Disentangled Cycle Consistency for Highly-realistic Virtual Try-On
2021 IEEE/CVF CONFERENCE ON COMPUTER VISION AND PATTERN RECOGNITION, CVPR 2021(2021)
摘要
Image virtual try-on replaces the clothes on a person image with a desired in-shop clothes image. It is challenging because the person and the in-shop clothes are unpaired. Existing methods formulate virtual try-on as either in-painting or cycle consistency. Both of these two formulations encourage the generation networks to reconstruct the input image in a self-supervised manner. However, existing methods do not differentiate clothing and non-clothing regions. A straightforward generation impedes the virtual try-on quality because of the heavily coupled image contents. In this paper, we propose a Disentangled Cycle-consistency Try-On Network (DCTON). The DCTON is able to produce highly-realistic try-on images by disentangling important components of virtual try-on including clothes warping, skin synthesis, and image composition. Moreover, DCTON can be naturally trained in a self-supervised manner following cycle consistency learning. Extensive experiments on challenging benchmarks show that DCTON outperforms state-of-the-art approaches favorably.
更多查看译文
关键词
cycle consistency,highly-realistic
AI 理解论文
溯源树
样例
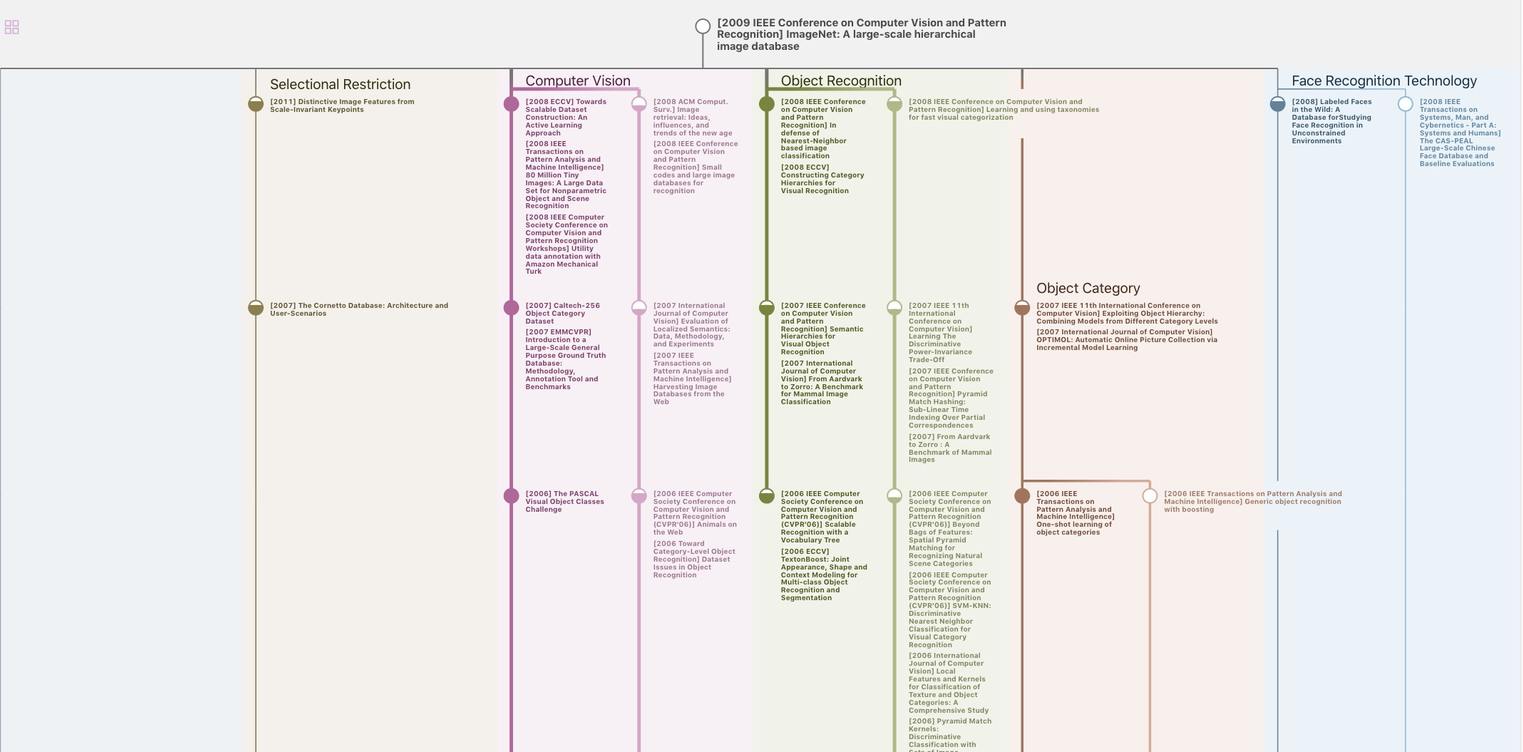
生成溯源树,研究论文发展脉络
Chat Paper
正在生成论文摘要