Bayesian sequential data assimilation for COVID-19 forecasting
Epidemics(2022)
摘要
We introduce a Bayesian sequential data assimilation and forecasting method for non-autonomous dynamical systems. We applied this method to the current COVID-19 pandemic. It is assumed that suitable transmission, epidemic and observation models are available and previously validated. The transmission and epidemic models are coded into a dynamical system. The observation model depends on the dynamical system state variables and parameters, and is cast as a likelihood function. The forecast is sequentially updated over a sliding window of epidemic records as new data becomes available. Prior distributions for the state variables at the new forecasting time are assembled using the dynamical system, calibrated for the previous forecast. Epidemic outbreaks are non-autonomous dynamical systems depending on human behavior, viral evolution and climate, among other factors, rendering it impossible to make reliable long-term epidemic forecasts. We show our forecasting method’s performance using a SEIR type model and COVID-19 data from several Mexican localities. Moreover, we derive further insights into the COVID-19 pandemic from our model predictions. The rationale of our approach is that sequential data assimilation is an adequate compromise between data fitting and dynamical system prediction.
更多查看译文
关键词
Bayesian inference,Data assimilation,COVID-19,SEIRD
AI 理解论文
溯源树
样例
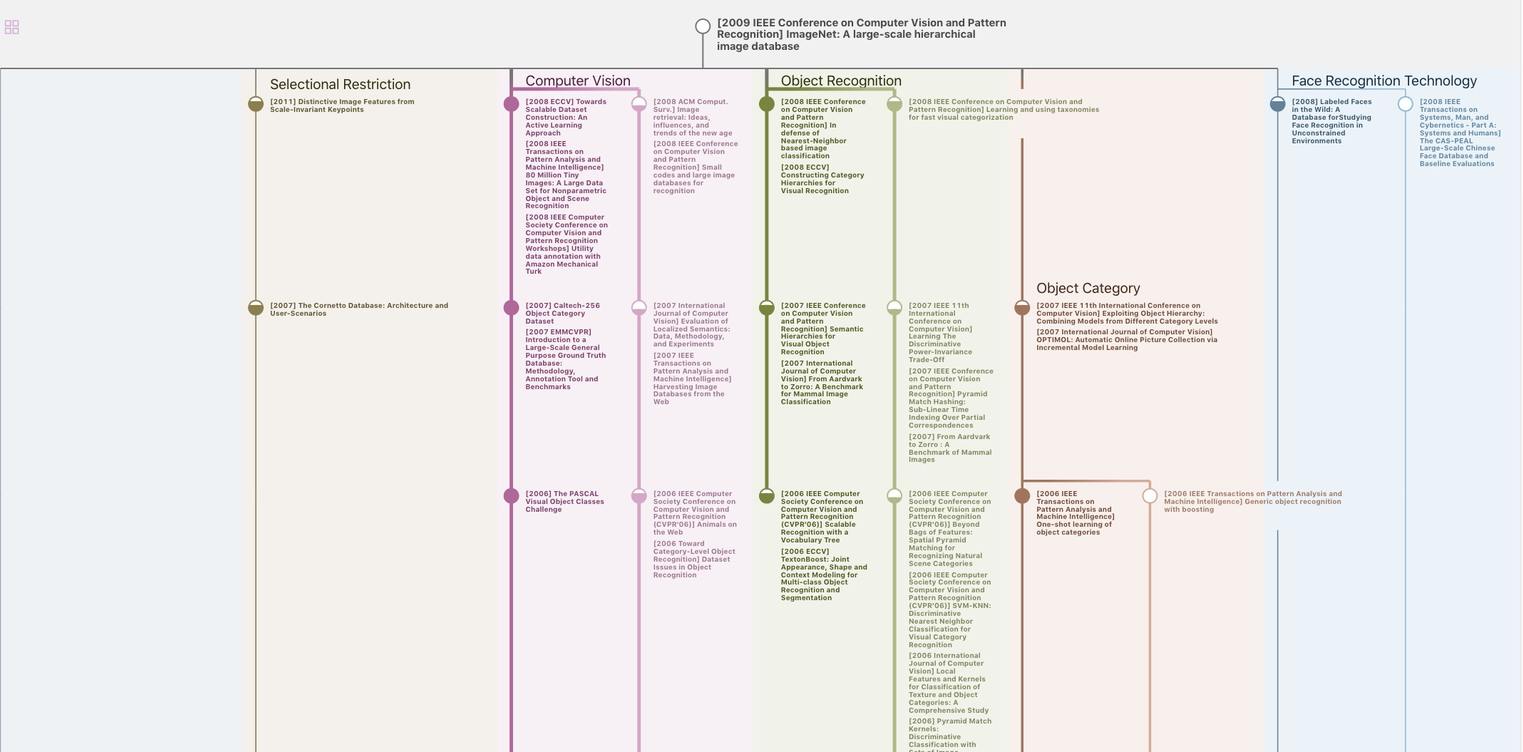
生成溯源树,研究论文发展脉络
Chat Paper
正在生成论文摘要