Visual Landmark Selection for Generating Grounded and Interpretable Navigation Instructions
user-5d8054e8530c708f9920ccce(2019)
Abstract
Instruction following for vision-and-language navigation (VLN) has prompted significant research efforts developing more powerful “follower” models since its inception in [1]; however, the inverse task of generating visually grounded instructions given a trajectory – or learning a “speaker” model – has been largely unexamined. This task is itself a challenging visually-grounded language generation problem akin to video or image captioning. Unlike these tasks however, instruction generation has a straightforward notion of correctness – can a follower arrive at the correct location based on generated instructions? Further, improved speaker models can be leveraged to strengthen follower models via data augmentation or back-translation. In this abstract we present a work-in-progress “speaker” model that generates navigation instructions in two stages, by first selecting a series of discrete visual landmarks along a trajectory using hard attention, and then second generating language instructions conditioned on these landmarks. This two-stage approach improves over prior work, while also permitting greater interpretability. We hope to extend this to a reinforcement learning setting where landmark selection is optimized to maximize a follower’s performance without disrupting the model’s language fluency.
MoreTranslated text
AI Read Science
Must-Reading Tree
Example
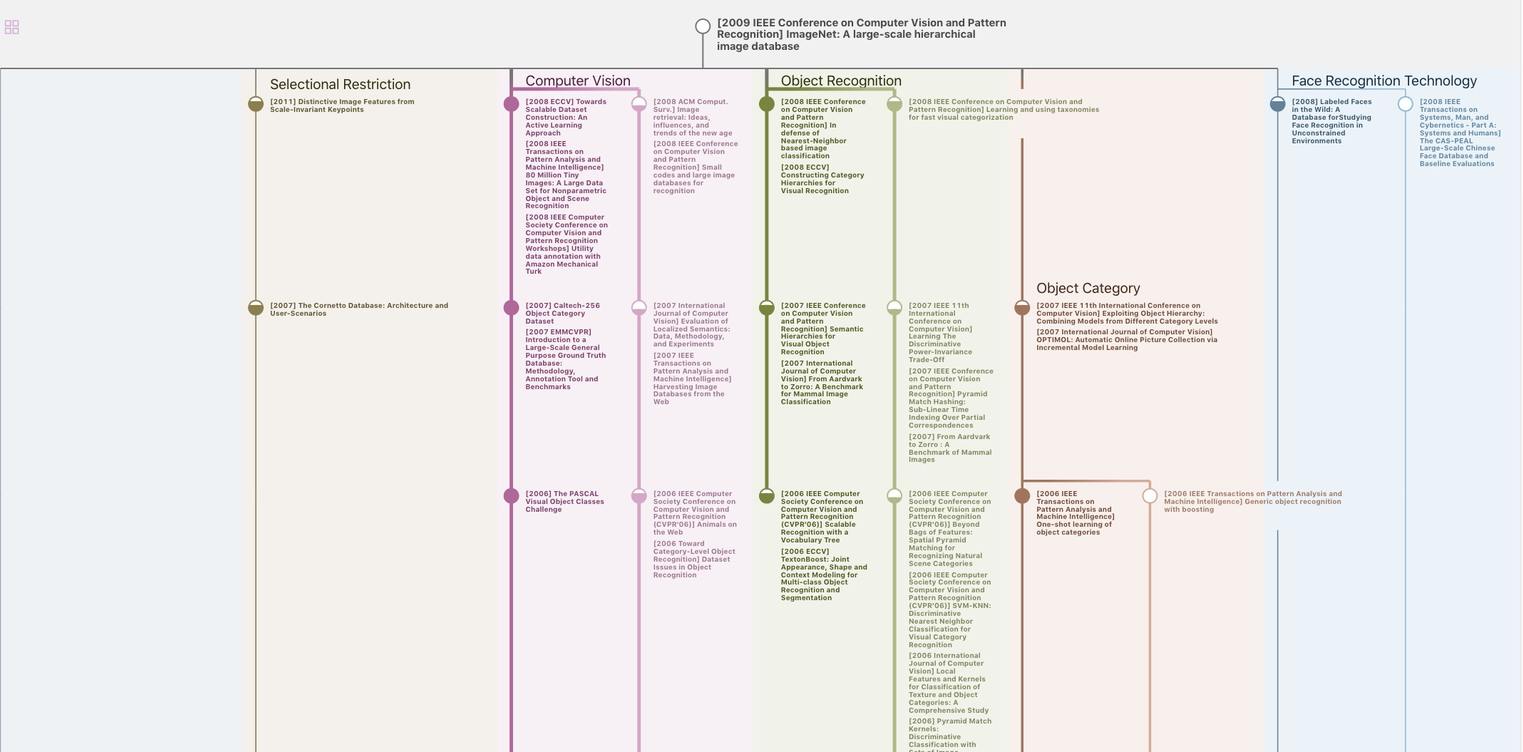
Generate MRT to find the research sequence of this paper
Chat Paper
Summary is being generated by the instructions you defined