Multi-Modal Imitation Learning in Partially Observable Environments
user-5fe1a78c4c775e6ec07359f9(2020)
摘要
We consider imitation learning for agents to learn good policies from expert demonstration without any reward signal. Typical methods focus on single-expert single-task imitation in a fully observable environment. In practice, however, agents mostly make decisions based on their local observations, and the ability to generalize across various experts’ behaviors and multiple tasks is crucial for practical imitation learning. In this paper, we propose to take advantage of InfoGAIL with RNN-based belief state representations for multi-modal imitation learning in partially observable environments. We confirm the effectiveness of multi-expert learning of our method in a 2-dimensional environment, in which expert trajectories consist of two human-distinguishable behaviors. Further experimental results in continuous-control locomotion tasks reveal that our method can also disentangle interpretable latent factors in unlabeled multi-task demonstrations.
更多查看译文
AI 理解论文
溯源树
样例
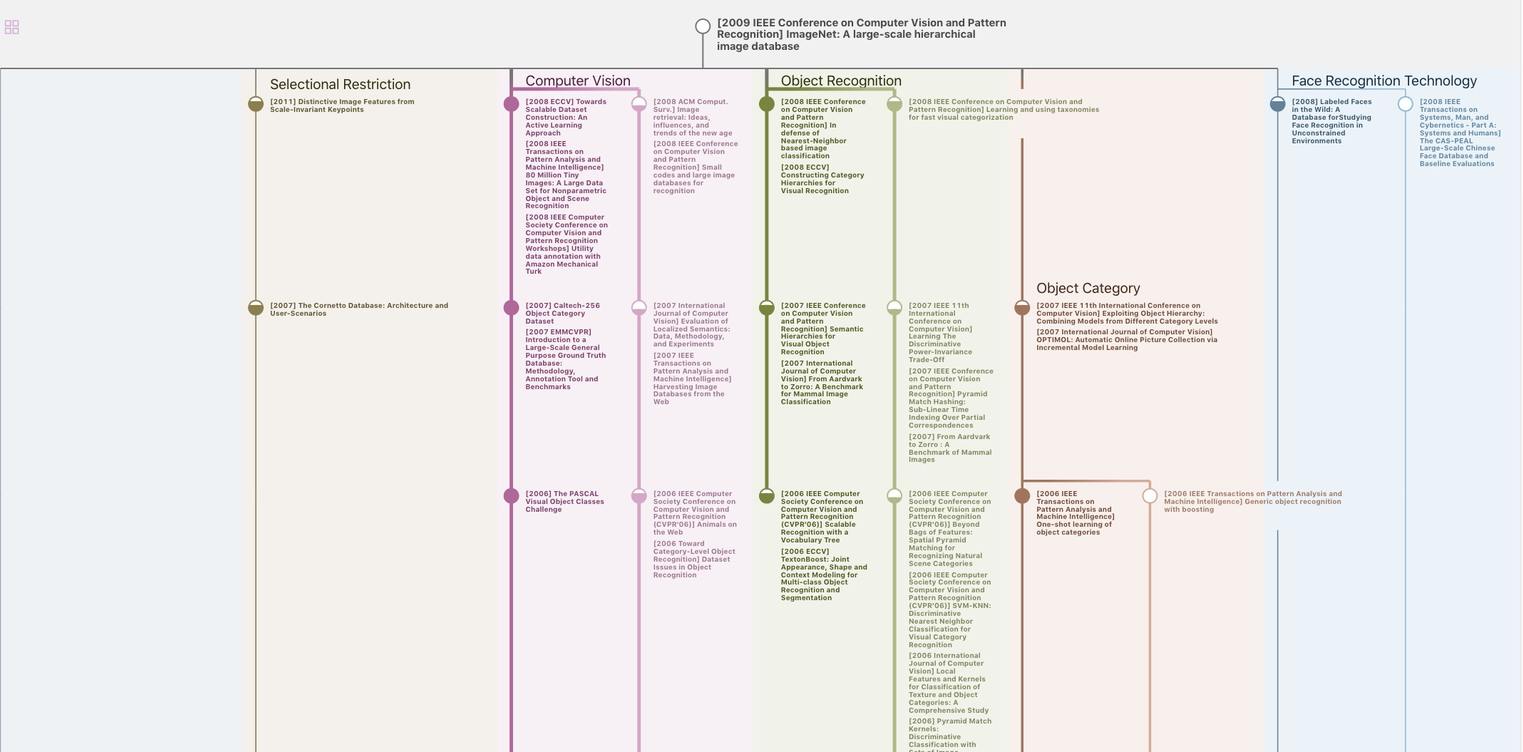
生成溯源树,研究论文发展脉络
Chat Paper
正在生成论文摘要