Learning Robust Models by Countering Spurious Correlations
user-5edf3a5a4c775e09d87cc848(2021)
摘要
Machine learning has demonstrated remarkable prediction accuracy over i.i.d data, but the accuracy often drops when tested with data from another distribution. One reason behind this accuracy drop is the reliance of models on the features that are only associated with the label in the training distribution, but not the test distribution. This problem is usually known as spurious correlation, confounding factors, or dataset bias. In this paper, we formally study the generalization error bound for this setup with the knowledge of how the spurious features are associated with the label. We also compare our analysis to the widely-accepted domain adaptation error bound and show that our bound can be tighter, with more assumptions that we consider realistic. Further, our analysis naturally offers a set of solutions for this problem, linked to established solutions in various topics about robustness in general, and these solutions all require some understandings of how the spurious features are associated with the label. Finally, we also briefly discuss a method that does not require such an understanding.
更多查看译文
关键词
Spurious relationship,Spurious correlation,Robustness (computer science),Algorithm,Confounding,Computer science,Domain adaptation,Generalization error
AI 理解论文
溯源树
样例
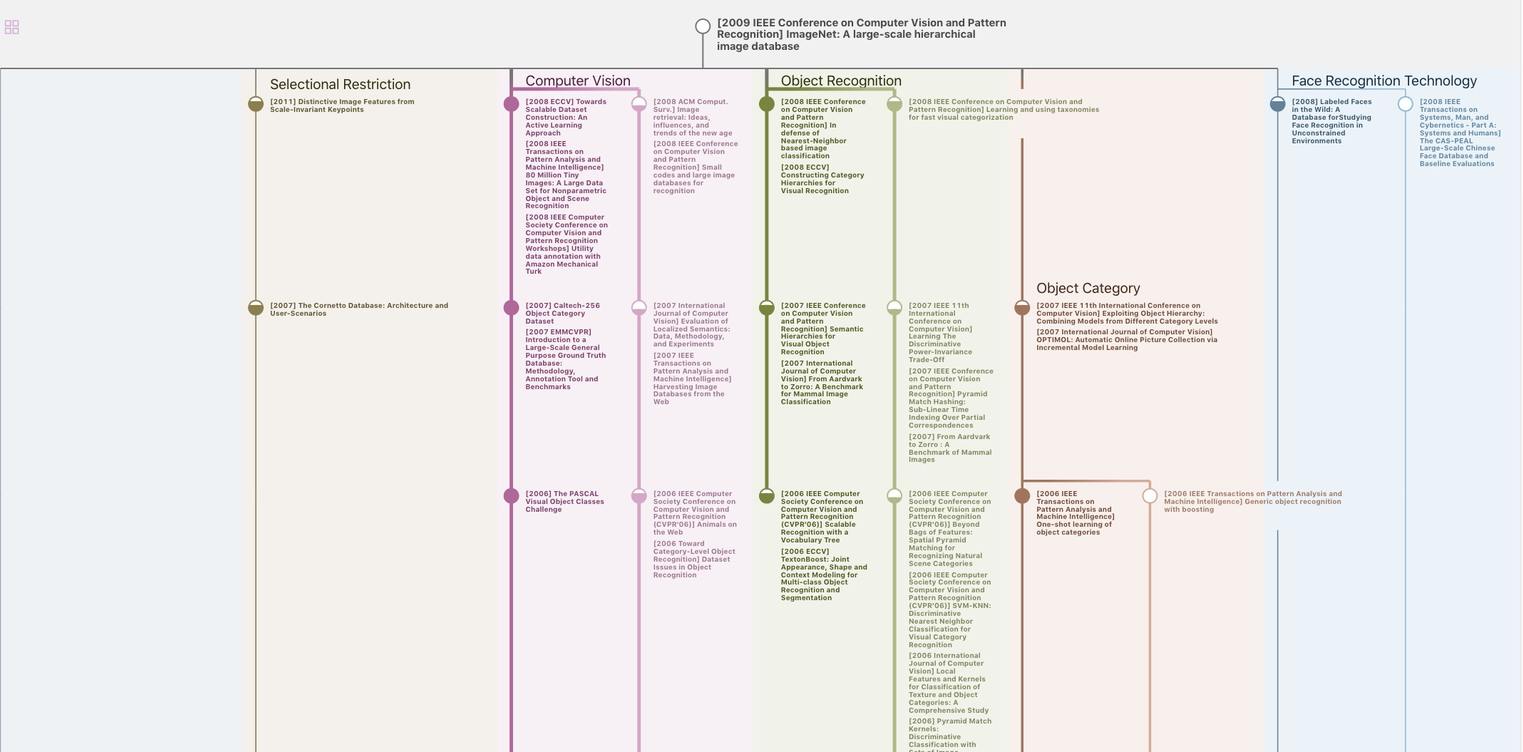
生成溯源树,研究论文发展脉络
Chat Paper
正在生成论文摘要