基于改进的期望值最大化自适应光学图像多帧迭代去卷积算法
Abstract
为提高自适应光学图像复原的效果,基于期望值最大化理论,提出了一种基于改进期望值最大化(EM)算法的自适应光学(AO)图像多帧联合去卷积算法.通过建立多帧AO图像退化的数学模型,推导出基于相位误差并随时间变化的点扩散函数(PSF)模型,根据图像功率谱密度及约束图像支持域的方法对AO图像进行去噪处理.应用A0成像系统参数与正则化技术相结合对EM算法进行改进,建立多帧AO图像联合去卷积的代价函数及其参数估计的优化模型.利用所建模型对模拟图像和实际观测的AO图像进行图像复原实验验证文中算法的复原效果.实验结果表明,与Wiener迭代盲去卷积、Richardson-Lucy迭代盲去卷积算法相比,文中算法迭代次数减少14.3%,估算精度有了明显提高,辨识出了AO图像的PSF,复原出了清晰的观测目标图像.研究结果对实际AO图像复原有一定的应用价值.
MorePDF
View via Publisher
AI Read Science
AI Summary
AI Summary is the key point extracted automatically understanding the full text of the paper, including the background, methods, results, conclusions, icons and other key content, so that you can get the outline of the paper at a glance.
Example
Background
Key content
Introduction
Methods
Results
Related work
Fund
Key content
- Pretraining has recently greatly promoted the development of natural language processing (NLP)
- We show that M6 outperforms the baselines in multimodal downstream tasks, and the large M6 with 10 parameters can reach a better performance
- We propose a method called M6 that is able to process information of multiple modalities and perform both single-modal and cross-modal understanding and generation
- The model is scaled to large model with 10 billion parameters with sophisticated deployment, and the 10 -parameter M6-large is the largest pretrained model in Chinese
- Experimental results show that our proposed M6 outperforms the baseline in a number of downstream tasks concerning both single modality and multiple modalities We will continue the pretraining of extremely large models by increasing data to explore the limit of its performance
Try using models to generate summary,it takes about 60s
Must-Reading Tree
Example
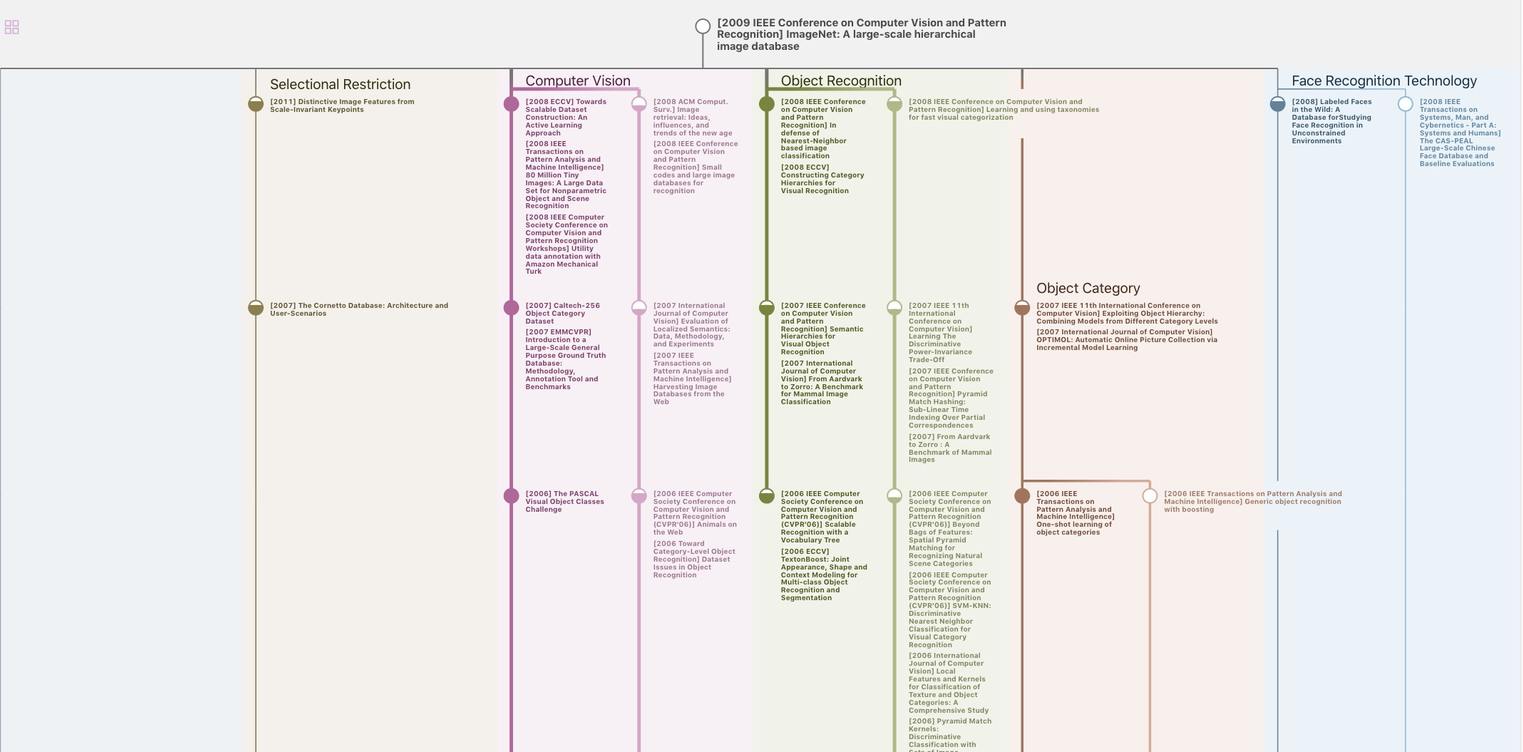
Generate MRT to find the research sequence of this paper
Related Papers
Chinese Journal of Liquid Crystals and Displays 2019
被引用8
LASER & OPTOELECTRONICS PROGRESS 2023
被引用0
Data Disclaimer
The page data are from open Internet sources, cooperative publishers and automatic analysis results through AI technology. We do not make any commitments and guarantees for the validity, accuracy, correctness, reliability, completeness and timeliness of the page data. If you have any questions, please contact us by email: report@aminer.cn
Chat Paper
GPU is busy, summary generation fails
Rerequest