A Novel Adaptive Greedy Strategy Based On Gaussian Mixture Clustering For Multiobjective Optimization
SWARM AND EVOLUTIONARY COMPUTATION(2021)
摘要
The tradeoff between exploitation and exploration is a dilemma inherent to evolutionary multiobjective optimiza-tion. In this paper, we propose a novel adaptive greedy strategy (AGS) to strike a balance between exploiting and exploring during the evolutionary search. Specifically, it consists of two interdependent parts, i.e., a clustering-based reproduction operator and a utility dependent exploitation probability. At each evolutionary cycle, in accordance with the manifold structure of the Pareto optimal set, the reproduction operator employs Gaussian mixture clustering to establish the mating pool for each solution, where the parent candidates of each solution are paired to generate offsprings from the same cluster for exploitation or from its extended set for exploration in terms of the exploitation probability. Thereafter, the exploitation probability is updated adaptively leaned on the utility of reproduction operator, it is evaluated from proximity or diversity at different evolutionary stage. Moreover, a multiobjective evolutionary algorithm with AGS, named MEAGS, is proposed and compared with four state-of-the-art algorithms on several benchmarks. The experimental results show that there is a significant improvement over the representative algorithms.
更多查看译文
关键词
Evolutionary algorithm, Estimation of distribution, Multiobjective optimization, Greedy strategy, Gaussian mixture model
AI 理解论文
溯源树
样例
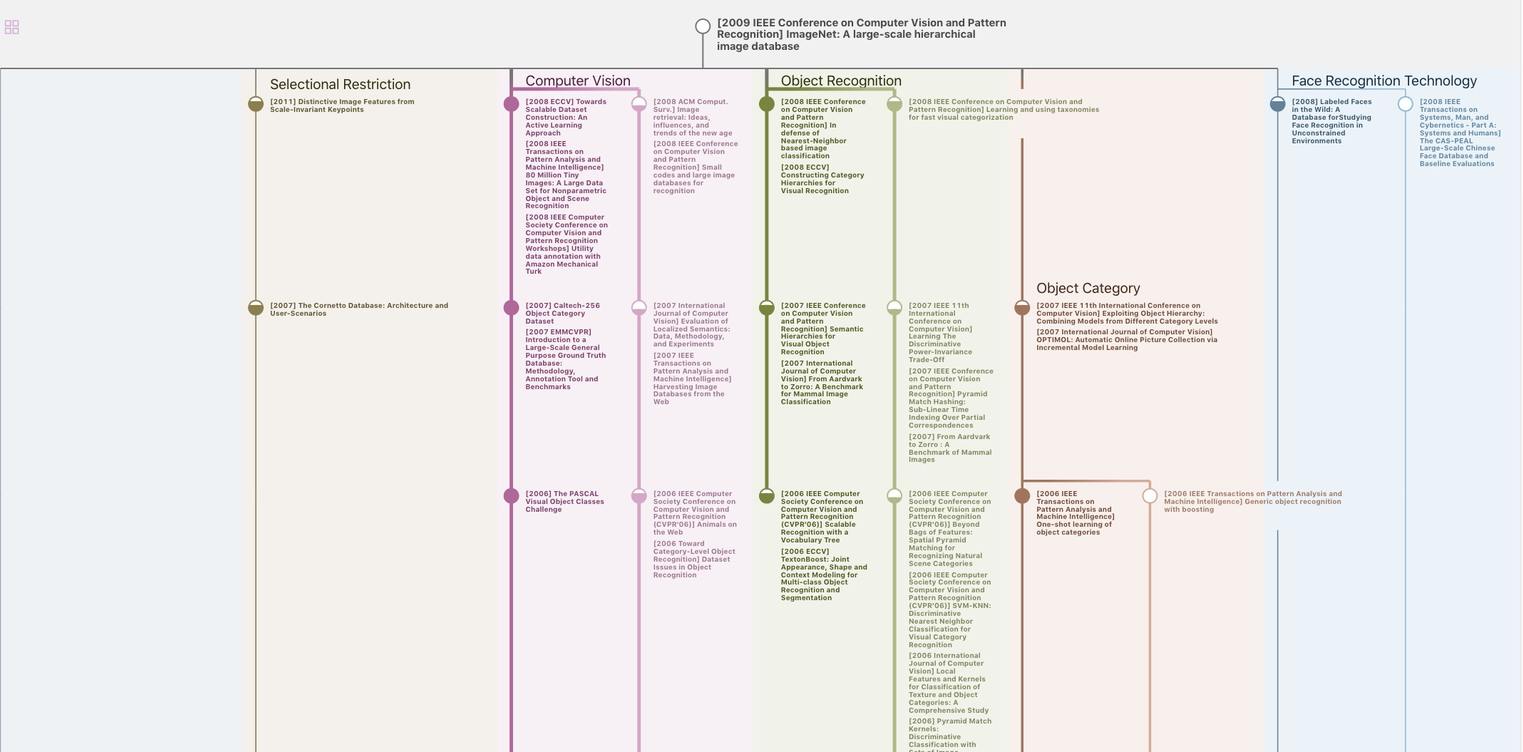
生成溯源树,研究论文发展脉络
Chat Paper
正在生成论文摘要