A Tree-based Federated Learning Approach for Personalized Treatment Effect Estimation from Heterogeneous Data Sources
arxiv(2021)
摘要
Federated learning is an appealing framework for analyzing sensitive data from distributed health data networks due to its protection of data privacy. Under this framework, data partners at local sites collaboratively build an analytical model under the orchestration of a coordinating site, while keeping the data decentralized. However, existing federated learning methods mainly assume data across sites are homogeneous samples of the global population, hence failing to properly account for the extra variability across sites in estimation and inference. Drawing on a multi-hospital electronic health records network, we develop an efficient and interpretable tree-based ensemble of personalized treatment effect estimators to join results across hospital sites, while actively modeling for the heterogeneity in data sources through site partitioning. The efficiency of our method is demonstrated by a study of causal effects of oxygen saturation on hospital mortality and backed up by comprehensive numerical results.
更多查看译文
关键词
personalized treatment effect estimation,federated learning approach,heterogeneous data sources,heterogeneous data,tree-based
AI 理解论文
溯源树
样例
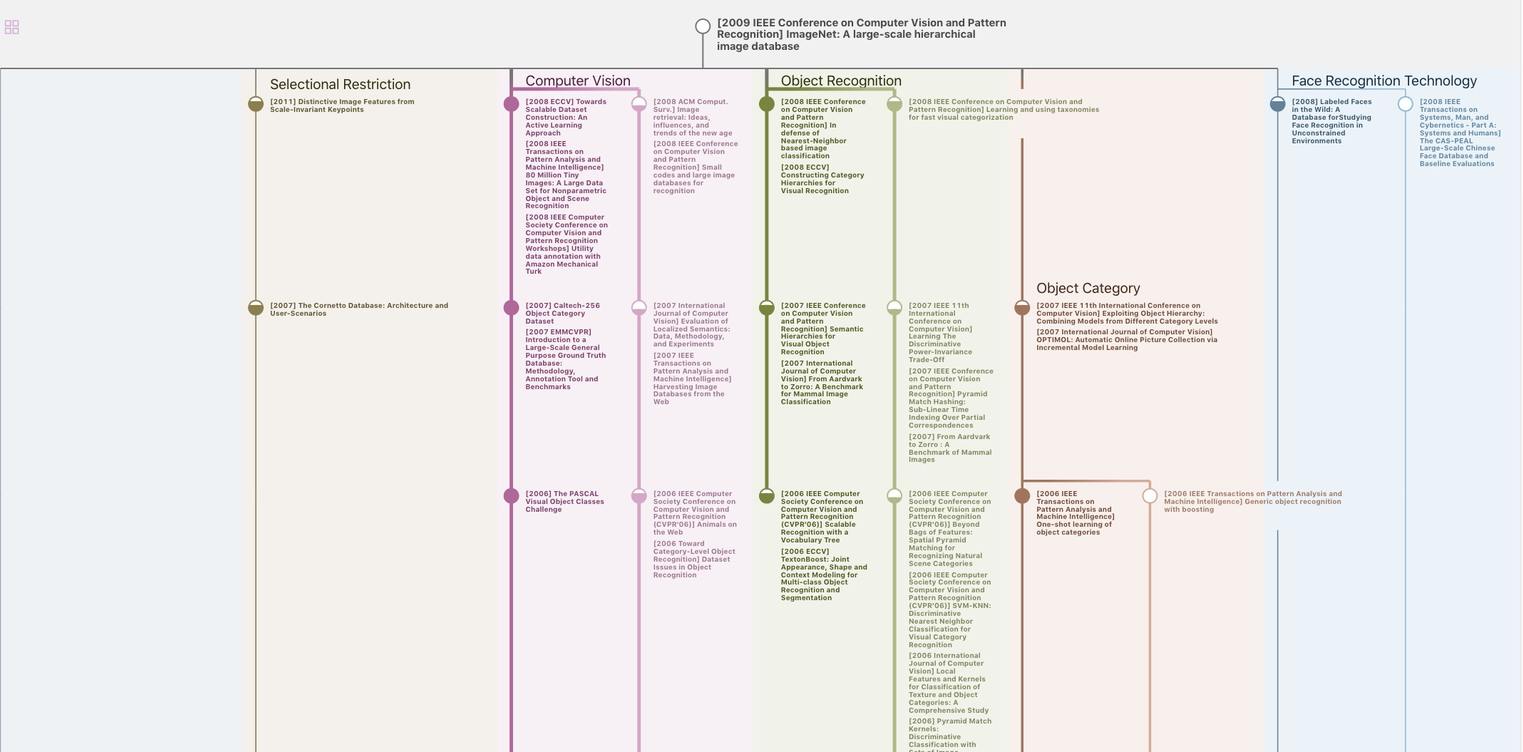
生成溯源树,研究论文发展脉络
Chat Paper
正在生成论文摘要