Exploiting Structured Data For Learning Contagious Diseases Under Incomplete Testing
INTERNATIONAL CONFERENCE ON MACHINE LEARNING, VOL 139(2021)
摘要
One of the ways that machine learning algorithms can help control the spread of an infectious disease is by building models that predict who is likely to become infected making them good candidates for preemptive interventions. In this work we ask: can we build reliable infection prediction models when the observed data is collected under limited, and biased testing that prioritizes testing symptomatic individuals? Our analysis suggests that when the infection is highly transmissible, incomplete testing might be sufficient to achieve good out-of-sample prediction error. Guided by this insight, we develop an algorithm that predicts infections, and show that it outperforms baselines on simulated data. We apply our model to data from a large hospital to predict Clostridioides difficile infections; a communicable disease that is characterized by both symptomatically infected and asymptomatic (i.e., untested) carriers. Using a proxy instead of the unobserved untested-infected state, we show that our model outperforms benchmarks in predicting infections.
更多查看译文
关键词
Communicable disease,Infectious disease (medical specialty),Data model,Predictive modelling,Artificial neural network,Ask price,Machine learning,Computer science,Artificial intelligence,Clostridioides,Mean squared prediction error
AI 理解论文
溯源树
样例
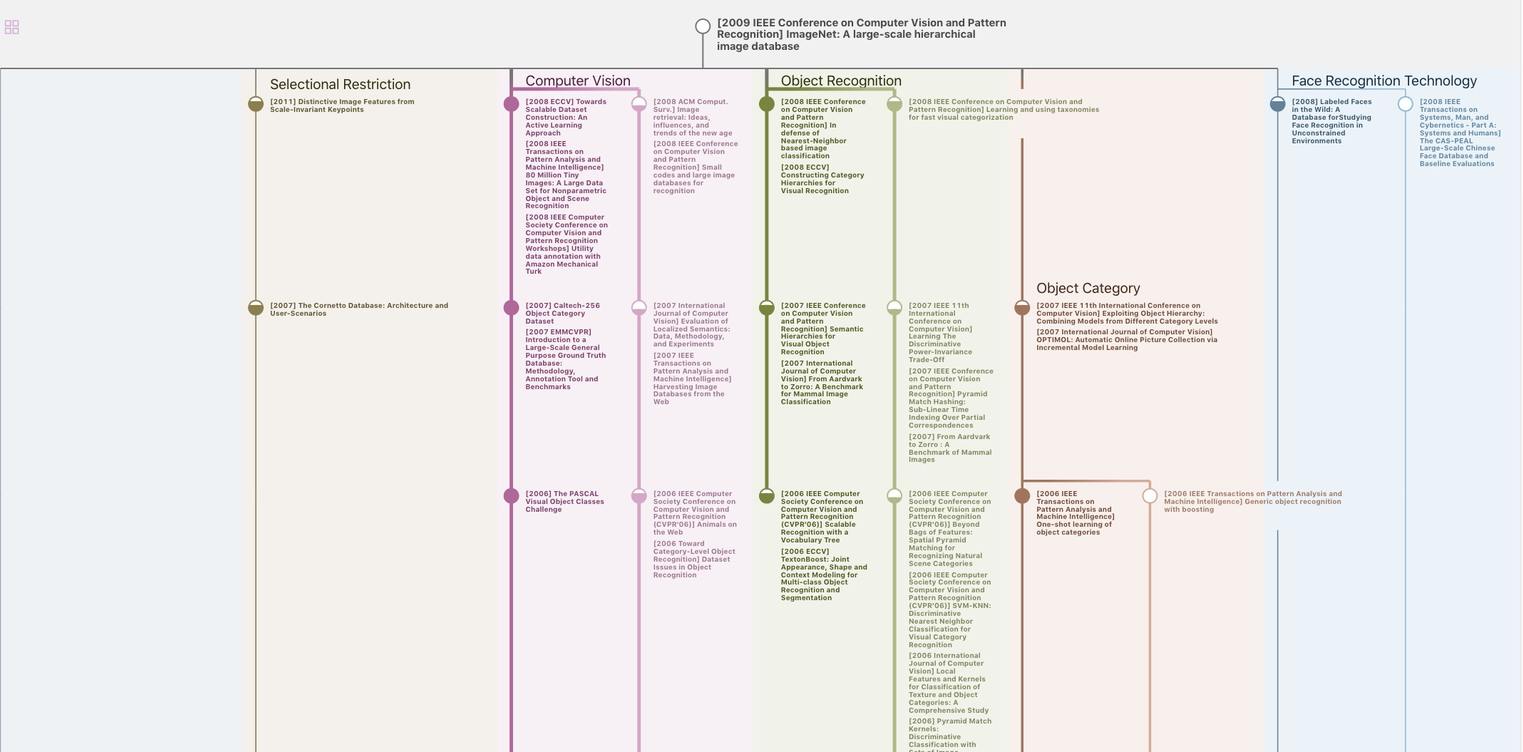
生成溯源树,研究论文发展脉络
Chat Paper
正在生成论文摘要