大数据下数据流聚类挖掘算法的优化分析
Internet of things technologies(2019)
AI Read Science
Must-Reading Tree
Example
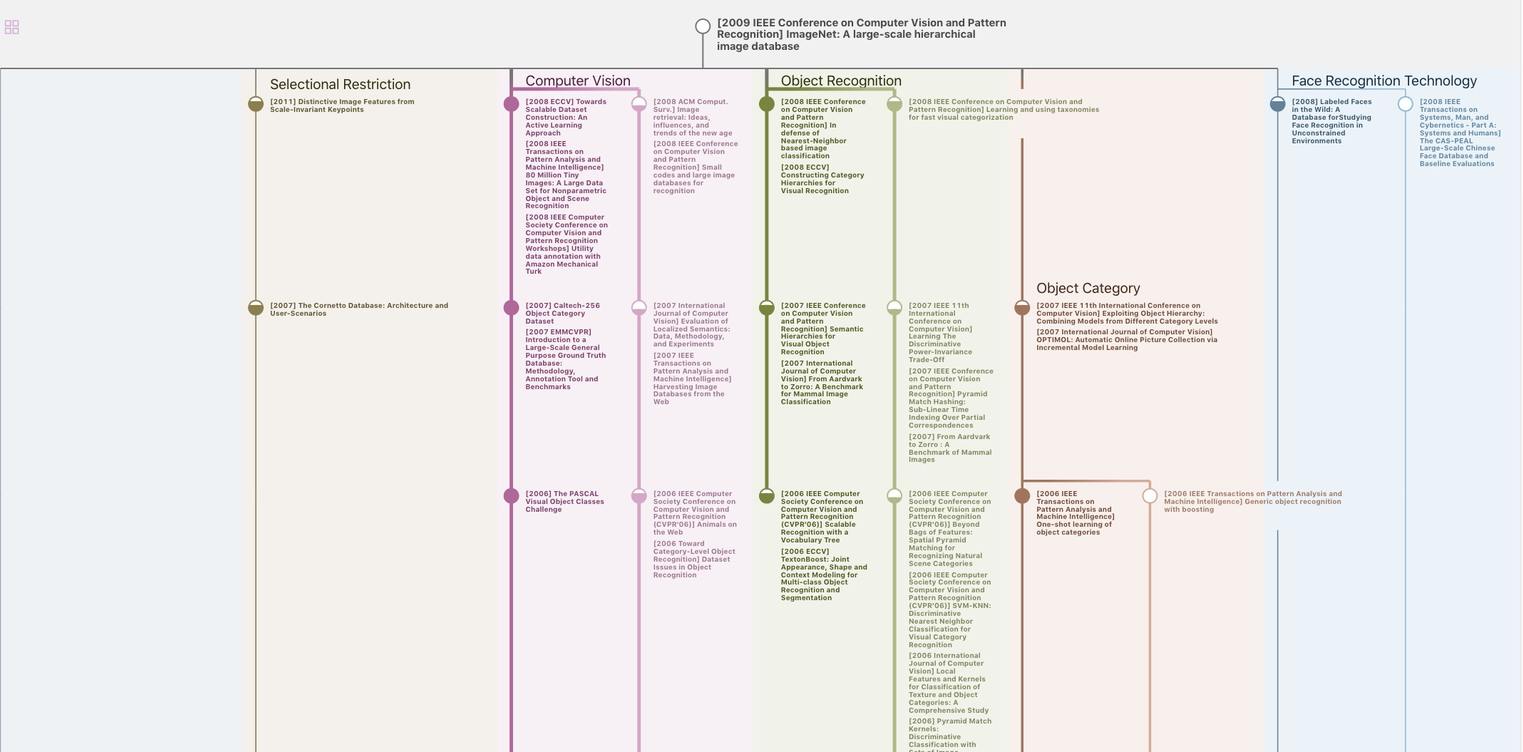
Generate MRT to find the research sequence of this paper
Chat Paper
Summary is being generated by the instructions you defined
Internet of things technologies(2019)