Improvement of Semantic Segmentation with DC-GAN in Autonomous driving
2020 28TH IRANIAN CONFERENCE ON ELECTRICAL ENGINEERING (ICEE)(2020)
摘要
Semantic segmentation is a long-standing challenge in computer vision. In the recent years, Generative Adversarial Network (GAN), has been turned to useful and common approach in data generation which can be used in deep learning problems. Adversarial training has been shown to produce efficient outcomes for generative image architecture. In current research, a generative adversarial framework is joint with a pre-trained deep semantic segmentation network as a post-processing unit to achieve better quality in the prediction masks. In the GAN, we attempt to culminate to minimum the loss between produced masks and ground truth masks. Our motivation is to generate masks which have the same distribution with ground truth yet more precise than output of semantic segmentation network. Hence, GAN is behaved as a sort of post-processing method for the semantic segmentation as a tricky problem to gain more high-quality throughput. Many of experiences are carried out on two open-source datasets, which include autonomous driving samples, show the efficiency of the recommended model. Our experiences demonstrate that our adversarial method propel to enhanced accuracy on the PASCAL VOC 2012 and Cityscape datasets.
更多查看译文
关键词
GAN,semantic segmentation,Autonomous driving,DC-GAN
AI 理解论文
溯源树
样例
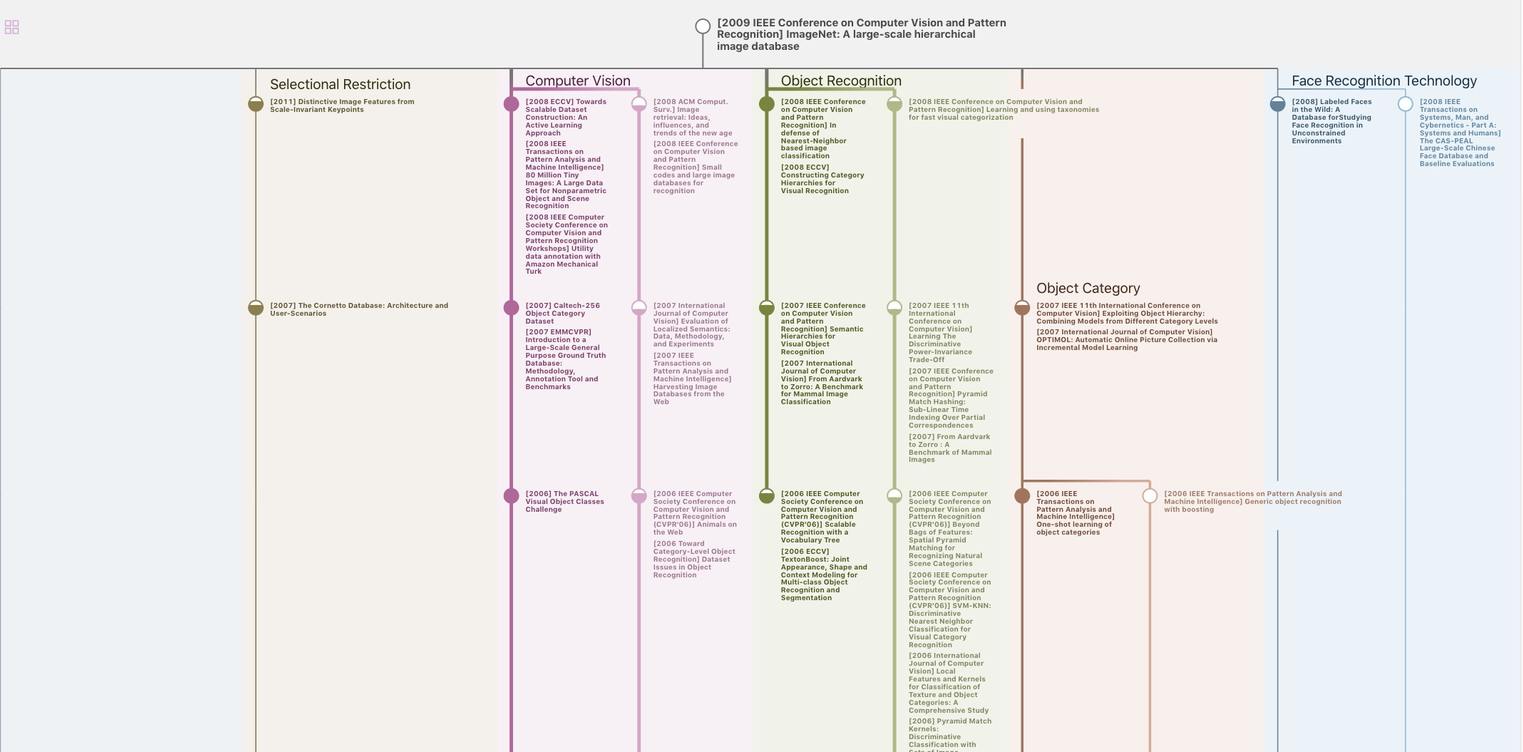
生成溯源树,研究论文发展脉络
Chat Paper
正在生成论文摘要