Self-Learning for Zero Shot Neural Machine Translation
CoRR(2021)
摘要
Neural Machine Translation (NMT) approaches employing monolingual data are showing steady improvements in resource rich conditions. However, evaluations using real-world low-resource languages still result in unsatisfactory performance. This work proposes a novel zero-shot NMT modeling approach that learns without the now-standard assumption of a pivot language sharing parallel data with the zero-shot source and target languages. Our approach is based on three stages: initialization from any pre-trained NMT model observing at least the target language, augmentation of source sides leveraging target monolingual data, and learning to optimize the initial model to the zero-shot pair, where the latter two constitute a self-learning cycle. Empirical findings involving four diverse (in terms of a language family, script and relatedness) zero-shot pairs show the effectiveness of our approach with up to +5.93 BLEU improvement against a supervised bilingual baseline. Compared to unsupervised NMT, consistent improvements are observed even in a domain-mismatch setting, attesting to the usability of our method.
更多查看译文
关键词
neural machine translation,shot,self-learning
AI 理解论文
溯源树
样例
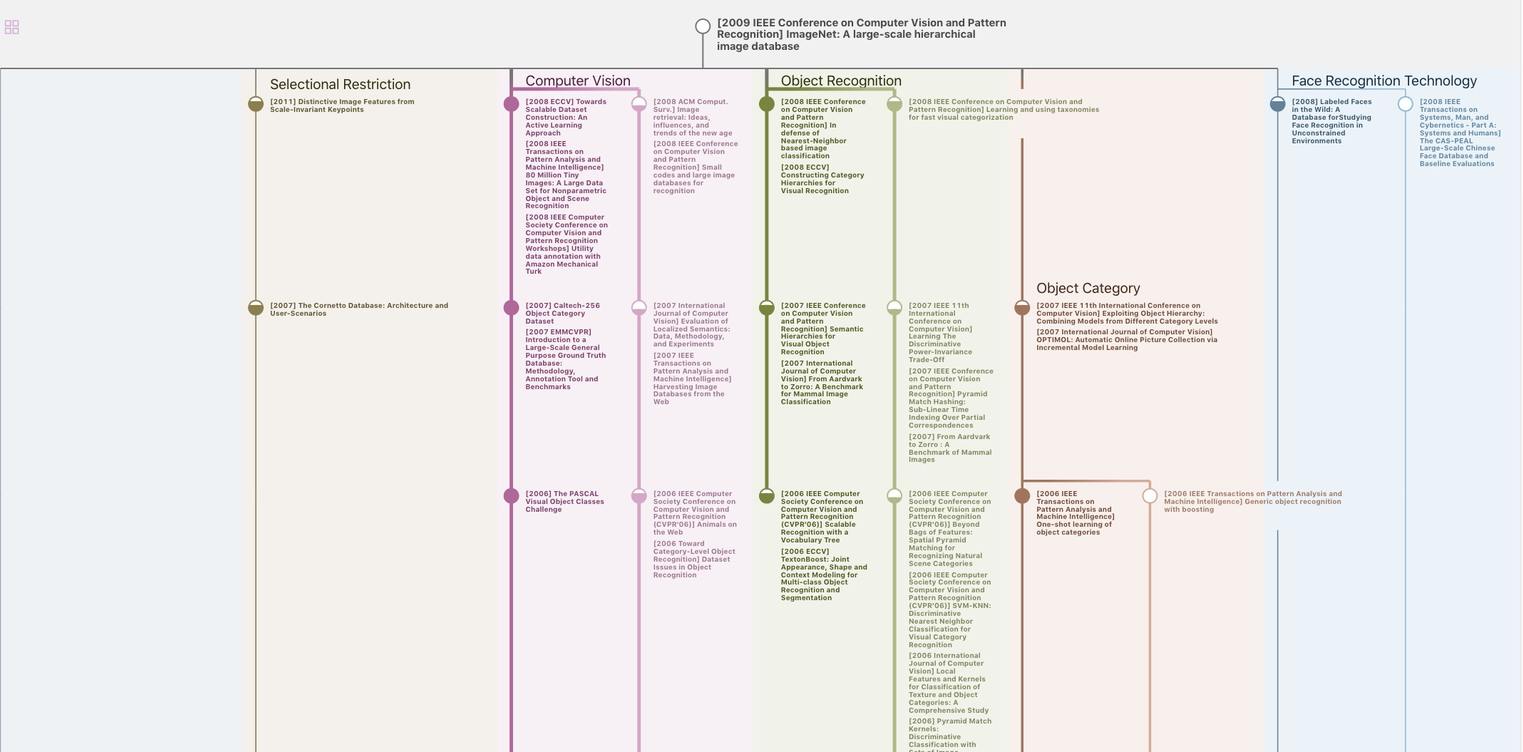
生成溯源树,研究论文发展脉络
Chat Paper
正在生成论文摘要