Who Needs MLOps: What Data Scientists Seek to Accomplish and How Can MLOps Help?
2021 IEEE/ACM 1st Workshop on AI Engineering - Software Engineering for AI (WAIN)(2021)
摘要
Following continuous software engineering practices, there has been an increasing interest in rapid deployment of machine learning (ML) features, called MLOps. In this paper, we study the importance of MLOps in the context of data scientists' daily activities, based on a survey where we collected responses from 331 professionals from 63 different countries in ML domain, indicating on what they were working on in the last three months. Based on the results, up to 40% respondents say that they work with both models and infrastructure; the majority of the work revolves around relational and time series data; and the largest categories of problems to be solved are predictive analysis, time series data, and computer vision. The biggest perceived problems revolve around data, although there is some awareness of problems related to deploying models to production and related procedures. To hypothesise, we believe that organisations represented in the survey can be divided to three categories - (i) figuring out how to best use data; (ii) focusing on building the first models and getting them to production; and (iii) managing several models, their versions and training datasets, as well as retraining and frequent deployment of retrained models. In the results, the majority of respondents are in category (i) or (ii), focusing on data and models; however the benefits of MLOps only emerge in category (iii) when there is a need for frequent retraining and redeployment. Hence, setting up an MLOps pipeline is a natural step to take, when an organization takes the step from ML as a proof-of-concept to ML as a part of nominal activities.
更多查看译文
关键词
Artificial intelligence,AI,machine learning,ML,continuous software engineering,delivery pipeline,DevOps,MLOps
AI 理解论文
溯源树
样例
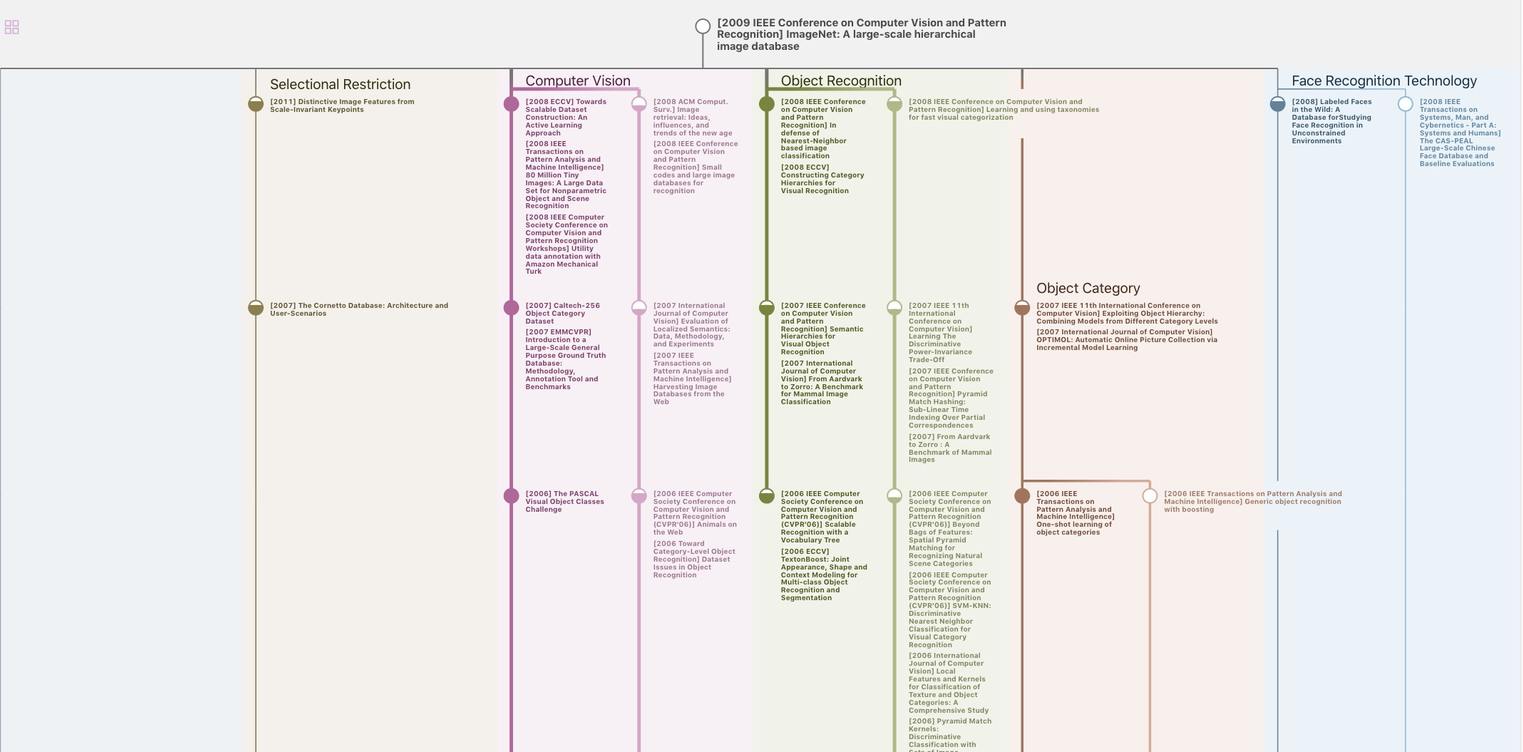
生成溯源树,研究论文发展脉络
Chat Paper
正在生成论文摘要