Lung Cancer and Granuloma Identification Using a Deep Learning Model to Extract 3-Dimensional Radiomics Features in CT Imaging
Clinical Lung Cancer(2021)
摘要
Surgeons and radiologists need a noninvasive approach to differentially diagnose and predict the probability of solitary solid pulmonary nodule malignancy before surgery. In this study, we developed and validated a deep learning model combining perinodular and intranodular radiomics and clinical features to preoperatively differentiate solitary granuloma nodules from solid lung cancer in patients with spiculation, lobulation, or pleural indentation on CT. Our findings suggest that the clinical image radiomics deep learning model is a noninvasive diagnostic tool to differentiate between granuloma nodules and solid lung cancer. Background: We aimed to evaluate a deep learning (DL) model combining perinodular and intranodular radiomics features and clinical features for preoperative differentiation of solitary granuloma nodules (GNs) from solid lung cancer nodules in patients with spiculation, lobulation, or pleural indentation on CT. Patients and Methods: We retrospectively recruited 915 patients with solitary solid pulmonary nodules and suspicious signs of malignancy. Data including clinical characteristics and subjective CT findings were obtained. A 3-dimensional U-Net-based DL model was used for tumor segmentation and extraction of 3-dimensional radiomics features. We used the Maximum Relevance and Minimum Redundancy (mRMR) algorithm and the eXtreme Gradient Boosting (XGBoost) algorithm to select the intranodular, perinodular, and gross nodular radiomics features. We propose a medical image DL (IDL) model, a clinical image DL (CIDL) model, a radiomics DL (RDL) model, and a clinical image radiomics DL (CIRDL) model to preoperatively differentiate GNs from solid lung cancer. Five-fold cross-validation was used to select and evaluate the models. The prediction performance of the models was evaluated using receiver operating characteristic and calibration curves. Results: The CIRDL model achieved the best performance in differentiating between GNs and solid lung cancer (area under the curve [AUC] = 0.9069), which was significantly higher compared with the IDL (AUC = 0.8322), CIDL (AUC = 0.8652), intraRDL (AUC = 0.8583), peri-RDL (AUC = 0.8259), and gross-RDL (AUC = 0.8705) models. Conclusion: The proposed CIRDL model is a noninvasive diagnostic tool to differentiate between granuloma nodules and solid lung cancer nodules and reduce the need for invasive diagnostic and surgical procedures. (C) 2021 Published by Elsevier Inc.
更多查看译文
关键词
Solitary pulmonary nodule,Convolutional neural network,Classification,Carcinoma,Computed tomography
AI 理解论文
溯源树
样例
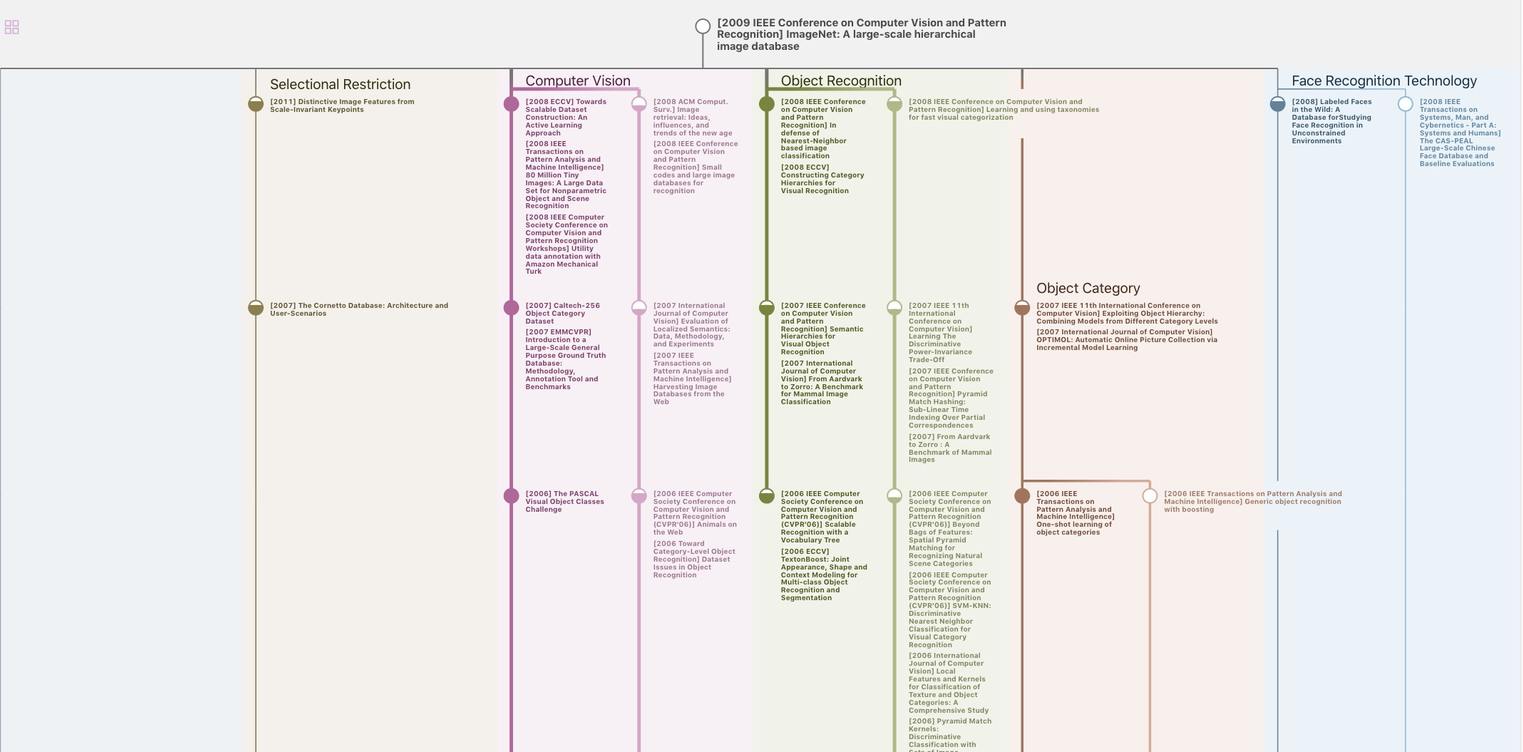
生成溯源树,研究论文发展脉络
Chat Paper
正在生成论文摘要