Unconventional computing using evolution-in-nanomaterio: neural networks meet nanoparticle networks
user-5d8054e8530c708f9920ccce(2016)
摘要
Recently published experimental work on evolution-in-materio applied to nanoscale materials shows promising results for future reconfigurable devices. These experiments were performed on disordered nano-particle networks that have no predefined design. The material has been treated as a blackbox, and genetic algorithms have been used to find appropriate configuration voltages to enable the target functionality. In order to support future experiments, we developed simulation tools for predicting candidate functionalities. One of these tools is based on a physical model, but the one we introduce in this paper is based on an artificial neural network. The advantage of this newly presented approach is that, after training the neural network to match either the real material or its physical model, it can be configured using gradient descent instead of a black-box optimisation. The experiments we report here demonstrate that the neural network can model the simulated nano-material quite accurately. The differentiable, neural network-based material model is then used to find logic gates, as a proof of principle. This shows that the new approach has great potential for partly replacing costly and time-consuming experiments with the real materials. Therefore, this approach has a high relevance for future computing, either as an alternative to digital computing or as an alternative way of producing multi-functional reconfigurable devices.
更多查看译文
关键词
Unconventional computing,Artificial neural network,Gradient descent,Proof of concept,Genetic algorithm,Logic gate,Computer engineering,Artificial intelligence,Differentiable function,Voltage,Engineering
AI 理解论文
溯源树
样例
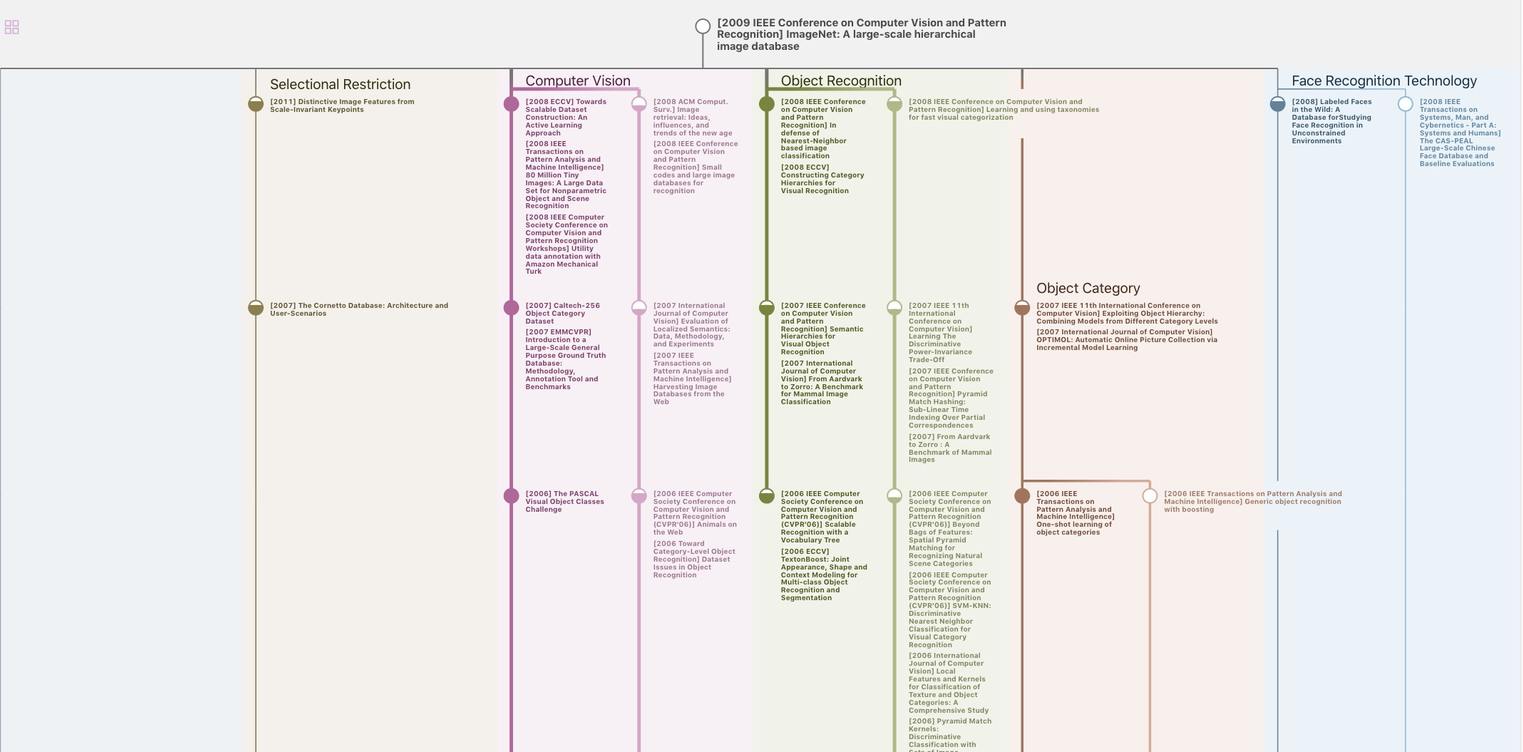
生成溯源树,研究论文发展脉络
Chat Paper
正在生成论文摘要