Decoding With Confidence: Statistical Control On Decoder Maps
NEUROIMAGE(2021)
摘要
In brain imaging, decoding is widely used to infer relationships between brain and cognition, or to craft brain imaging biomarkers of pathologies. Yet, standard decoding procedures do not come with statistical guarantees, and thus do not give confidence bounds to interpret the pattern maps that they produce. Indeed, in whole-brain decoding settings, the number of explanatory variables is much greater than the number of samples, hence classical statistical inference methodology cannot be applied. Specifically, the standard practice that consists in thresholding decoding maps is not a correct inference procedure. We contribute a new statistical-testing framework for this type of inference. To overcome the statistical inefficiency of voxel-level control, we generalize the Family Wise Error Rate (FWER) to account for a spatial tolerance 6, introducing the 6-Family Wise Error Rate ( 6-FWER). Then, we present a decoding procedure that can control the 6-FWER: the Ensemble of Clustered Desparsified Lasso (EnCluDL), a procedure for multivariate statistical inference on high-dimensional structured data. We evaluate the statistical properties of EnCluDL with a thorough empirical study, along with three alternative procedures including decoder map thresholding. We show that EnCluDL exhibits the best recovery properties while ensuring the expected statistical control.
更多查看译文
关键词
fMRI, Decoding, Statistical methods, Multivariate model, Inference, Statistical control, Support recovery, High dimension
AI 理解论文
溯源树
样例
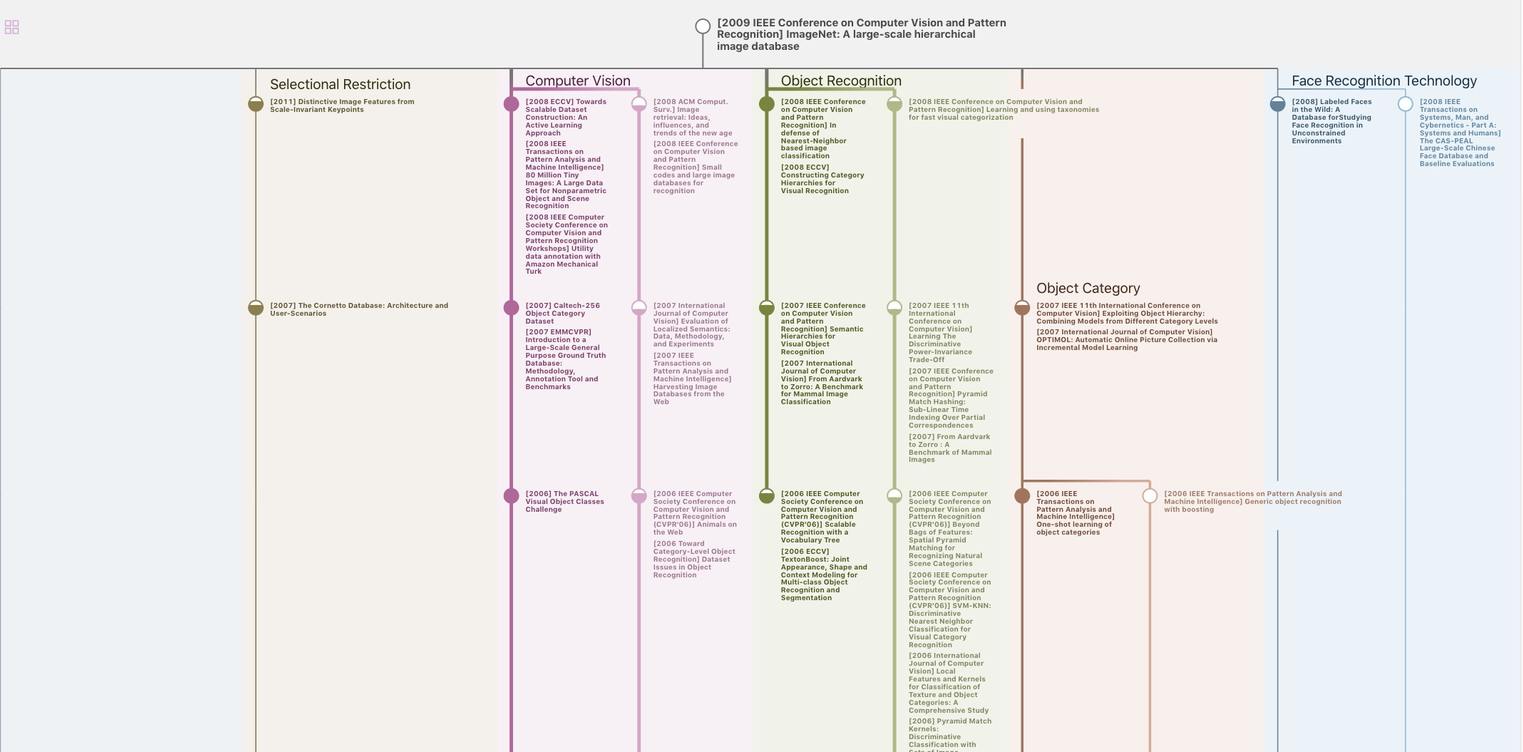
生成溯源树,研究论文发展脉络
Chat Paper
正在生成论文摘要