Anti-Adversarially Manipulated Attributions for Weakly and Semi-Supervised Semantic Segmentation
CVPR(2021)
摘要
Weakly supervised semantic segmentation produces a pixel-level localization from class labels; but a classifier trained on such labels is likely to restrict its focus to a small discriminative region of the target object. AdvCAM is an attribution map of an image that is manipulated to increase the classification score produced by a classifier. This manipulation is realized in an anti-adversarial manner, which perturbs the original images along pixel gradients in the opposite direction from those used in an adversarial attack. It forces regions initially considered not to be discriminative to become involved in subsequent classifications, and produces attribution maps that successively identify more regions of the target object. In addition, we introduce a new regularization procedure that inhibits both the incorrect attribution of regions unrelated to the target object and excessive concentration of attributions on a small region of that object. Our method is a post-hoc analysis of a trained classifier, which does not need to be altered or retrained. On PASCAL VOC 2012 test images, we achieve mIoUs of 68.0 and 76.9 for weakly and semi-supervised semantic segmentation respectively, which represent a new state-of-the-art.
更多查看译文
关键词
attributions,weakly,anti-adversarially,semi-supervised
AI 理解论文
溯源树
样例
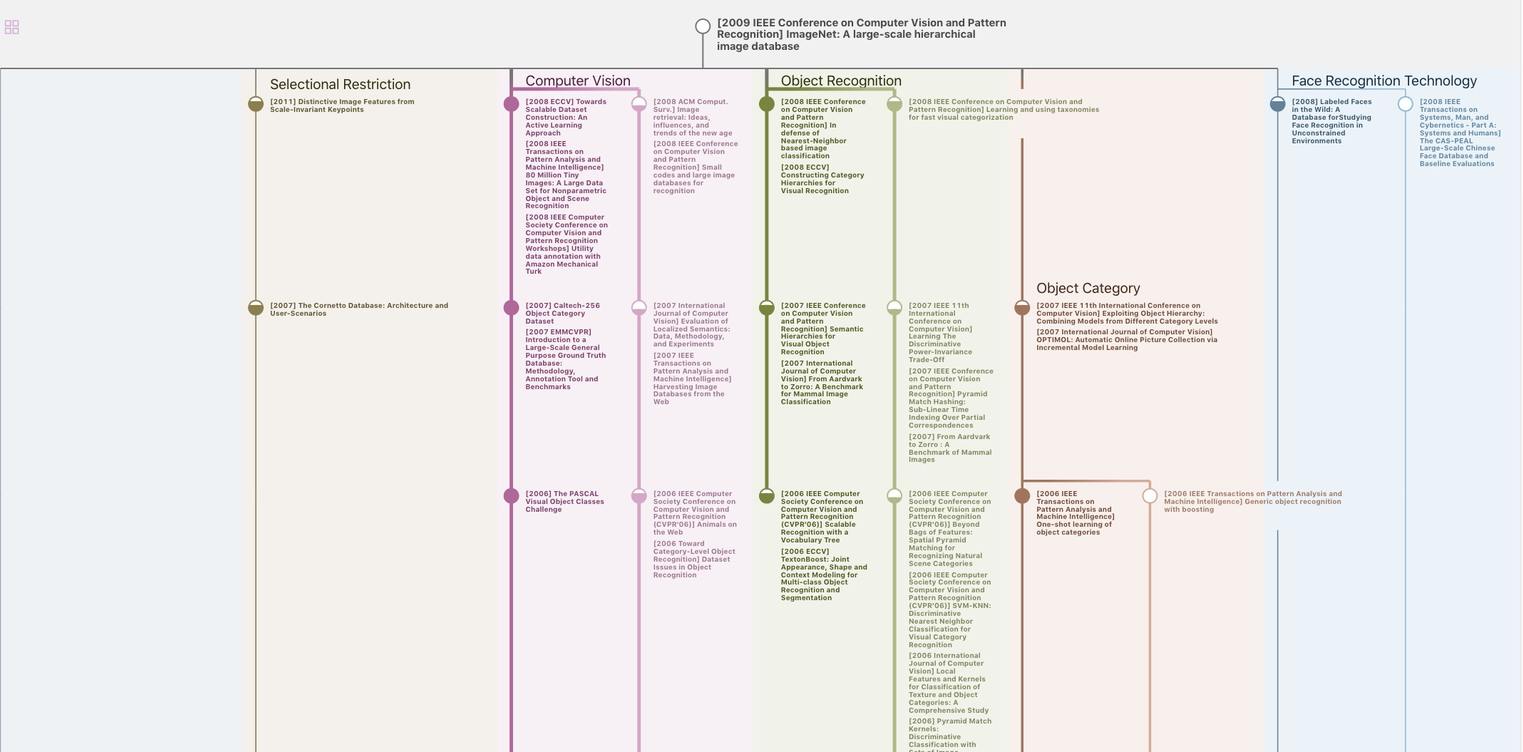
生成溯源树,研究论文发展脉络
Chat Paper
正在生成论文摘要