You only Learn Once: Universal Anatomical Landmark Detection
MEDICAL IMAGE COMPUTING AND COMPUTER ASSISTED INTERVENTION - MICCAI 2021, PT V(2021)
摘要
Detecting anatomical landmarks in medical images plays an essential role in understanding the anatomy and planning automated processing. In recent years, a variety of deep neural network methods have been developed to detect landmarks automatically. However, all of those methods are unary in the sense that a highly specialized network is trained for a single task say associated with a particular anatomical region. In this work, for the first time, we investigate the idea of "You Only Learn Once (YOLO)" and develop a universal anatomical landmark detection model to realize multiple landmark detection tasks with end-to-end training based on mixed datasets. The model consists of a local network and a global network: The local network is built upon the idea of universal U-Net to learn multi-domain local features and the global network is a parallelly-duplicated sequential of dilated convolutions that extract global features to further disambiguate the landmark locations. It is worth mentioning that the new model design requires much fewer parameters than models with standard convolutions to train. We evaluate our YOLO model on three X-ray datasets of 1,588 images on the head, hand, and chest, collectively contributing 62 landmarks. The experimental results show that our proposed universal model behaves largely better than any previous models trained on multiple datasets. It even beats the performance of the model that is trained separately for every single dataset. Our code is available at https://github.com/ICT-MIRACLE-lab/YOLO_Universal_Anatomical_Landmark_Detection.
更多查看译文
关键词
Landmark detection, Multi-domain learning
AI 理解论文
溯源树
样例
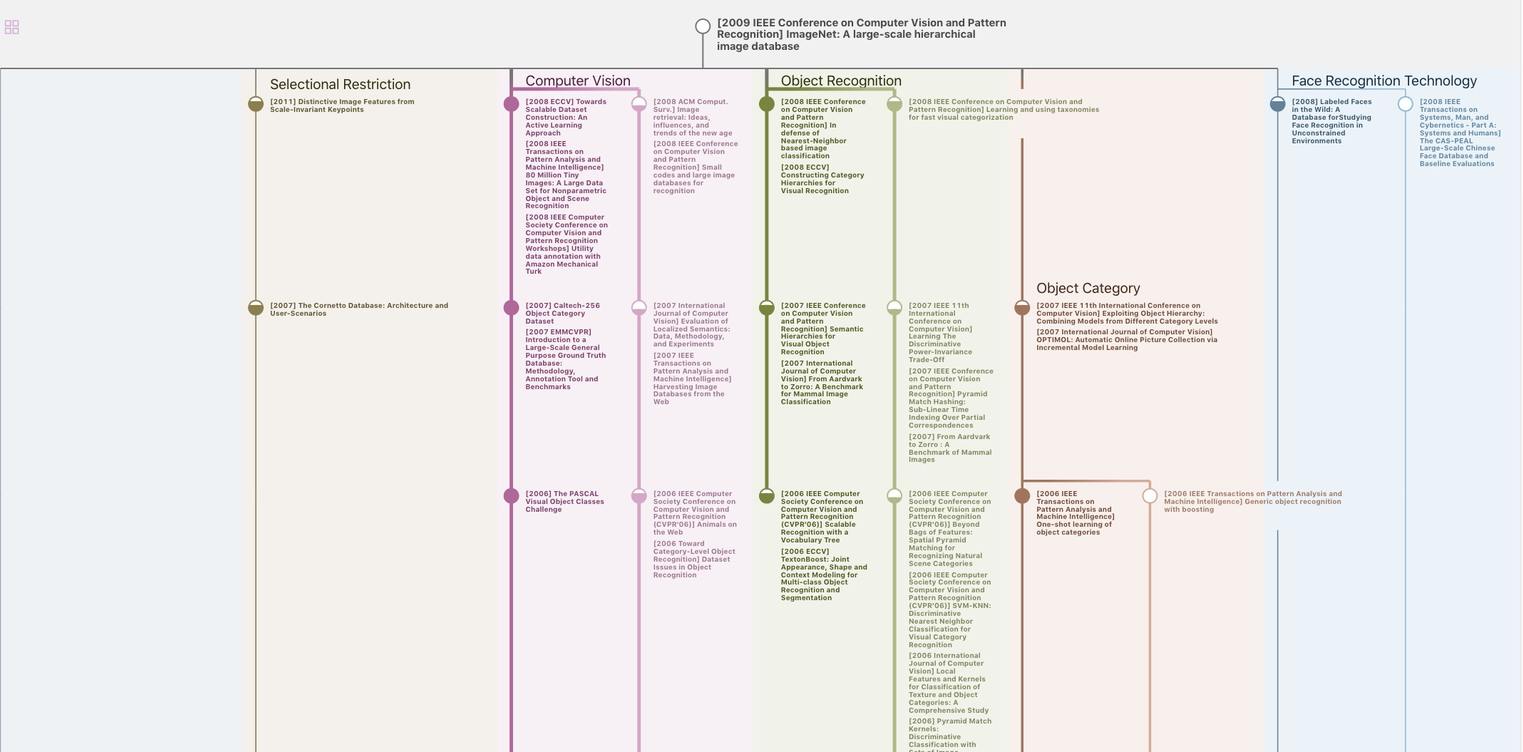
生成溯源树,研究论文发展脉络
Chat Paper
正在生成论文摘要