Scale-Residual Learning Network for Scene Text Detection
IEEE transactions on circuits and systems for video technology(2021)
Abstract
Detecting incidentally captured text in the wild remains an open problem due to challenging factors including unconstrained scenarios and large scale variation. In this paper, we establish a large-scale scene text detection dataset (LS-Text), containing 36, 000 images and 270, 783 text instances with various scales and complex scenarios, to promote the research of text detection. We propose a Scale-residual Learning Network (SLN) to deal with the scale variation problem in a progressive optimization manner. Specifically, we integrate both learnable feature concatenation and feature up-sampling operator. It can effectively eliminate the residuals between the outputs of SLN and ground-truth text instances by processing both the Feature Fusion Residuals (FFR) and the Scale Transformation Residuals (STR), simultaneously. By stacking multi-scale feature maps in a deep-to-shallow manner, SLN continuously optimizes feature representation by accumulating strong semantic information and rich texture details in a scale-residual learning way. Extensive experimental results on five challenging datasets demonstrate the state-of-the-art performance of the proposed SLN model, and the challenging aspects related to real-world scenarios of the proposed LS-Text dataset. Both the source code of SLN and the LS-Text dataset are available at https://github.com/SLN-Text-Detection.
MoreTranslated text
Key words
Feature extraction,Cameras,Image segmentation,Benchmark testing,Stacking,Task analysis,Transforms,Text detection,scale-residual learning,LS-Text dataset
AI Read Science
Must-Reading Tree
Example
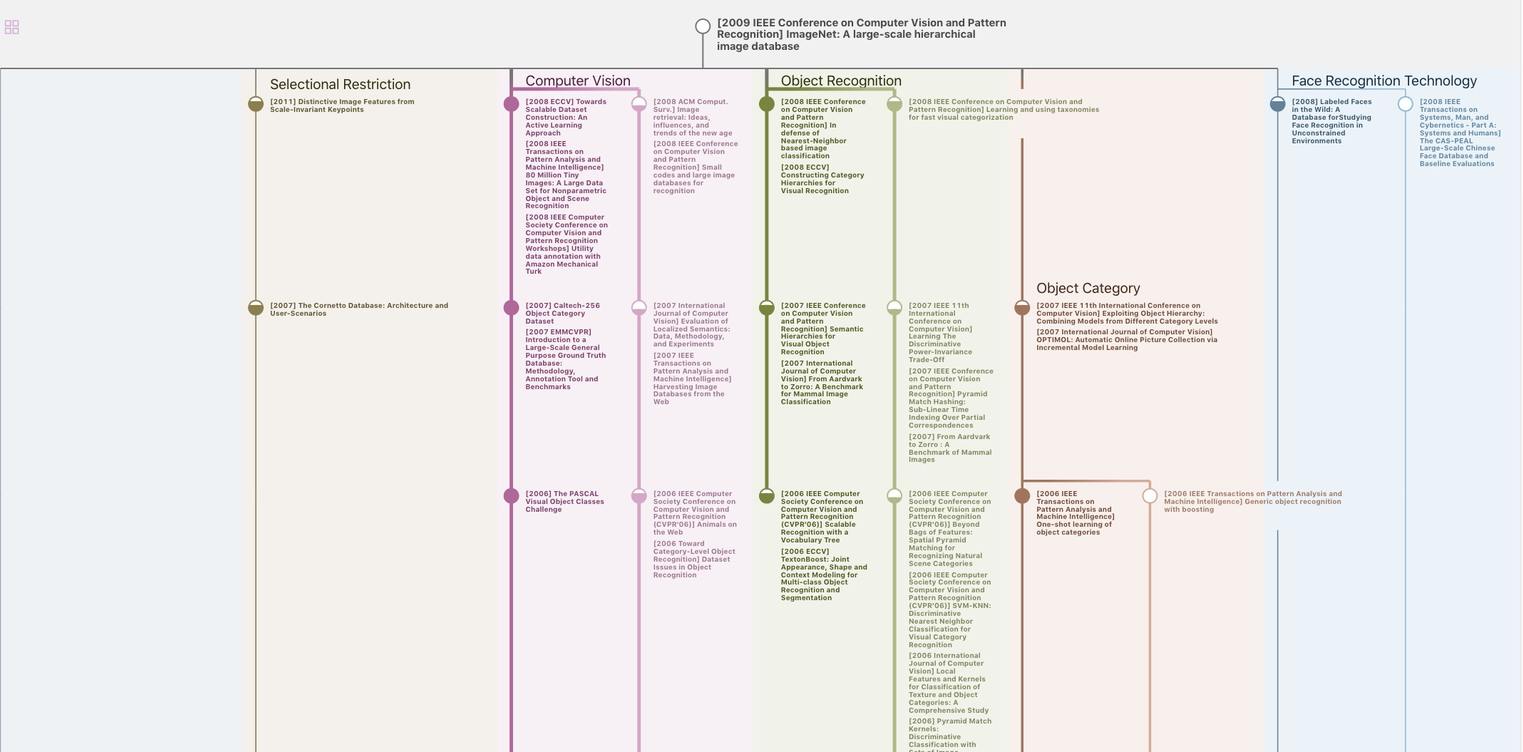
Generate MRT to find the research sequence of this paper
Chat Paper
Summary is being generated by the instructions you defined