Machine Learning Models For Predicting Critical Illness Risk In Hospitalized Patients With Covid-19 Pneumonia
JOURNAL OF THORACIC DISEASE(2021)
摘要
Background: To develop machine learning classifiers at admission for predicting which patients with coronavirus disease 2019 (COVID-19) who will progress to critical illness.Methods: A total of 158 patients with laboratory-confirmed COVID-19 admitted to three designated hospitals between December 31, 2019 and March 31, 2020 were retrospectively collected. 27 clinical and laboratory variables of COVID-19 patients were collected from the medical records. A total of 201 quantitative CT features of COVID-19 pneumonia were extracted by using an artificial intelligence software. The critically ill cases were defined according to the COVID-19 guidelines. The least absolute shrinkage and selection operator (LASSO) logistic regression was used to select the predictors of critical illness from clinical and radiological features, respectively. Accordingly, we developed clinical and radiological models using the following machine learning classifiers, including naive bayes (NB), linear regression (LR), random forest (RF), extreme gradient boosting (XGBoost), adaptive boosting (AdaBoost), K-nearest neighbor (KNN), kernel support vector machine (k-SVM), and back propagation neural networks (BPNN). The combined model incorporating the selected clinical and radiological factors was also developed using the eight above-mentioned classifiers. The predictive efficiency of the models is validated using a 5-fold cross validation method. The performance of the models was compared by the area under the receiver operating characteristic curve (AUC).Results: The mean age of all patients was 58.9 +/- 13.9 years and 89 (56.3%) were males. 35 (22.2%) patients deteriorated to critical illness. After LASSO analysis, four clinical features including lymphocyte percentage, lactic dehydrogenase, neutrophil count, and D-dimer and four quantitative CT features were selected. The XGBoost-based clinical model yielded the highest AUC of 0.960 [95% confidence interval (CI): 0.913-1.000)]. The XGBoost-based radiological model achieved an AUC of 0.890 (95% CI: 0.757-1.000). However, the predictive efficacy of XGBoost-based combined model was very close to that of the XGBoost based clinical model, with an AUC of 0.955 (95% CI: 0.906-1.000).Conclusions: A XGBoost-based based clinical model on admission might be used as an effective tool to identify patients at high risk of critical illness.
更多查看译文
关键词
COVID-19, critical illness, chest CT, machine learning, prediction
AI 理解论文
溯源树
样例
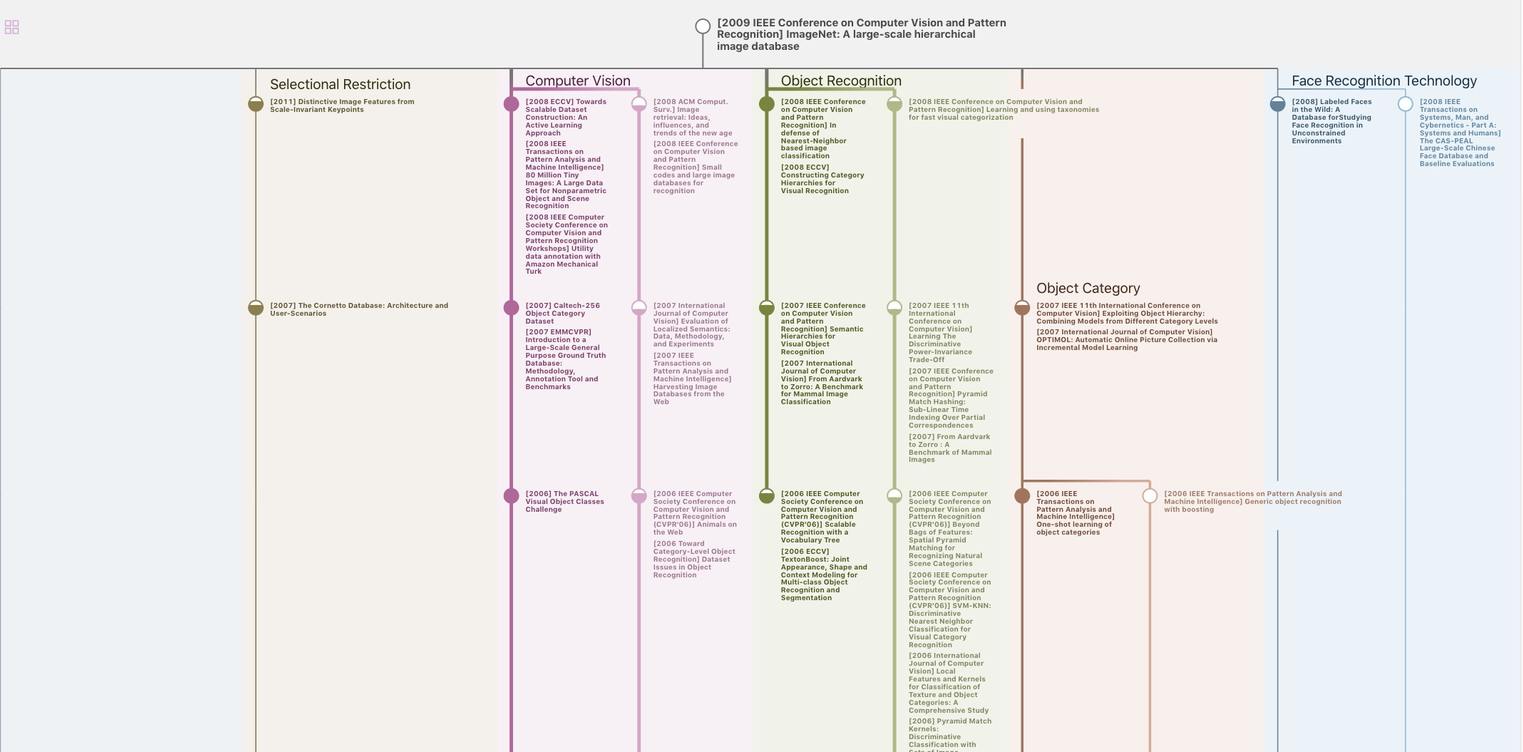
生成溯源树,研究论文发展脉络
Chat Paper
正在生成论文摘要