U-DuDoNet: Unpaired Dual-Domain Network for CT Metal Artifact Reduction
MEDICAL IMAGE COMPUTING AND COMPUTER ASSISTED INTERVENTION - MICCAI 2021, PT VI(2021)
摘要
Recently, both supervised and unsupervised deep learning methods have been widely applied on the CT metal artifact reduction (MAR) task. Supervised methods such as Dual Domain Network (Du-DoNet) work well on simulation data; however, their performance on clinical data is limited due to domain gap. Unsupervised methods are more generalized, but do not eliminate artifacts completely through the sole processing on the image domain. To combine the advantages of both MAR methods, we propose an unpaired dual-domain network (U-DuDoNet) trained using unpaired data. Unlike the artifact disentanglement network (ADN) that utilizes multiple encoders and decoders for disentangling content from artifact, our U-DuDoNet directly models the artifact generation process through additions in both sinogram and image domains, which is theoretically justified by an additive property associated with metal artifact. Our design includes a self-learned sinogram prior net, which provides guidance for restoring the information in the sinogram domain, and cyclic constraints for artifact reduction and addition on unpaired data. Extensive experiments on simulation data and clinical images demonstrate that our novel framework outperforms the state-of-the-art unpaired approaches.
更多查看译文
关键词
Metal artifact reduction, Dual-domain learning, Unpaired learning
AI 理解论文
溯源树
样例
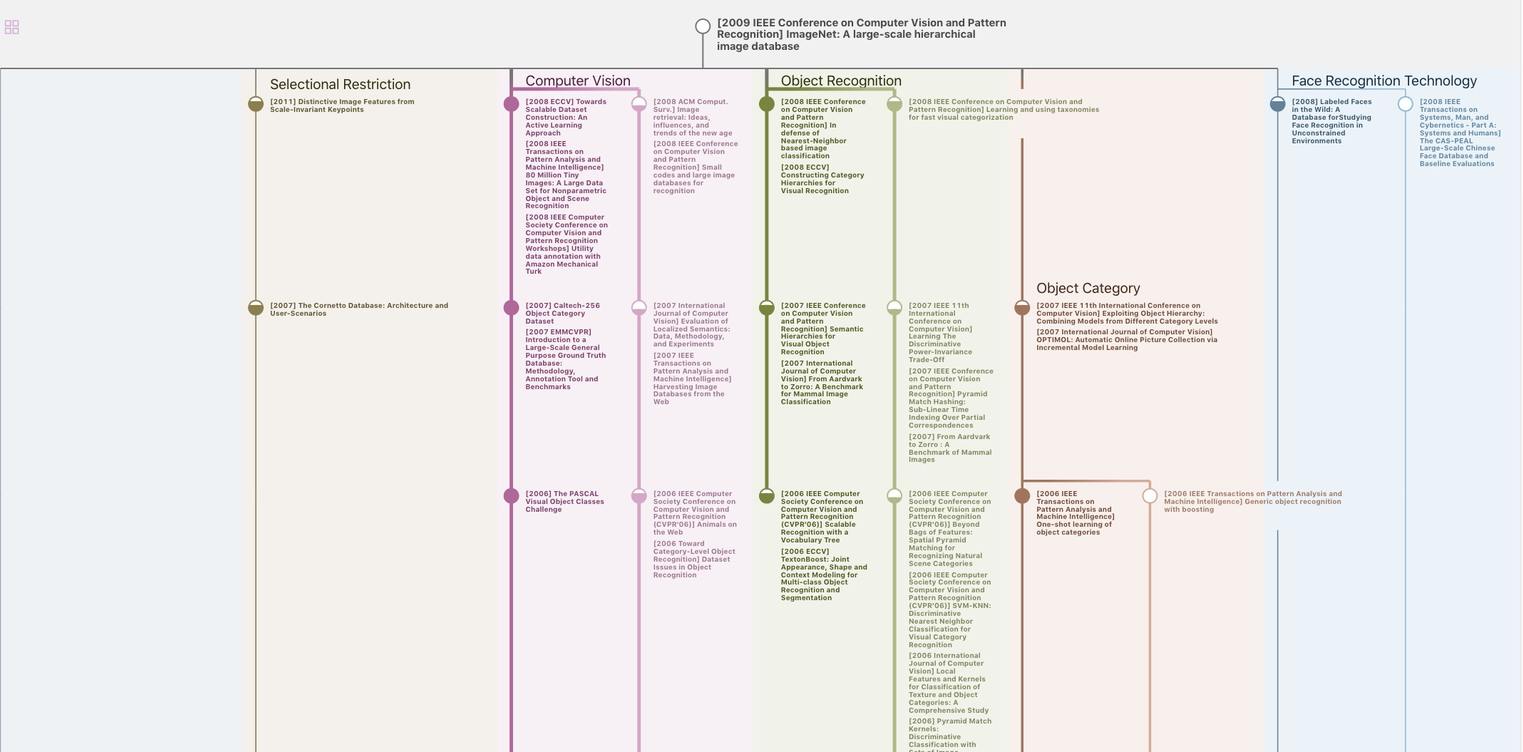
生成溯源树,研究论文发展脉络
Chat Paper
正在生成论文摘要