DISC : A Dynamic Shape Compiler for Machine LearningWorkloads
EUROSYS(2021)
摘要
Many recent machine learning models show dynamic shape characteristics. However, existing AI compiler optimization systems suffer a lot from problems brought by dynamic shape models, including compilation overhead, memory usage, optimization pipeline and deployment complexity. This paper provides a compiler system to natively support optimization for dynamic shape workloads, named DISC. DISC enriches a set of IR to form a fully dynamic shape representation. It generates the runtime flow at compile time to support processing dynamic shape based logic, which avoids the interpretation overhead at runtime and enlarges the opportunity of host-device co-optimization. It addresses the kernel fusion problem of dynamic shapes with shape propagation and constraints collecting methods. This is the first work to demonstrate how to build an end-to-end dynamic shape compiler based on MLIR infrastructure. Experiments show that DISC achieves up to 3.3x speedup than TensorFlow/PyTorch, and 1.8x than Nimble.
更多查看译文
关键词
dynamic shape compiler,machine learning
AI 理解论文
溯源树
样例
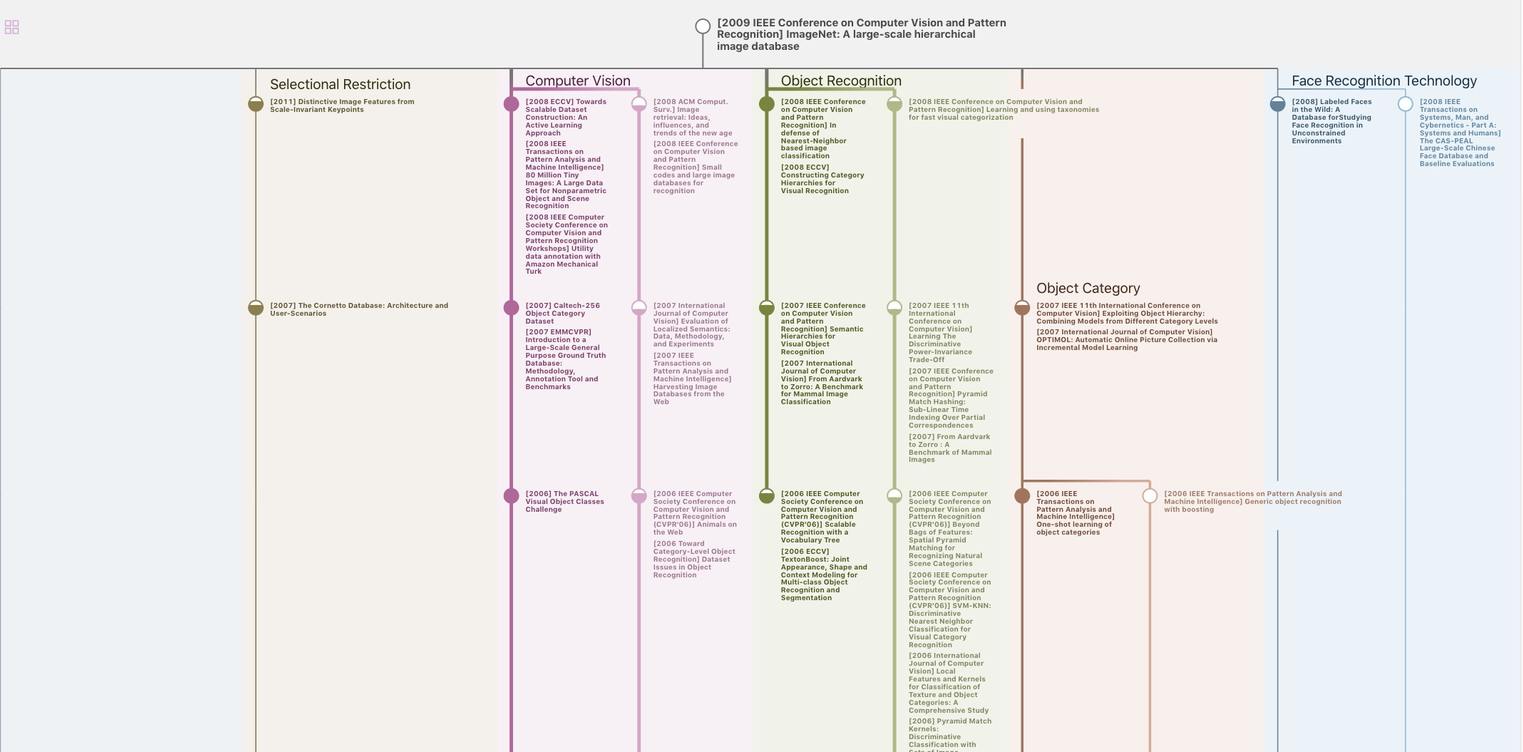
生成溯源树,研究论文发展脉络
Chat Paper
正在生成论文摘要