Multi-nuclide source term estimation method for severe nuclear accidents from sequential gamma dose rate based on a recurrent neural network
Journal of Hazardous Materials(2021)
摘要
When severe nuclear accidents at nuclear power plants release radioactive material into the atmosphere, the source term information is typically unknown. Estimating the emission rate of radionuclides is essential to assess the consequences of the accident before adequate decision-making can be performed. A recurrent neural network-based model, optimized with the Bayesian method, is proposed to estimate the emission rates of multi-nuclides using off-site sequential gamma dose rate monitoring data. Compared with the existing method that is based on least squares, this new model does not require a priori information and the complicated and time-consuming process of conducting atmospheric dispersion simulations following a nuclear accident, which is conducive to a faster response. Six typical radionuclides (Sr-91, La-140, Te-132, Xe-133, I-131, and Cs-137) were set as mixed source terms, combined with meteorological parameters, and input into the International Radiological Assessment System for simulation to generate data sets for model training. The results indicate that with the input of data describing the sequential gamma dose rate, the accuracy of the nuclide emission rates estimated by this new method is continuously improved, with a mean absolute percentage error for Te-132 of below 7% over 10 h.
更多查看译文
关键词
Nuclear emergency management,Source term inversion,Environmental monitoring data,InterRas,Bayesian optimization
AI 理解论文
溯源树
样例
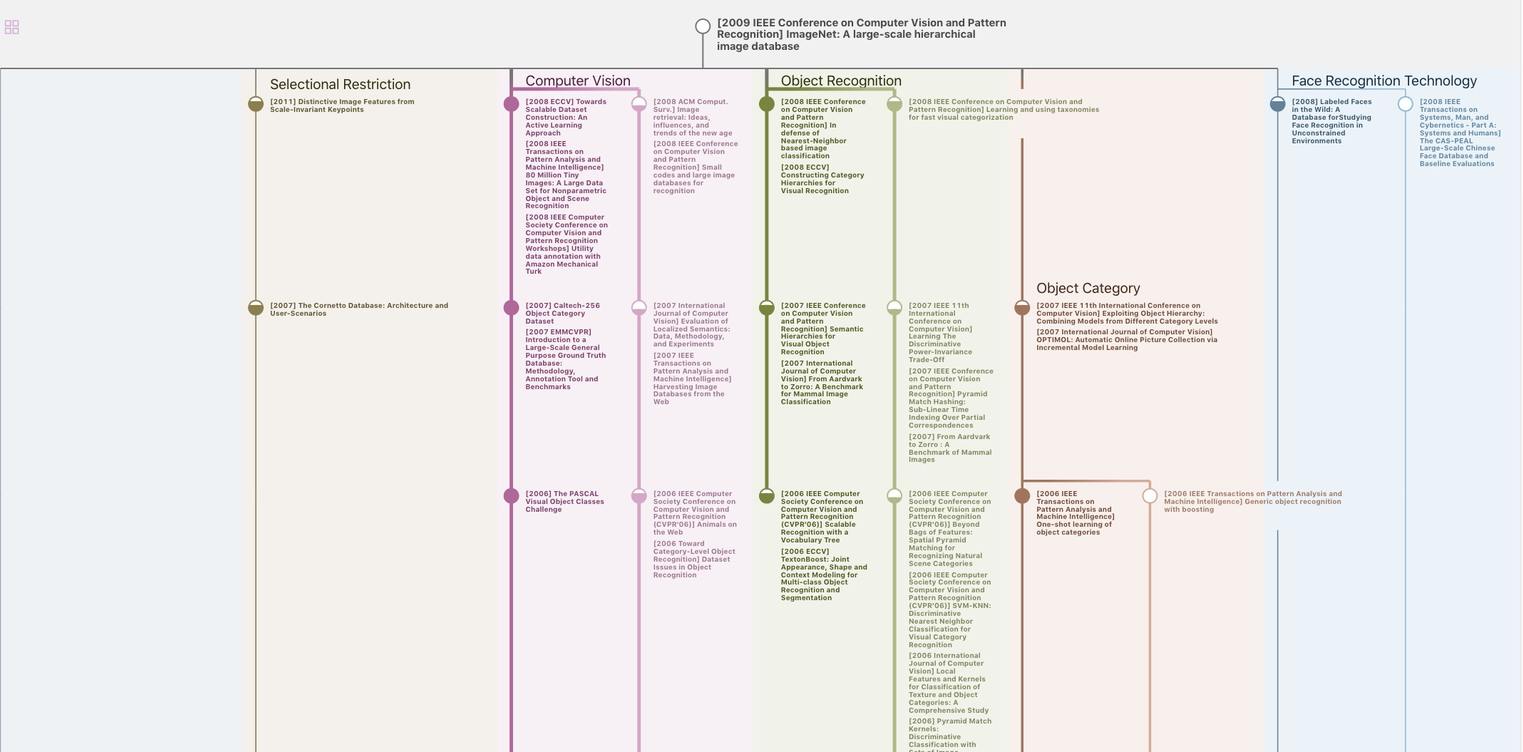
生成溯源树,研究论文发展脉络
Chat Paper
正在生成论文摘要