System Reliability Analyses Of Slopes Based On Active-Learning Radial Basis Function
Rock and Soil Mechanics(2020)
摘要
The strength reduction method (SRM) has many advantages compared with the limit equilibrium method (LEM) in computing the safety factor of slopes, but the high computational cost, to some extent, limits the application of SRM in system reliability analyses of slopes. To effectively reduce the number of numerical analyses required for reliability analyses to alleviate the computation work when employing SRM, an efficient analysis method based on active-learning radial basis function (ARBF) surrogate model is introduced. This model uses the active-learning function to select trained samples near the limit state surface to update the surrogate model, which accelerates the convergence speed of the training process. With the linear kernel-based radial basis function, the optimization procedure of model parameters is simplified, by which a concise and stable surrogate model can be established. Moreover, an initial sampling strategy considering the characteristics of soil slopes is proposed to fully take advantage of active-learning process. Once a stable surrogate model is established, Monte Carlo simulation (MCS) is used to calculate the probability of system failure. As a comparison, two conventional reliability methods: active-learning Kriging (AK) model and the quadratic response surface method (QRMS), together with two typical soil slope cases, are tested to illustrate the computational efficiency and model stability of the introduced ARBF.
更多查看译文
关键词
slope, system reliability, shear strength reduction method, active-learning surrogate models, radial basis function
AI 理解论文
溯源树
样例
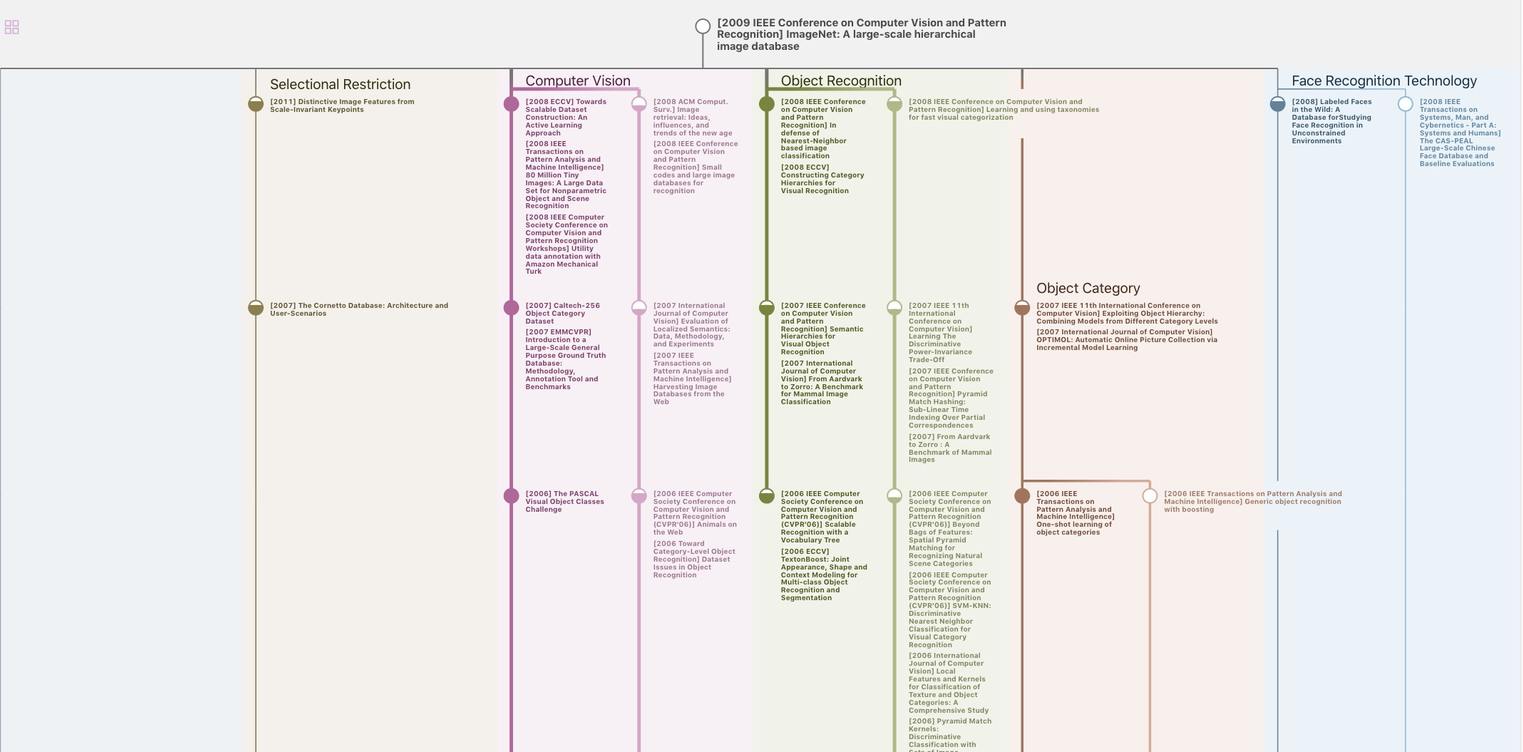
生成溯源树,研究论文发展脉络
Chat Paper
正在生成论文摘要