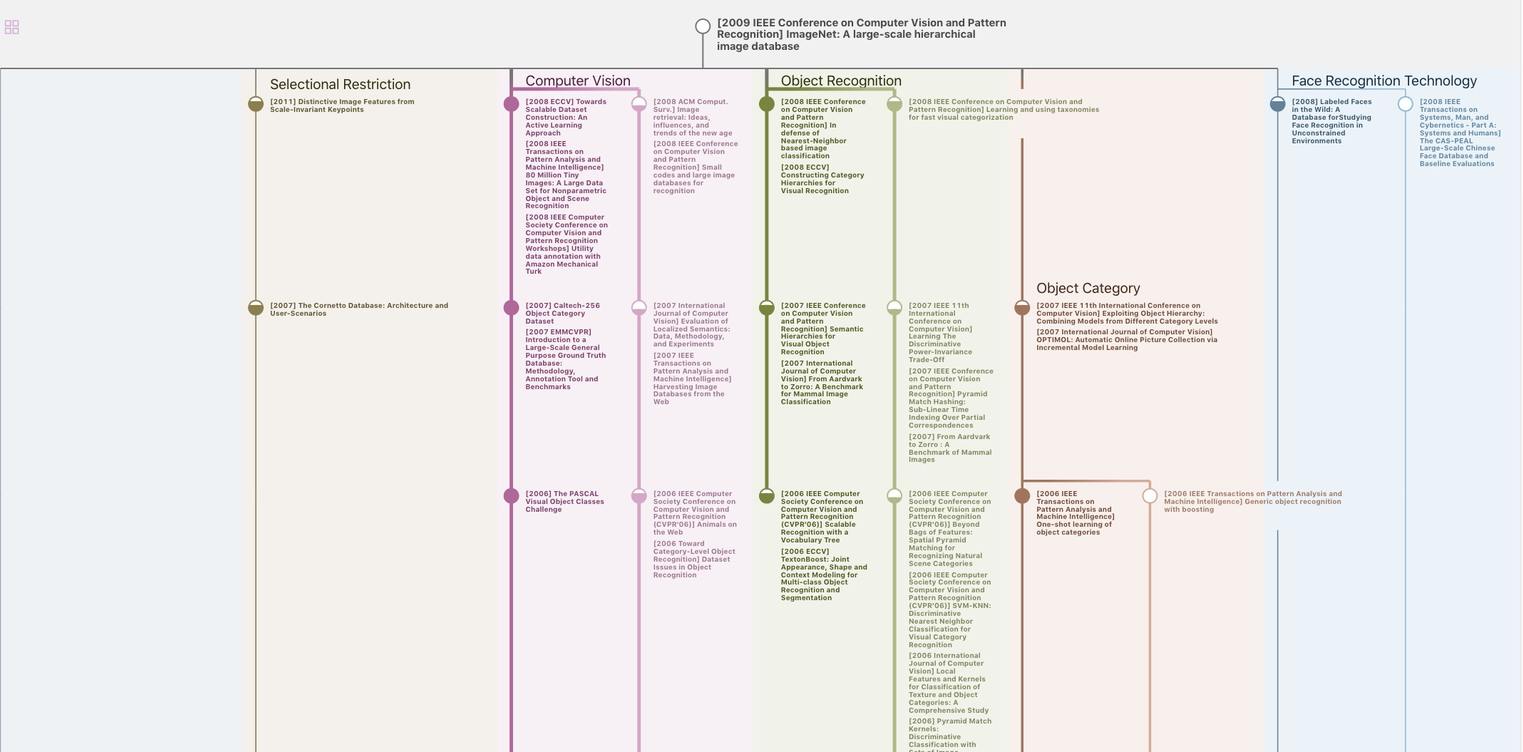
生成溯源树,研究论文发展脉络
Chat Paper
正在生成论文摘要