BIAS REDUCTION IN SAMPLE-BASED OPTIMIZATION
SIAM JOURNAL ON OPTIMIZATION(2022)
摘要
We consider the stochastic optimization problems which use observed data to estimate essential characteristics of the random quantities involved. Sample average approximation (SAA) or empirical (plug-in) estimation are very popular ways to use data in optimization. It is well known that SAA suffers from downward bias. Our proposal is to use smooth estimators rather than empirical ones in the optimization problems. We establish consistency results for the optimal value and the set of optimal solutions of the new problem formulation. The performance of the proposed approach is compared to SAA theoretically and numerically. We analyze the bias of the new problems and identify sufficient conditions for ensuring less biased estimation of the optimal value of the true problem. At the same time, the error of the new estimator remains controlled. Those conditions are satisfied for many popular statistical problems such as regression models, classification problems, and optimization problems with average (conditional) value at risk. We have proved that smoothing the least-squares objective in a regression problem by a normal kernel leads to a ridge regression. Our numerical experience shows that the new estimators also frequently exhibit smaller variance and smaller mean-square error than those of SAA.
更多查看译文
关键词
kernel estimators, stochastic programming, sample average approximation, strong law of large numbers, smoothing, regularized regression
AI 理解论文
溯源树
样例
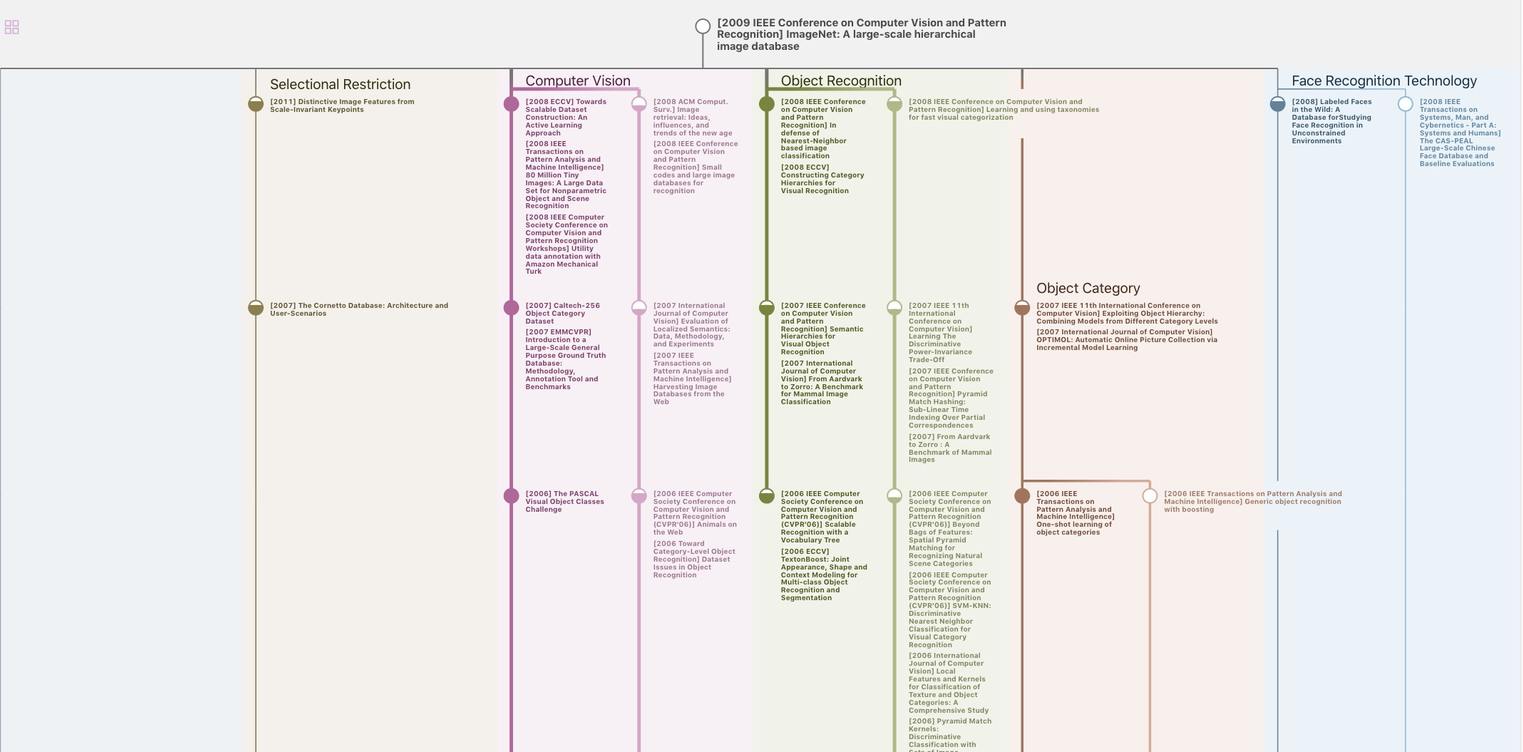
生成溯源树,研究论文发展脉络
Chat Paper
正在生成论文摘要