SYNTHG: Mimicking RDF Graphs Using Tensor Factorization
2021 IEEE 15th International Conference on Semantic Computing (ICSC)(2021)
摘要
There is a need for synthetic graphs to help benchmarking efforts. Synthetic graphs that mimic real-world graphs can be used to avoid sending sensitive information to third parties while preserving topological characteristics of the input original graph. They can also be used to evaluate the scalability of different algorithms since the size of synthetic graphs can be scaled. In view of these applications, we introduce a novel approach to mimik RDF graphs. Our approach introduces a random rotation in the tensor factorization of the input RDF graph. By combining this matrix with the core tensor computed by the factorization, our approach can generate a graph which maintains the querying characteristics of the input graph, while not permitting a reconstruction of the input graph. We use Semantic Web Dog Food and DBpedia 2016 to evaluate our approach and compare the original, reconstructed and synthetic graphs by using them to benchmark five triple stores. The results show that the Pearson correlation between the performance of the triple stores under original and synthetic graphs is 0.91, 0.64 for Semantic Web Dog Food and DBpedia respectively. Our results also suggest that the synthetic graphs inherit the main graph characteristics of the original graphs. SynthG is open-source and is available at: https://github.com/dice-group/SynthG.
更多查看译文
关键词
mimicking RDF graphs,tensor factorization,synthetic graphs,real-world graphs,input original graph,input RDF graph,input graph,original graphs,reconstructed graphs,main graph characteristics
AI 理解论文
溯源树
样例
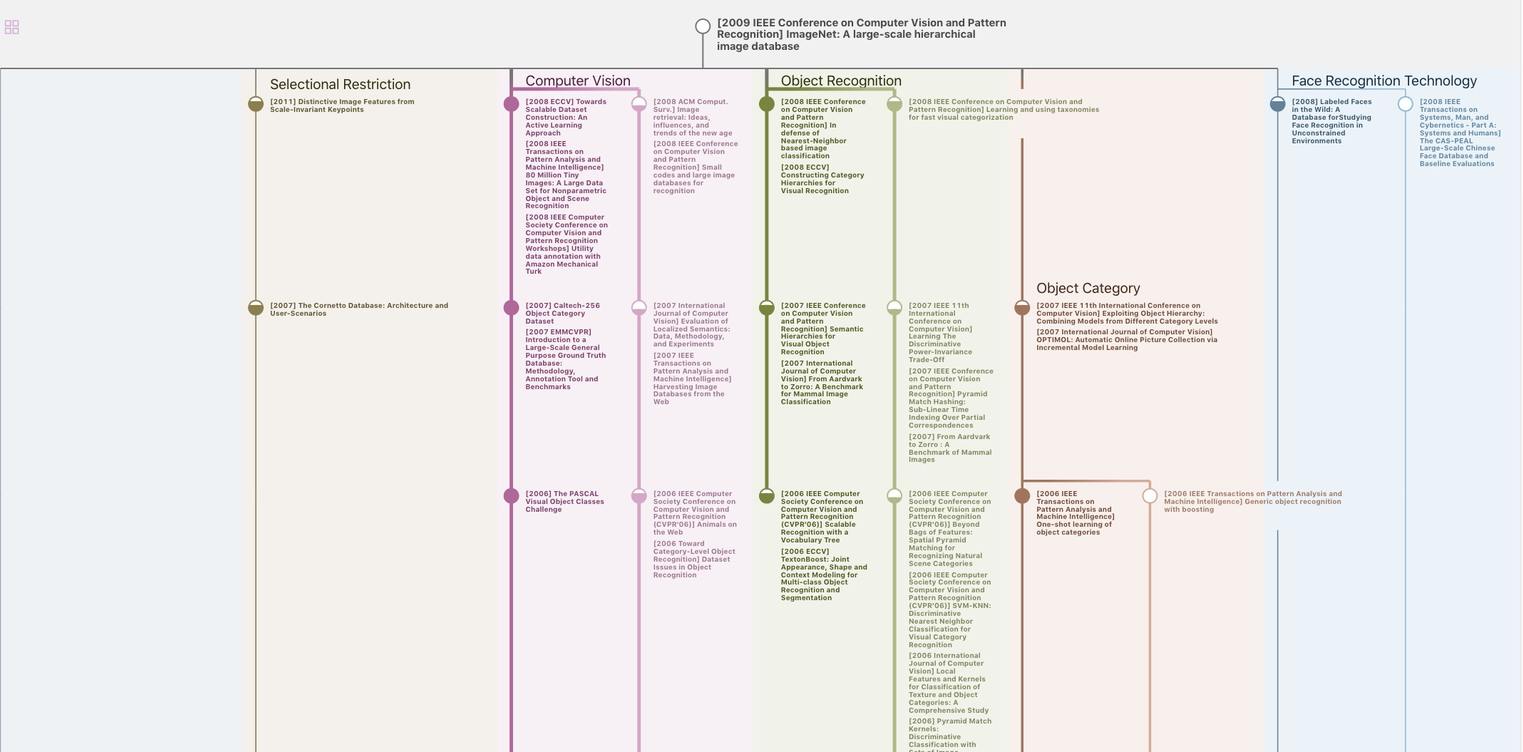
生成溯源树,研究论文发展脉络
Chat Paper
正在生成论文摘要