On the Stability of Multi-branch Network
user-5d4bc4a8530c70a9b361c870(2021)
摘要
Multi-branch architectures are widely used in state-of-the-art neural networks. Their empirical success relies on some design wisdom, like adding normalization layers or/and scaling down the initialization. In this paper, we investigate the multi-branch architecture from the stability perspective. Specifically, we establish the forward/backward stability of multi-branch network, which leads to several new findings. Our analysis shows that only scaling down the initialization may not be enough for training multi-branch network successfully because of the uncontrollable backward process. We also unveil a new role of the normalization layer in terms of stabilizing the multi-branch architectures. More importantly, we propose a new design ``STAM aggregation" that can guarantee to STAbilize the forward/backward process of Multi-branch networks irrespective of the number of branches. We demonstrate that with STAM aggregation, the same training strategy is applicable to models with different numbers of branches, which can reduce the hyper-parameter tuning burden. Our experiments verify our theoretical findings and also demonstrate that the STAM aggregation can improve the performance of multi-branch networks considerably.
更多查看译文
关键词
Initialization,Normalization (statistics),Artificial neural network,Algorithm,Scaling,Computer science,Backward propagation
AI 理解论文
溯源树
样例
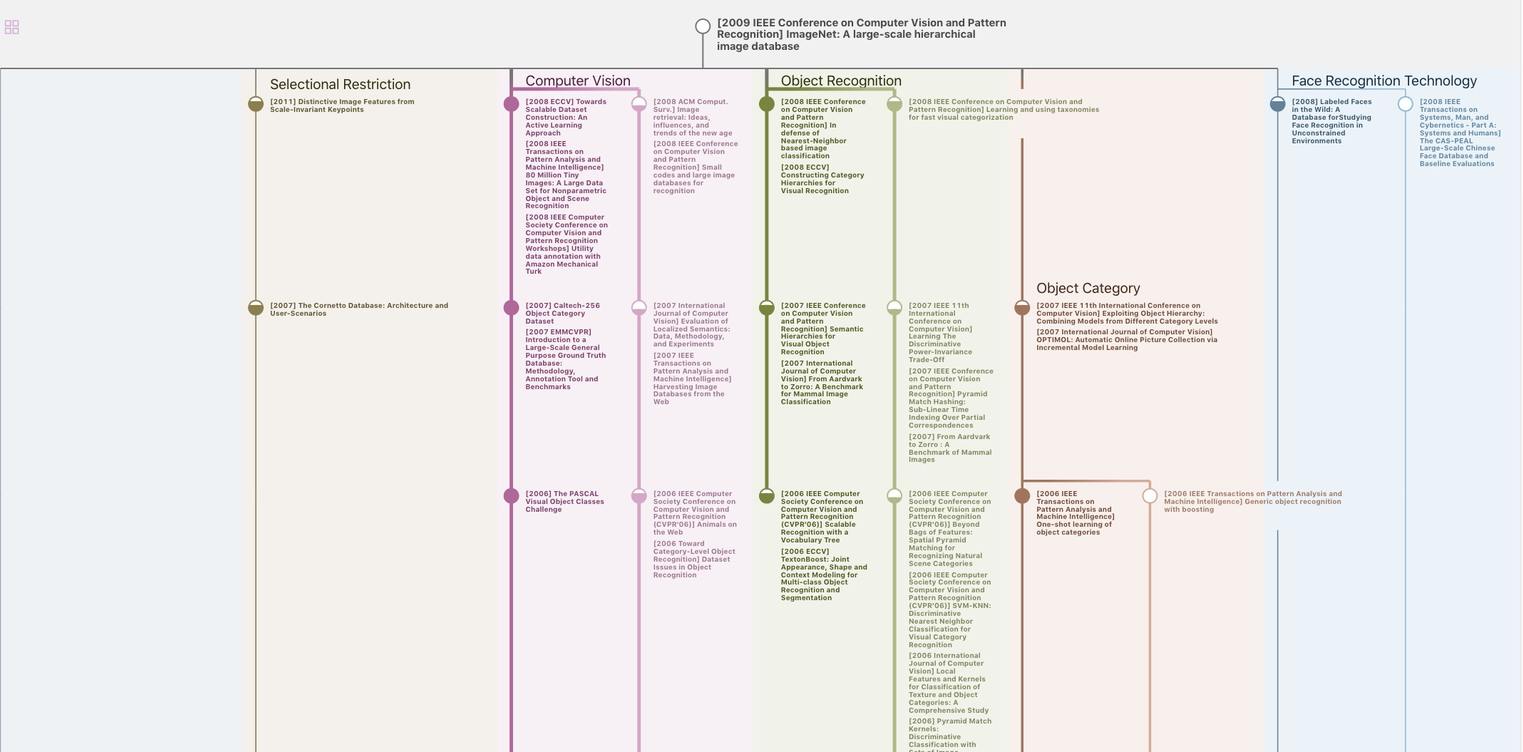
生成溯源树,研究论文发展脉络
Chat Paper
正在生成论文摘要